Multi-level multilingual semantic alignment for zero-shot cross-lingual transfer learning
NEURAL NETWORKS(2024)
摘要
Recently, cross -lingual transfer learning has attracted extensive attention from both academia and industry. Previous studies usually focus only on the single -level alignment (e.g., word -level, sentence -level), based on pretrained language models. However, it leads to suboptimal performance in downstream tasks of the low -resource language due to the missing correlation of hierarchical semantic information (e.g., sentence -to -word, word -toword). Therefore, in this paper, we propose a novel multi -level alignment framework, which hierarchically learns the semantic correlation between multiple levels by leveraging well -designed alignment training tasks. In addition, we devise an attention -based fusion mechanism (AFM) to infuse semantic information from high levels. Extensive experiments on mainstream cross -lingual tasks (e.g., text classification, paraphrase identification, and named entity recognition) demonstrate the effectiveness of our proposed method, and also show that our model achieves state-of-the-art performance across various benchmarks compared to other strong baselines.
更多查看译文
关键词
Multilingual semantic alignment,Cross-lingual transfer learning
AI 理解论文
溯源树
样例
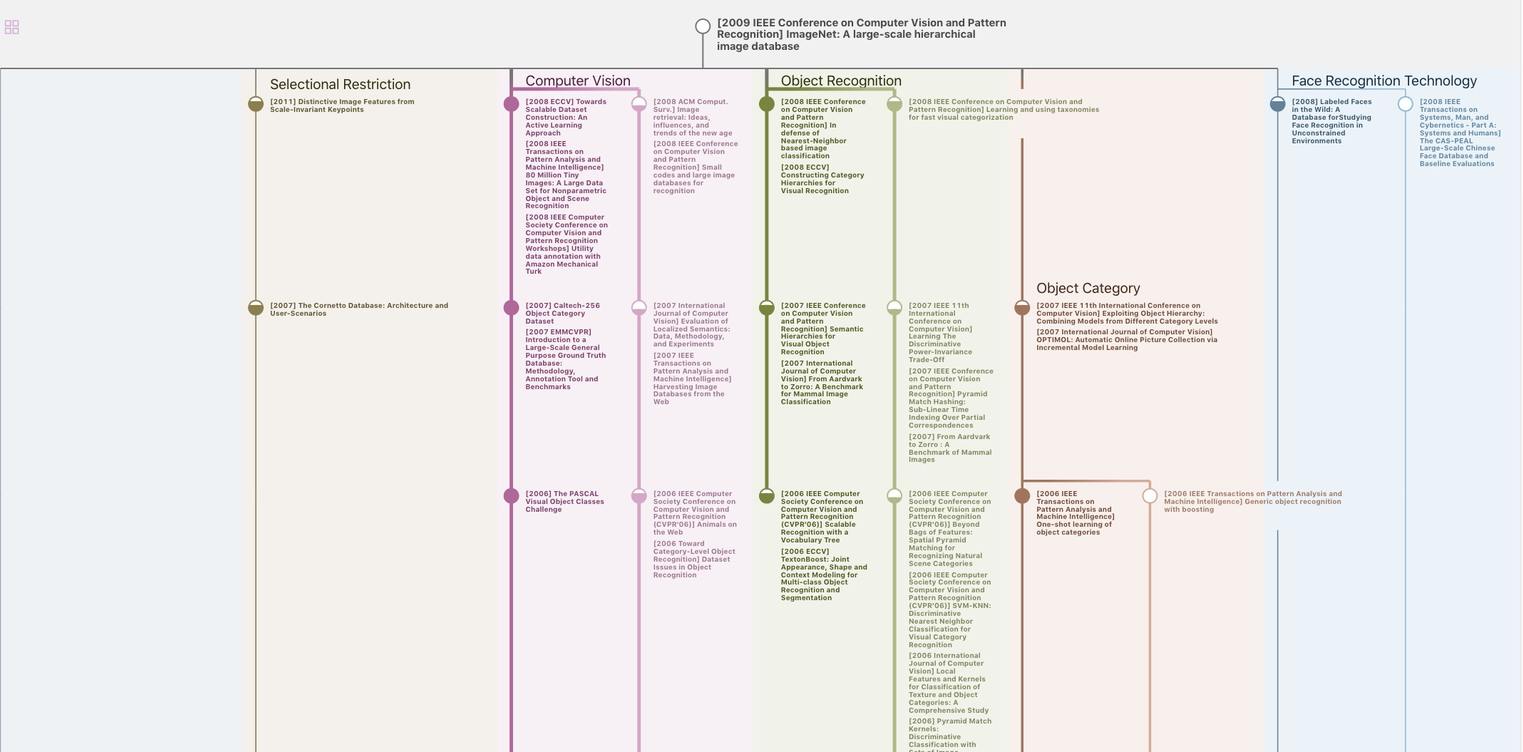
生成溯源树,研究论文发展脉络
Chat Paper
正在生成论文摘要