A Channel-Wise Multi-Scale Network for Single Image Super-Resolution.
IEEE Signal Process. Lett.(2024)
摘要
Existing multi-scale feature extraction methods extract image features using various convolution window sizes conducted on the spatial dimension of the feature maps. However, such an approach inevitably encounters redundant convolution operations. To address this concern, we propose to extract multiscale features on the channel dimension rather than on the spatial dimension. To demonstrate, a channel-wise multi-scale network (CMSN) is proposed for conducting single image super-resolution (SISR). In our CMSN, a sequence of channel-wise multi-scale blocks (CMSBs) is designed to extract multi-scale features at increasing levels by performing convolutions with different channel numbers (i.e., scales). To fuse the image features generated from different levels in our CMSN, a hybrid attention-aware feature fusion block (HAFFB) is proposed. Extensive experimental results have clearly shown the superiority of our CMSN to that of several state-of-the-art SISR methods on delivering superior high-resolution images, both objectively and subjectively. This reveals the potential of channel-wise, versus spatial-wise, on the effectiveness of multi-scale feature extraction.
更多查看译文
关键词
Single image super-resolution,spatial-wise,channel-wise multi-scale feature extraction,attention-aware feature fusion,convolutional neural network
AI 理解论文
溯源树
样例
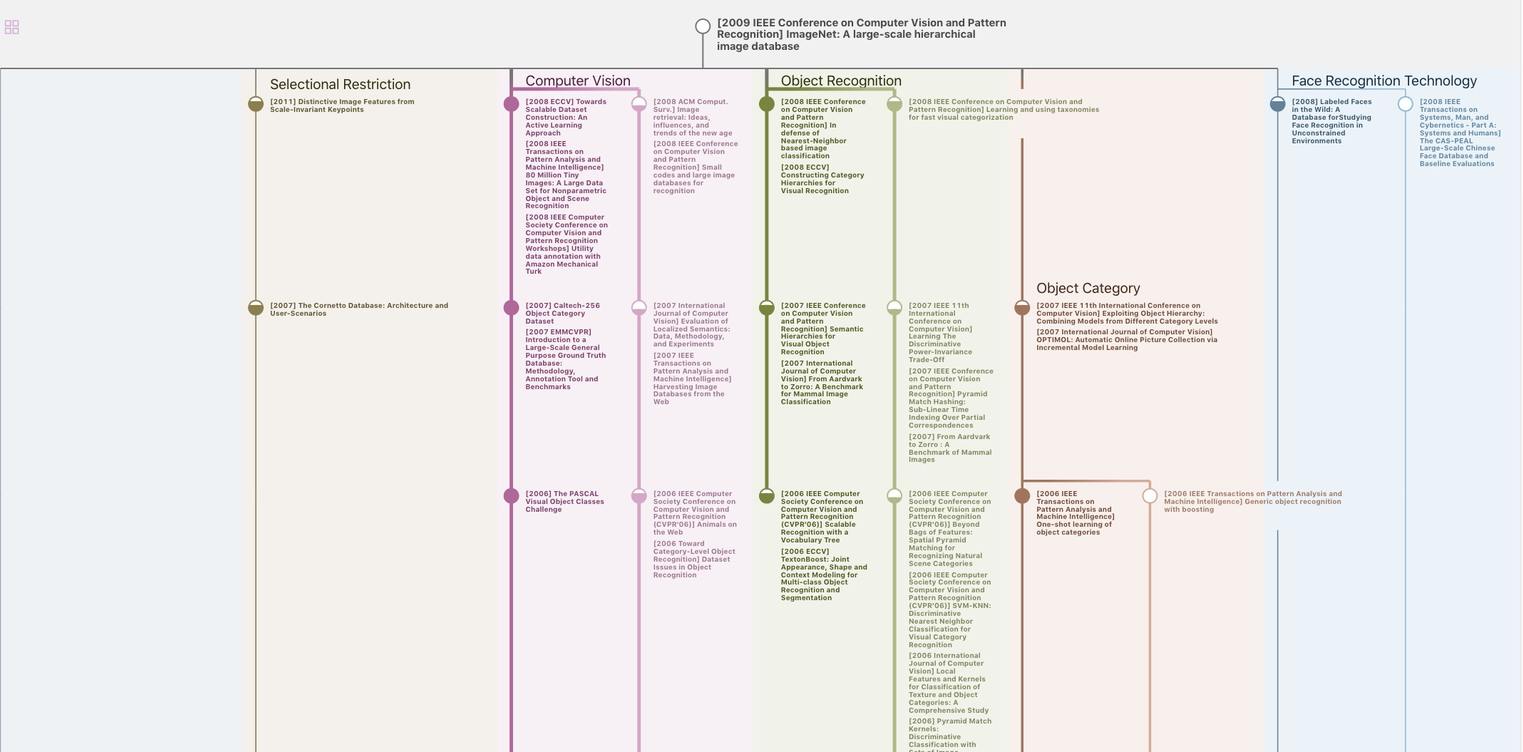
生成溯源树,研究论文发展脉络
Chat Paper
正在生成论文摘要