A deep learning-based model for tropospheric wet delay prediction based on multi-layer 1D convolution neural network
Advances in Space Research(2024)
摘要
Tropospheric delay constitutes a primary source of error in Global Navigation Satellite System (GNSS) navigation positioning. Existing machine learning zenith wet delay (ZWD) models have limitations in their feature extraction capabilities. To address these limitations, we propose a ZWD (CNN_ZWD) model which is built upon observation data collected from 88 radiosonde (RS) stations in China from 2015 to 2017, employing the one-dimensional convolutional neural network (1D-CNN) deep learning method. The accuracy of the CNN_ZWD model is validated using the 2018 RS data and is compared with other models. The results reveal that the root mean square error (RMSE) of the 1D-CNN-based empirical model CNN_ZWD-A is 4.29 cm. This marks a 0.17 cm (3.81%) improvement over the machine learning empirical models based on the random forest (RF) and back propagation neural network (BPNN) and a 0.70 cm (14.03%) enhancement over the GPT3 empirical model. Moreover, when meteorological data is available at the station, the meteorological parameterized CNN_ZWD-B model has an RMSE of 2.69 cm. Its precision closely matches that of the RF_ZWD-D model and slightly exceeds the BP_ZWD-F model (RMSE: 2.85 cm). Remarkably, compared to the conventional Saastamoinen (Saa_ZWD) model, our proposed model demonstrates a 32.24% increase in accuracy. This underscores that incorporating surface meteorological parameters into the functional formulation can significantly enhance the accuracy of regional ZWD prediction in China. Furthermore, compared with the empirical model, the predictive accuracy of the meteorological parameterized ZWD model based on the 1D-CNN exhibits significant improvement, particularly in China's monsoon climate region.
更多查看译文
关键词
Zenith wet delay,Deep learning,1D convolution neural network,Random forest,Back propagation neural network
AI 理解论文
溯源树
样例
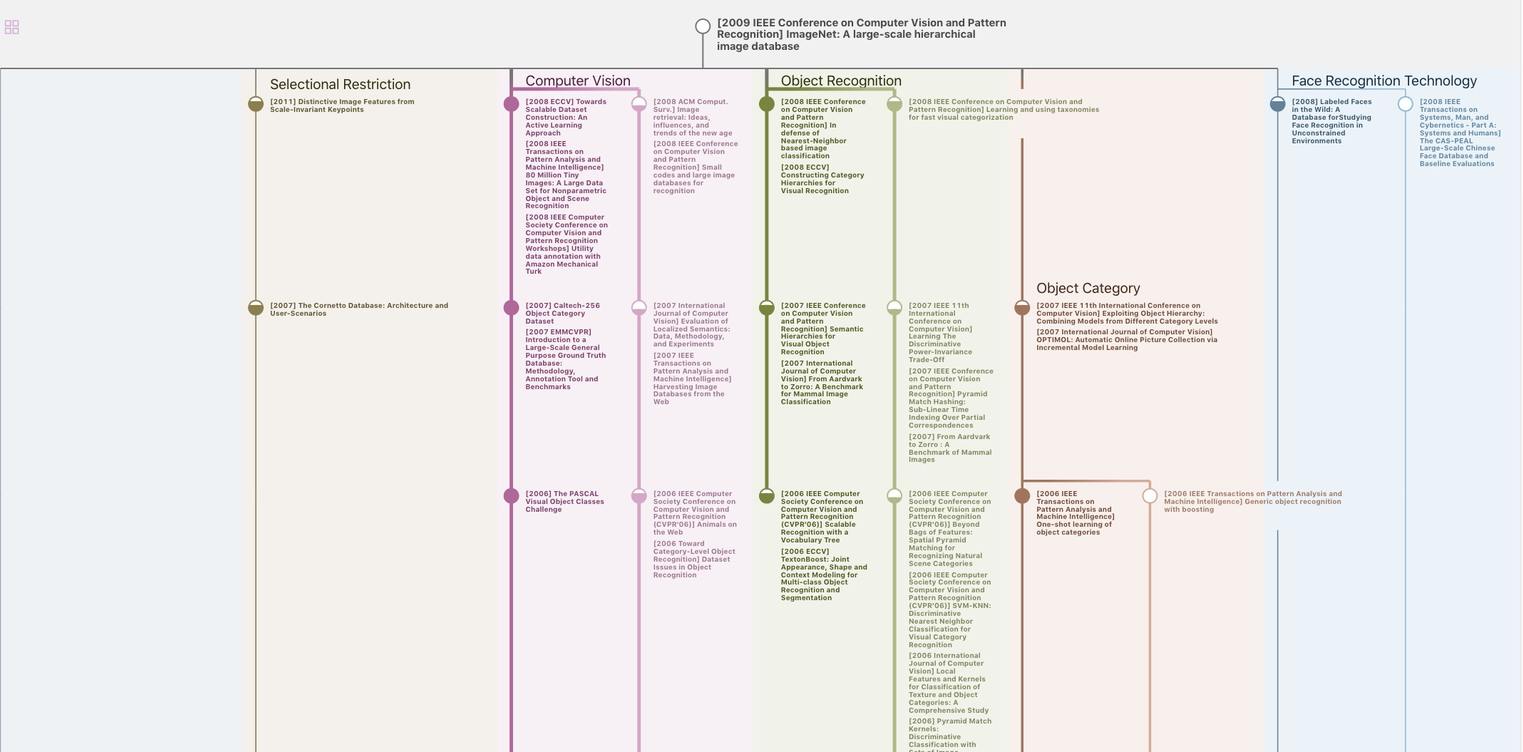
生成溯源树,研究论文发展脉络
Chat Paper
正在生成论文摘要