CDSKNNXMBD: a novel clustering framework for large-scale single-cell data based on a stable graph structure
Journal of Translational Medicine(2024)
摘要
Accurate and efficient cell grouping is essential for analyzing single-cell transcriptome sequencing (scRNA-seq) data. However, the existing clustering techniques often struggle to provide timely and accurate cell type groupings when dealing with datasets with large-scale or imbalanced cell types. Therefore, there is a need for improved methods that can handle the increasing size of scRNA-seq datasets while maintaining high accuracy and efficiency. We propose CDSKNNXMBD (Community Detection based on a Stable K-Nearest Neighbor Graph Structure), a novel single-cell clustering framework integrating partition clustering algorithm and community detection algorithm, which achieves accurate and fast cell type grouping by finding a stable graph structure. We evaluated the effectiveness of our approach by analyzing 15 tissues from the human fetal atlas. Compared to existing methods, CDSKNN effectively counteracts the high imbalance in single-cell data, enabling effective clustering. Furthermore, we conducted comparisons across multiple single-cell datasets from different studies and sequencing techniques. CDSKNN is of high applicability and robustness, and capable of balancing the complexities of across diverse types of data. Most importantly, CDSKNN exhibits higher operational efficiency on datasets at the million-cell scale, requiring an average of only 6.33 min for clustering 1.46 million single cells, saving 33.3
更多查看译文
关键词
scRNA-seq,Clustering,Large-scale,Imbalance ratio
AI 理解论文
溯源树
样例
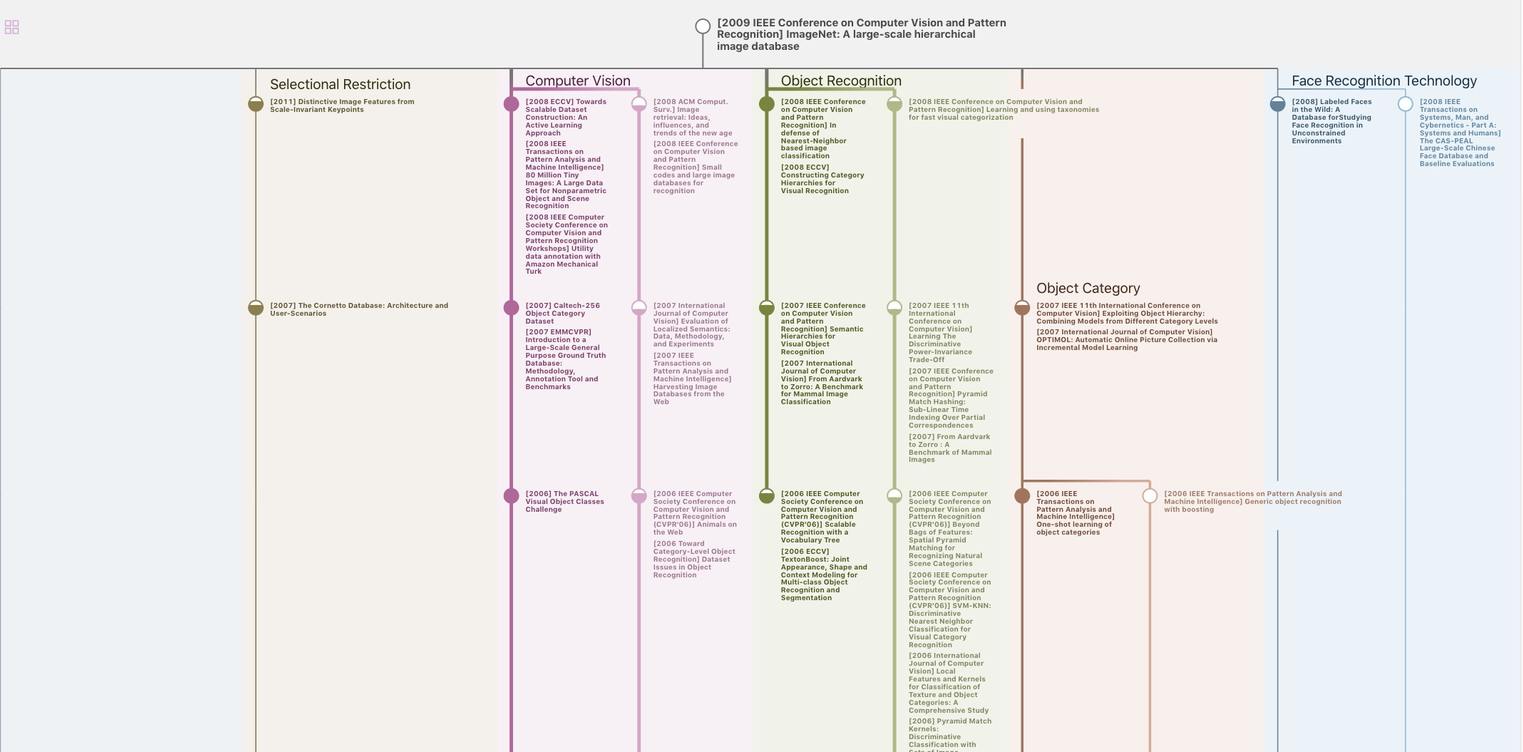
生成溯源树,研究论文发展脉络
Chat Paper
正在生成论文摘要