Less is More: Hop-Wise Graph Attention for Scalable and Generalizable Learning on Circuits
arxiv(2024)
摘要
While graph neural networks (GNNs) have gained popularity for learning
circuit representations in various electronic design automation (EDA) tasks,
they face challenges in scalability when applied to large graphs and exhibit
limited generalizability to new designs. These limitations make them less
practical for addressing large-scale, complex circuit problems. In this work we
propose HOGA, a novel attention-based model for learning circuit
representations in a scalable and generalizable manner. HOGA first computes
hop-wise features per node prior to model training. Subsequently, the hop-wise
features are solely used to produce node representations through a gated
self-attention module, which adaptively learns important features among
different hops without involving the graph topology. As a result, HOGA is
adaptive to various structures across different circuits and can be efficiently
trained in a distributed manner. To demonstrate the efficacy of HOGA, we
consider two representative EDA tasks: quality of results (QoR) prediction and
functional reasoning. Our experimental results indicate that (1) HOGA reduces
estimation error over conventional GNNs by 46.76
logic synthesis; (2) HOGA improves 10.0
identifying functional blocks on unseen gate-level netlists after complex
technology mapping; (3) The training time for HOGA almost linearly decreases
with an increase in computing resources.
更多查看译文
AI 理解论文
溯源树
样例
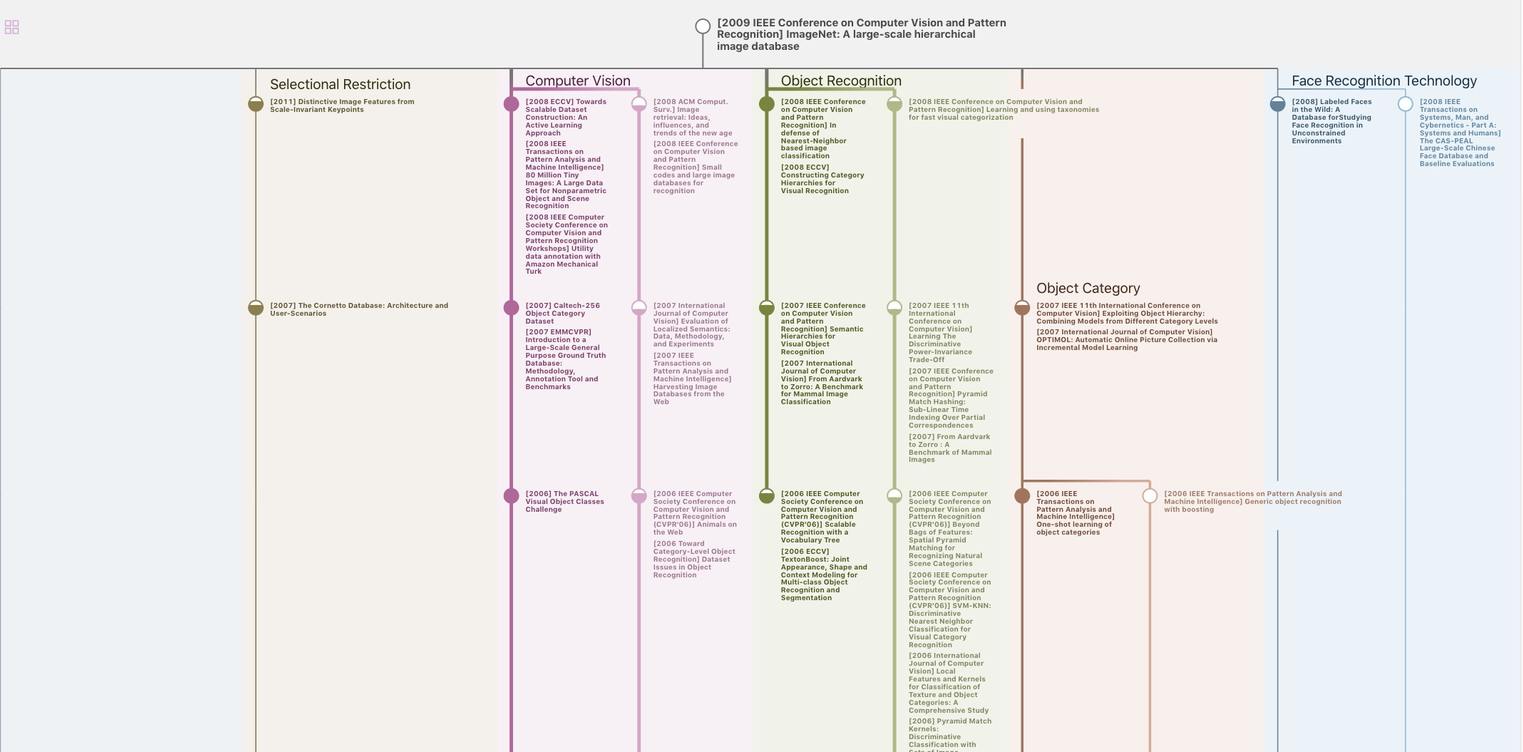
生成溯源树,研究论文发展脉络
Chat Paper
正在生成论文摘要