iPCa-Former: A Multi-Task Transformer Framework for Perceiving Incidental Prostate Cancer
IEEE SIGNAL PROCESSING LETTERS(2024)
摘要
Despite significant progress in medical image analysis using deep learning, predicting incidental prostate cancer (iPCa) remains challenging due to subtle differences in multiparametric magnetic resonance imaging (mpMRI) and a lower incidence rate. To address these challenges, we propose iPCa-Former, a transformer-based framework designed to enhance iPCa prediction within prostate mpMRI slices. Firstly, built on an encoder-decoder architecture, our iPCa-Former facilitates the simultaneous optimization of two tasks through mutual learning: prostate transition zone segmentation and iPCa prediction. Secondly, we introduce a joint optimization function that combines focal loss and boundary-based mutual information (BMI) loss, effectively addressing the imbalance of positive and negative samples in classification and the challenge posed by a small proportion of the foreground region in segmentation. Moreover, we construct an iPCa mpMRI dataset comprising 10,276 prostate mpMRI slices from 485 patients clinically diagnosed with benign prostatic hyperplasia; however, 27 out of these patients are identified as iPCa. When evaluated on this benchmark dataset, our iPCa-Former outperforms state-of-the-art methods, demonstrating the superior performance of our approach.
更多查看译文
关键词
Incidental prostate cancer,transformer,mutual learning,boundary-based mutual information loss
AI 理解论文
溯源树
样例
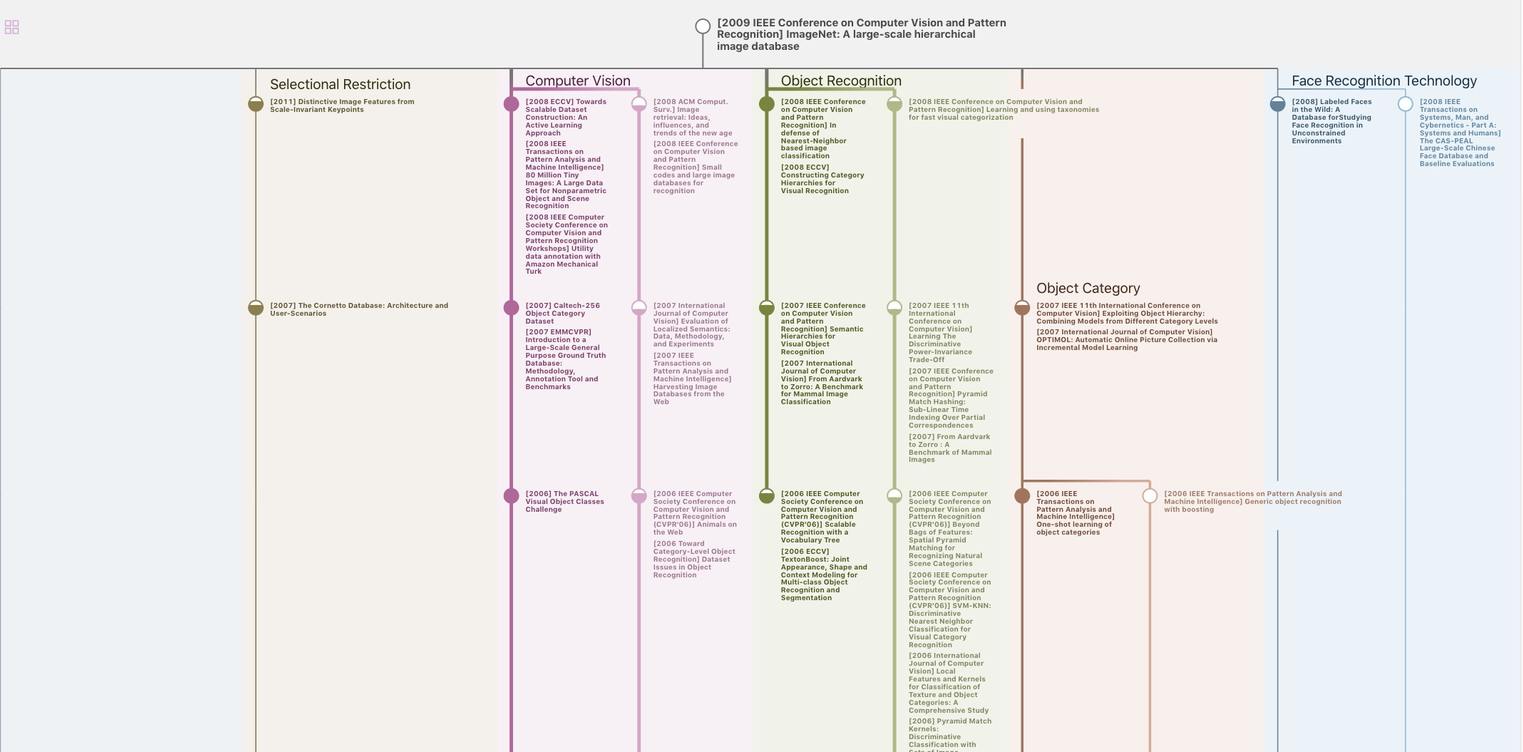
生成溯源树,研究论文发展脉络
Chat Paper
正在生成论文摘要