Enhancing Contrastive Learning with Positive Pair Mining for Few-shot Hyperspectral Image Classification
IEEE Journal of Selected Topics in Applied Earth Observations and Remote Sensing(2024)
摘要
In recent years, deep learning has emerged as the dominant approach for Hyperspectral Image (HSI) classification. However, deep neural networks require large annotated datasets to generalize well. This limits the applicability of deep learning for real-world HSI classification problems, as manual labeling of thousands of pixels per scene is costly and timeconsuming. In this paper, we tackle the problem of few-shot HSI classification by leveraging state-of-the-art self-supervised contrastive learning with an improved view-generation approach. Traditionally, contrastive learning algorithms heavily rely on hand-crafted data augmentations tailored for natural imagery to generate positive pairs. However, these augmentations are not directly applicable to hyperspectral images, limiting the potential of self-supervised learning in the hyperspectral domain. To overcome this limitation, we introduce two positive pair mining strategies for contrastive learning on hyperspectral images. The proposed strategies mitigate the need for high-quality data augmentations, providing an effective solution for few-shot HSI classification. Through extensive experiments, we show that the proposed approach improves accuracy and label efficiency on four popular HSI classification benchmarks. Furthermore, we conduct a thorough analysis of the impact of data augmentation in contrastive learning, highlighting the advantage of our positive pair mining approach.
更多查看译文
关键词
Hyperspectral Image Classification,SelfSupervised Learning,Contrastive Learning,Positive Pair Mining
AI 理解论文
溯源树
样例
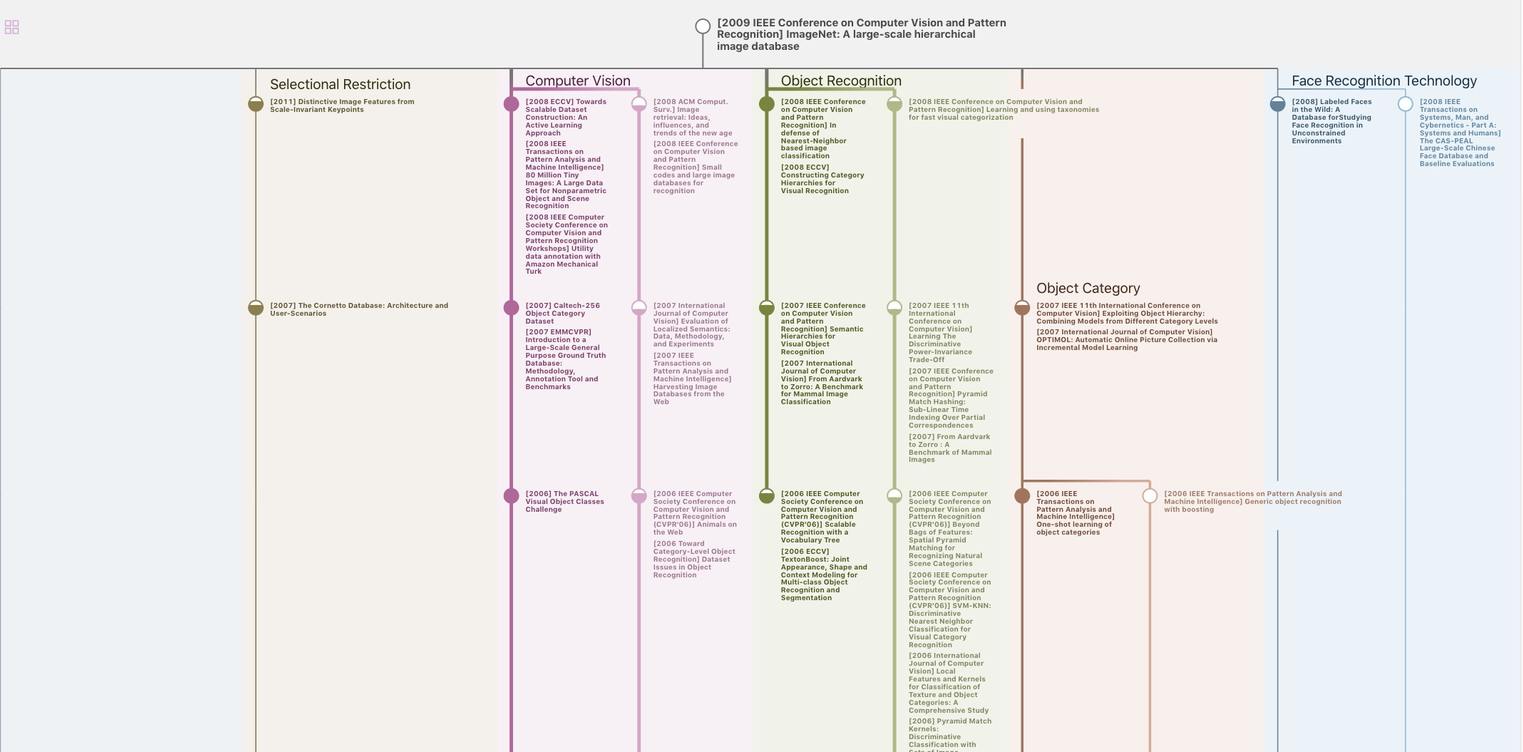
生成溯源树,研究论文发展脉络
Chat Paper
正在生成论文摘要