Intelligent Diagnosis of Rolling Bearing Based on ICEEMDAN-WTD of Noise Reduction and Multi-Strategy Fusion Optimization SCNs
IEEE ACCESS(2024)
摘要
Aiming at the problem of noise interference leading to poor fault diagnosis effect of rolling bearing, a two-stage signal noise reduction method based on multi-strategy coati optimization algorithm (MFCOA) optimized ICEEMDAN combined with wavelet threshold denoising (ICEEMDAN-WTD) is proposed. The MFCOA-optimized stochastic configured networks (MFCOA-SCNs) is used for fault type identification. Firstly, ICEEMDAN is used to decompose noisy signals into several IMF signals, and then a wavelet threshold denoising method is used to process IMF signals whose arrangement entropy is lower than the pre-set value to obtain reconstructed signals. In this process, the ICEEMDAN decomposition is affected by the number of white noise additions and the degree of addition. Therefore, MFCOA is introduced to optimize the parameters to ensure the effect of signal noise reduction. Secondly, ICEEMDAN is applied to the reconstructed signal, and the entropy of IMF signal samples of each order is calculated to obtain the fault characteristics in different simultaneous frequency domains. Finally, the scale factor $\lambda $ and regularization coefficient $r$ in SCNs are optimized by MFCOA, and the sample entropy feature is used as the input of the MFCOA-SCNs model to achieve fault identification. Several simulation experiments have verified that the proposed method can effectively reduce the impact of noise in bearing signals, and the experimental results are better than other methods, and the diagnostic accuracy is better.
更多查看译文
关键词
Coati optimization algorithm,ICCEMDAN,wavelet threshold denoising,stochastic configuration networks,parameter optimization
AI 理解论文
溯源树
样例
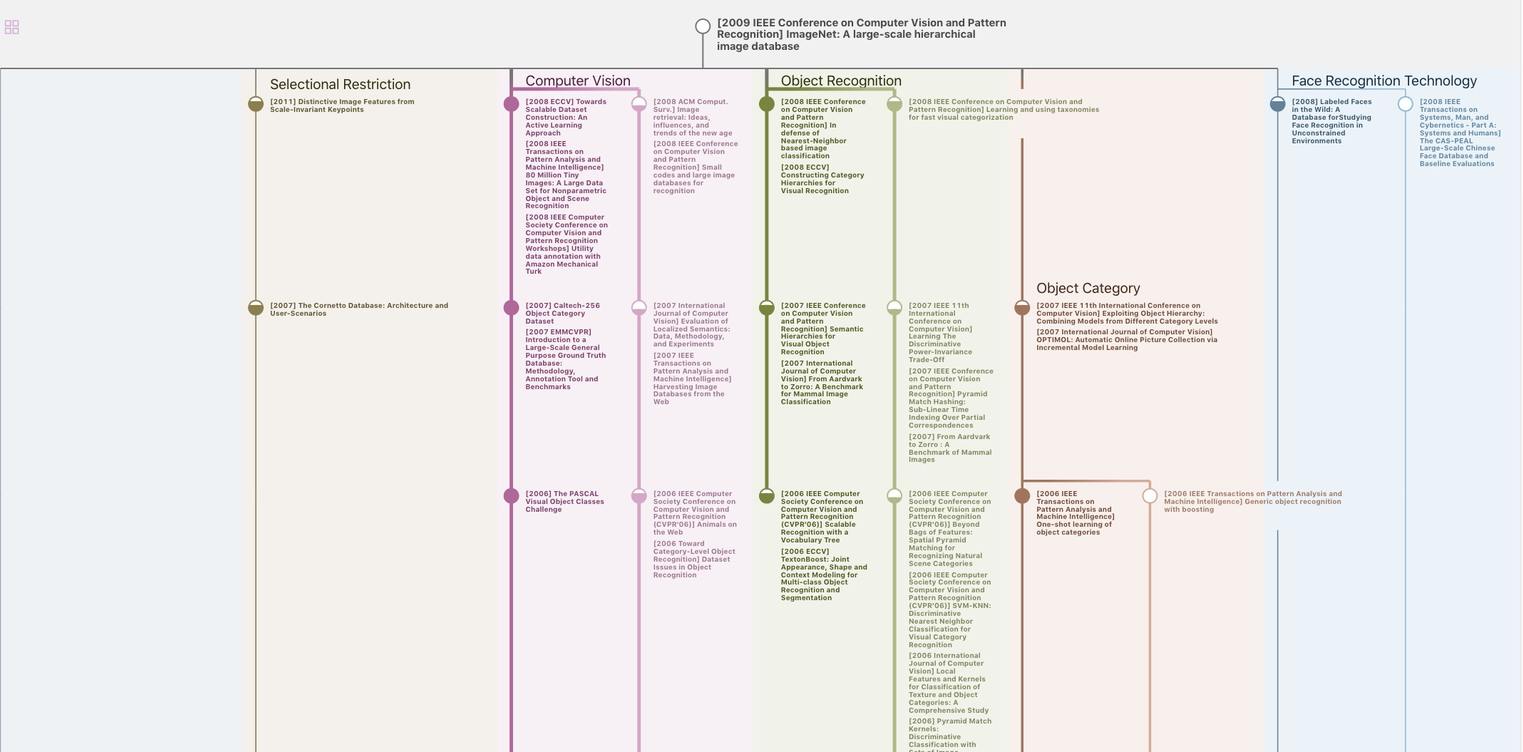
生成溯源树,研究论文发展脉络
Chat Paper
正在生成论文摘要