Discriminative elastic-net broad learning systems for visual classification
Applied Soft Computing(2024)
摘要
The broad learning system (BLS) has garnered significant attention in the realm of visual classification due to its exceptional balance between accuracy and efficiency. However, the supervision mechanism in BLS typically relies on strict binary labels, limiting the approximation freedom and failing to represent the data distribution adequately. Furthermore, the inadequacy of the guidance mechanism for the output weights hinders the precise approximation of the target spaces by the input features. To tackle these issues, in this paper, we propose two discriminative elastic-net regularized BLS models with label enhancement, achieving the following significant objectives: Firstly, the proposed label enhancement technologies can substantially augment the margins between different classes while enhancing the diversity within label spaces. Secondly, the compactness and effectiveness of the output weights can be further improved via the guidance of elastic-net regularization of singular values. Thirdly, our proposed algorithms can be efficiently optimized with the augmented Lagrangian method, whose convergence and calculation complexity can be guaranteed well with solid theoretical analysis. Extensive experiments are intended on various popular databases to compare our proposed models with numerous other state-of-the-art recognition algorithms. The numerical results indicate that our proposed algorithms can achieve the best face recognition accuracy of 99.67%, and 97.54% for object recognition. Even in challenging recognition tasks, our methods can still yield an average improvement of 0.8 percentage points.
更多查看译文
关键词
Broad learning system,Label enhancement,Elastic-net regularization,Discriminative,Visual classification
AI 理解论文
溯源树
样例
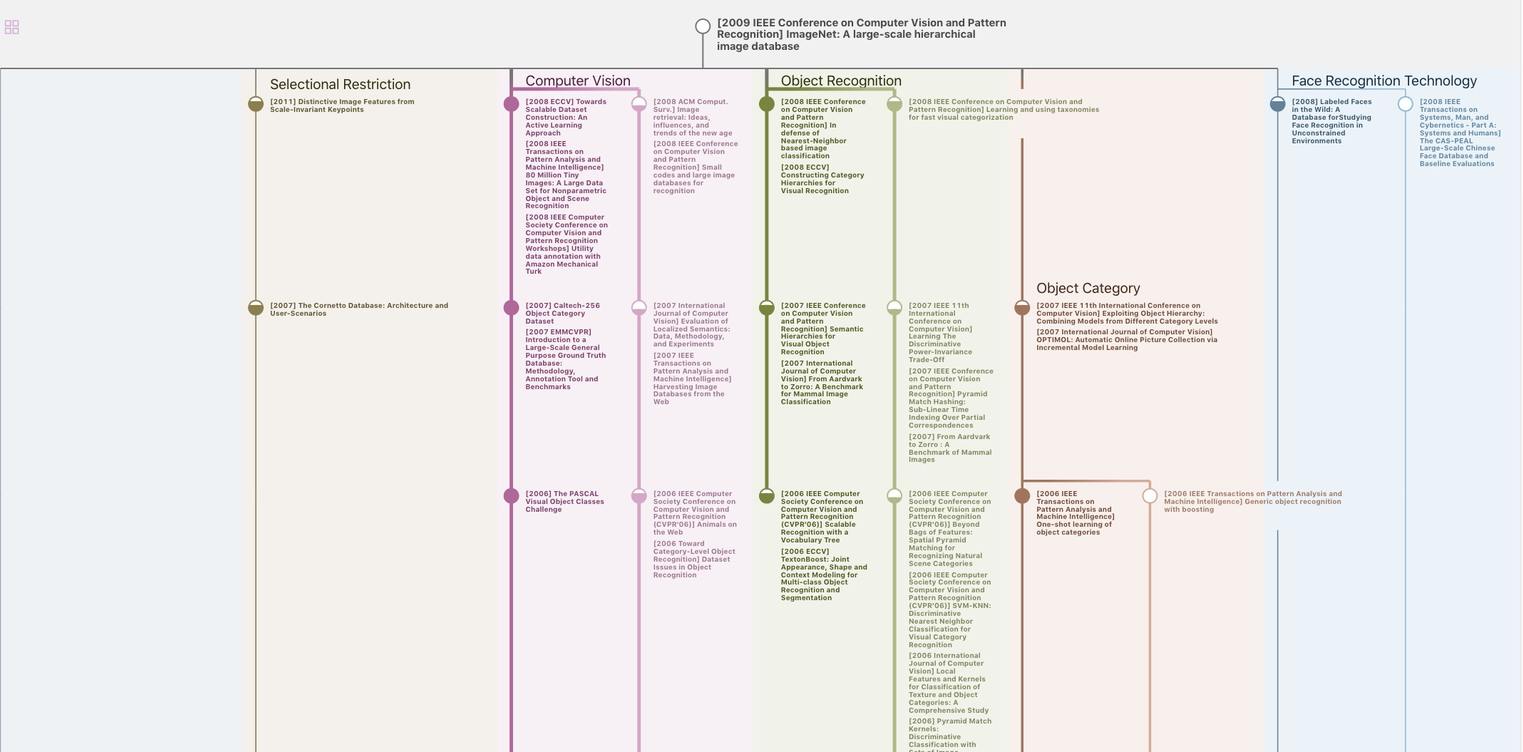
生成溯源树,研究论文发展脉络
Chat Paper
正在生成论文摘要