Pseudo-Label Calibration Semi-supervised Multi-Modal Entity Alignment
AAAI 2024(2024)
摘要
Multi-modal entity alignment (MMEA) aims to identify equivalent entities between two multi-modal knowledge graphs for integration. Unfortunately, prior arts have attempted to improve the interaction and fusion of multi-modal information, which have overlooked the influence of modal-specific noise and the usage of labeled and unlabeled data in semi-supervised settings. In this work, we introduce a Pseudo-label Calibration Multi-modal Entity Alignment (PCMEA) in a semi-supervised way. Specifically, in order to generate holistic entity representations, we first devise various embedding modules and attention mechanisms to extract visual, structural, relational, and attribute features. Different from the prior direct fusion methods, we next propose to exploit mutual information maximization to filter the modal-specific noise and to augment modal-invariant commonality. Then, we combine pseudo-label calibration with momentum-based contrastive learning to make full use of the labeled and unlabeled data, which improves the quality of pseudo-label and pulls aligned entities closer. Finally, extensive experiments on two MMEA datasets demonstrate the effectiveness of our PCMEA, which yields state-of-the-art performance.
更多查看译文
关键词
DMKM: Linked Open Data, Knowledge Graphs & KB Completio,DMKM: Mining of Visual, Multimedia & Multimodal Data
AI 理解论文
溯源树
样例
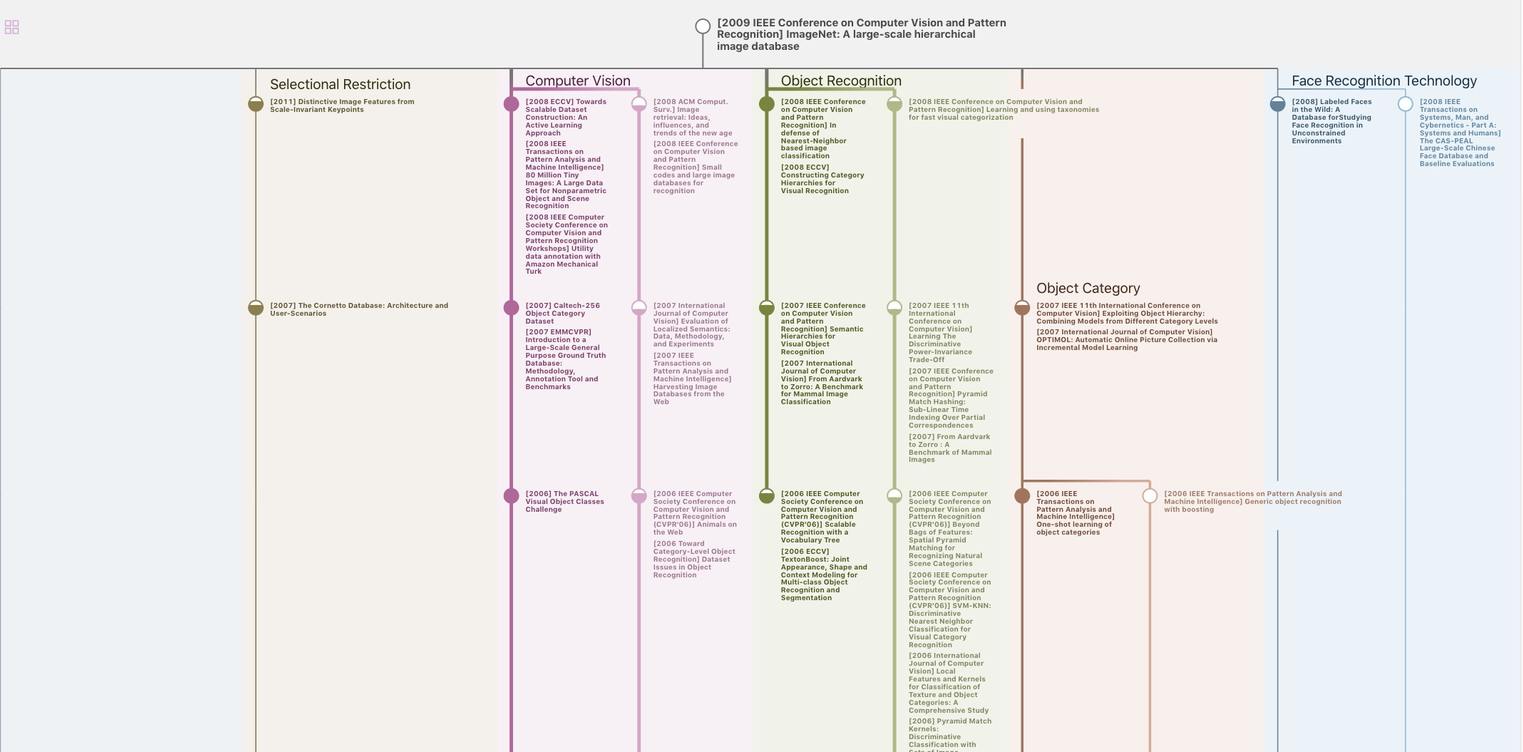
生成溯源树,研究论文发展脉络
Chat Paper
正在生成论文摘要