Stochastic gradient descent for streaming linear and rectified linear systems with Massart noise
arxiv(2024)
摘要
We propose SGD-exp, a stochastic gradient descent approach for linear and
ReLU regressions under Massart noise (adversarial semi-random corruption model)
for the fully streaming setting. We show novel nearly linear convergence
guarantees of SGD-exp to the true parameter with up to 50% Massart
corruption rate, and with any corruption rate in the case of symmetric
oblivious corruptions. This is the first convergence guarantee result for
robust ReLU regression in the streaming setting, and it shows the improved
convergence rate over previous robust methods for L_1 linear regression due
to a choice of an exponentially decaying step size, known for its efficiency in
practice. Our analysis is based on the drift analysis of a discrete stochastic
process, which could also be interesting on its own.
更多查看译文
AI 理解论文
溯源树
样例
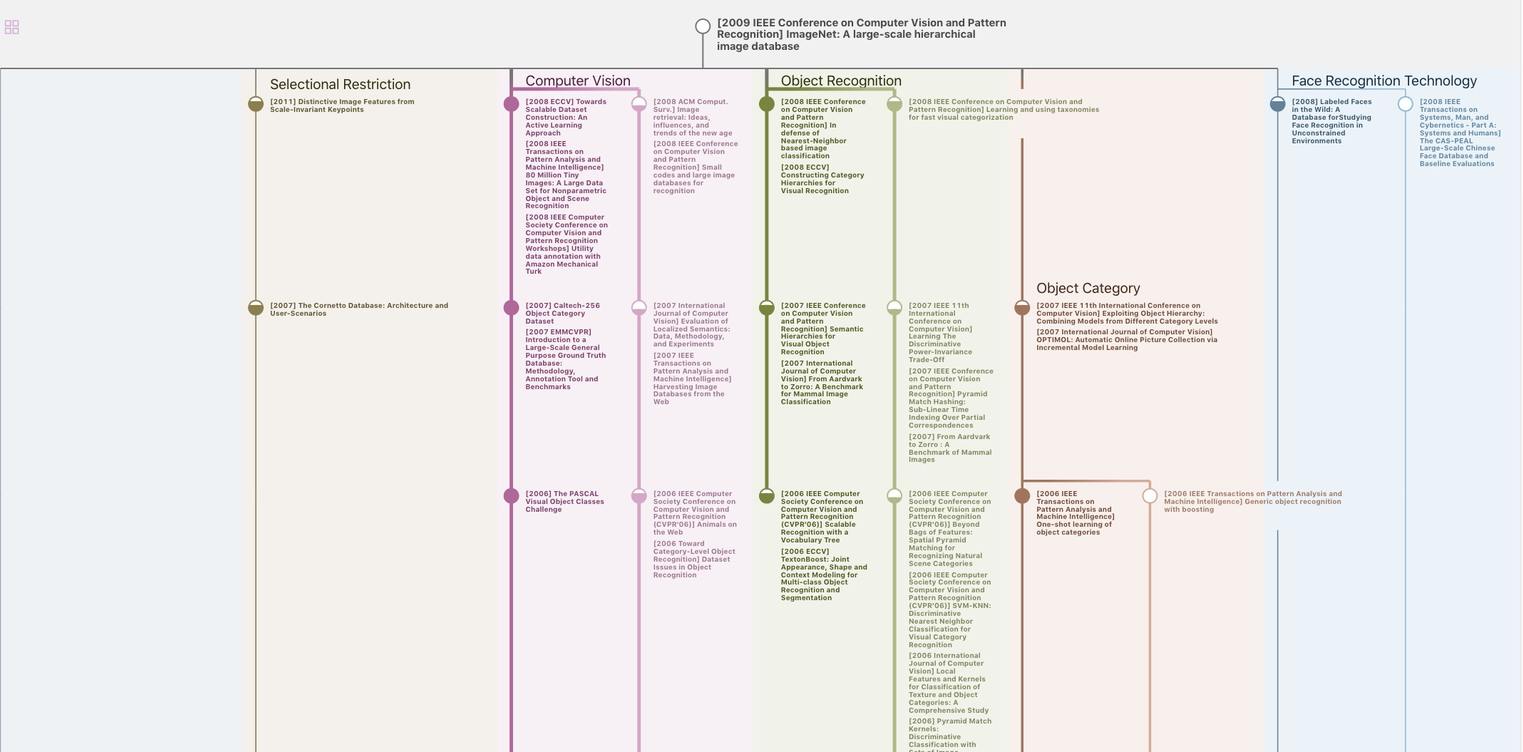
生成溯源树,研究论文发展脉络
Chat Paper
正在生成论文摘要