Neural Field Classifiers via Target Encoding and Classification Loss
ICLR 2024(2024)
摘要
Neural field methods have seen great progress in various long-standing tasks
in computer vision and computer graphics, including novel view synthesis and
geometry reconstruction. As existing neural field methods try to predict some
coordinate-based continuous target values, such as RGB for Neural Radiance
Field (NeRF), all of these methods are regression models and are optimized by
some regression loss. However, are regression models really better than
classification models for neural field methods? In this work, we try to visit
this very fundamental but overlooked question for neural fields from a machine
learning perspective. We successfully propose a novel Neural Field Classifier
(NFC) framework which formulates existing neural field methods as
classification tasks rather than regression tasks. The proposed NFC can easily
transform arbitrary Neural Field Regressor (NFR) into its classification
variant via employing a novel Target Encoding module and optimizing a
classification loss. By encoding a continuous regression target into a
high-dimensional discrete encoding, we naturally formulate a multi-label
classification task. Extensive experiments demonstrate the impressive
effectiveness of NFC at the nearly free extra computational costs. Moreover,
NFC also shows robustness to sparse inputs, corrupted images, and dynamic
scenes.
更多查看译文
关键词
Neural Fields,NeRF,3D Vision,Scene Reconstruction
AI 理解论文
溯源树
样例
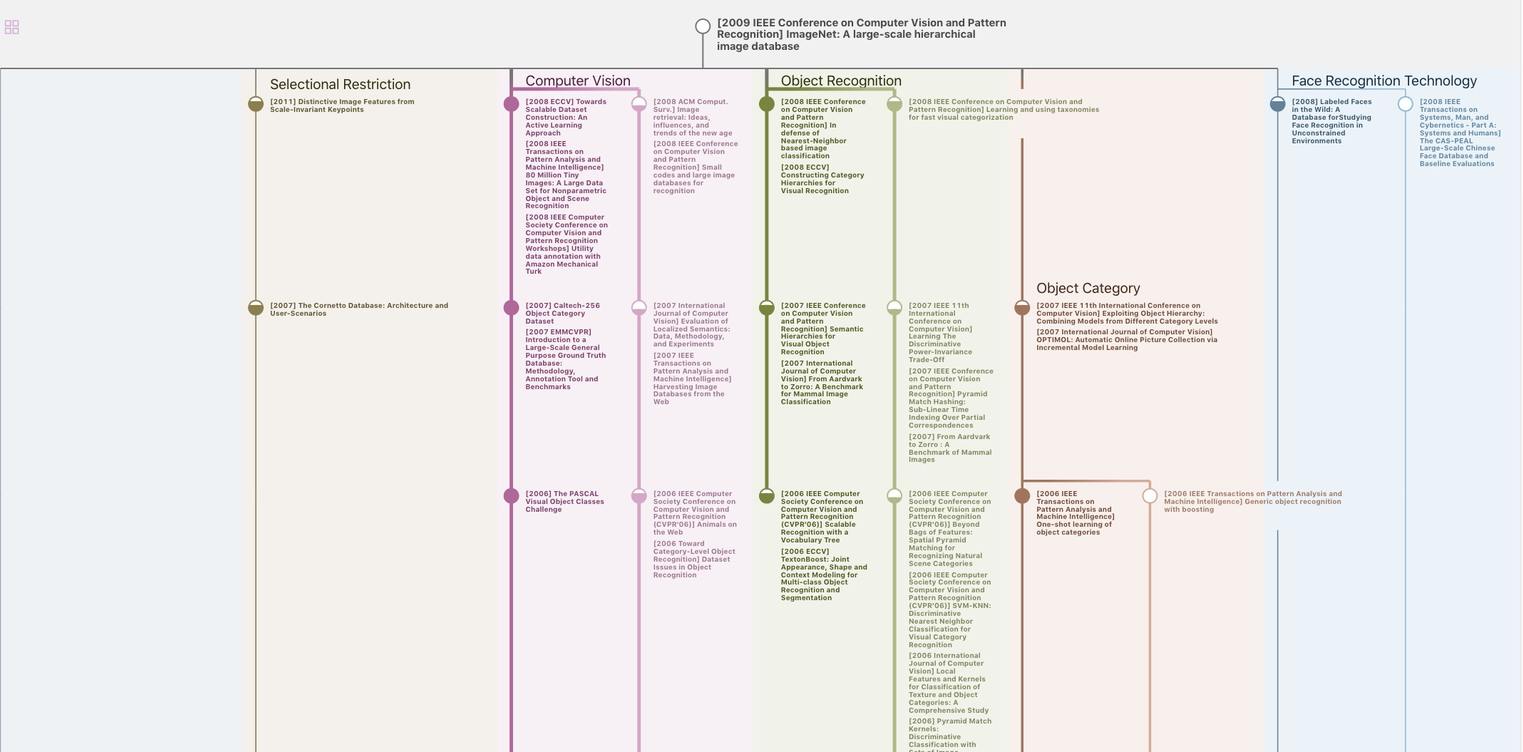
生成溯源树,研究论文发展脉络
Chat Paper
正在生成论文摘要