An Improved Dynamic Window Approach Based on Reinforcement Learning for the Trajectory Planning of Automated Guided Vehicles
IEEE ACCESS(2024)
摘要
The traditional dynamic window approach (DWA) adopts the constant intervals for the sampling window, which limits the trajectory exploration possibility. This paper employs the twin delayed deep deterministic policy gradient (TD3) approach to generate a reinforcement-learning-based auxiliary candidate trajectory with variable sampling mechanism in the prediction domain for the automated guided vehicle (AGV). Subsequently, this auxiliary trajectory would compete with the traditional DWA sampling trajectories in the optimal evaluation. The proposed method significantly reduces computational costs while expanding the search space of the DWA scheme, which improving sampling utilization efficiency and planning effectiveness. In contrast to completed data-driven methods that directly generate planning solutions through the policy networks, the proposed method overall ensures planning effectiveness by the DWA mechanism unit and demonstrates superior generalization capabilities. Simulation results reveal that the proposed reinforcement-learning-based DWA generates the improvement with 11.97% in planning reward and 17.46% in calculation efficiency towards the traditional DWA approach, which demonstrating a significant performance improvement over the traditional DWA method in AGV local planning mission.
更多查看译文
关键词
Adaptive motion planning,dynamic window approach,deep reinforcement learning
AI 理解论文
溯源树
样例
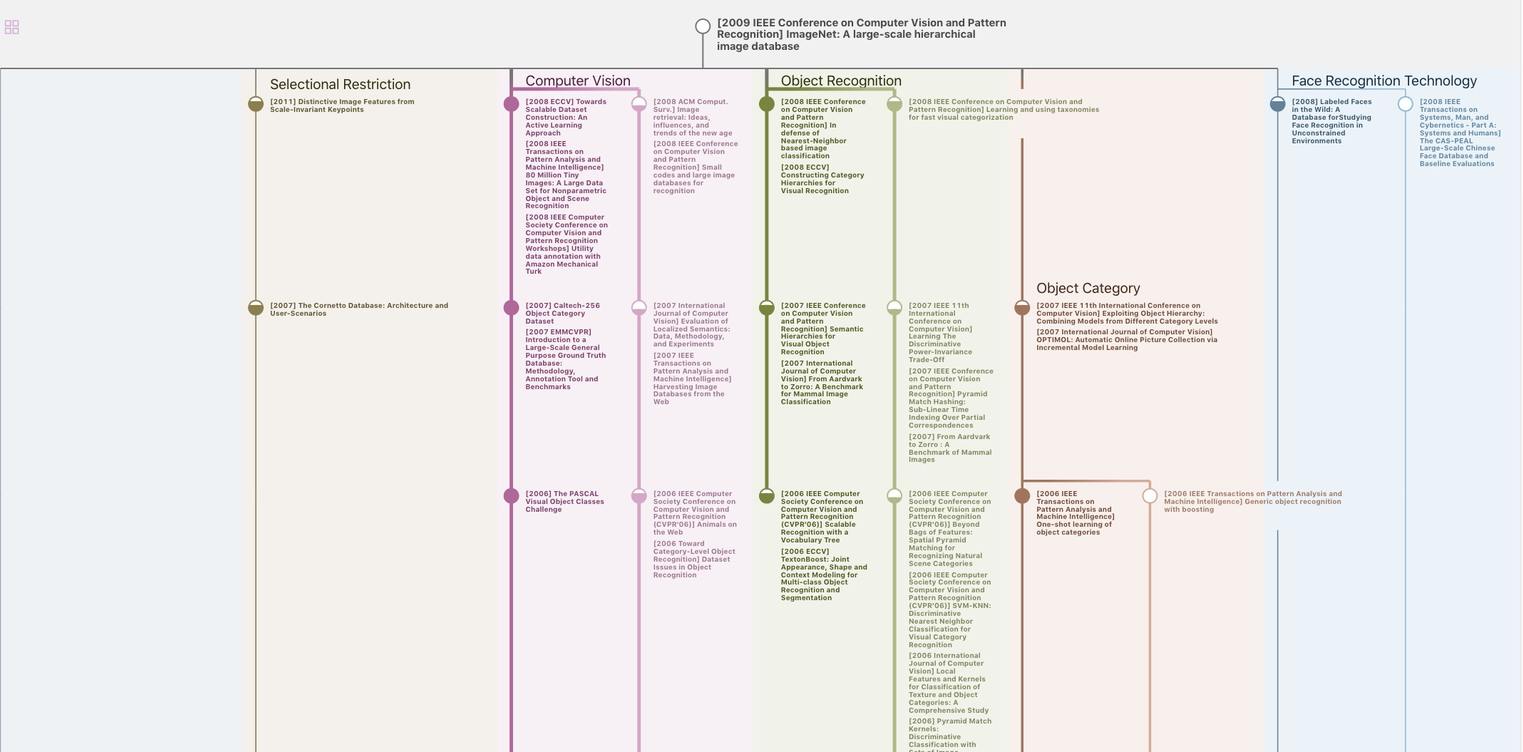
生成溯源树,研究论文发展脉络
Chat Paper
正在生成论文摘要