Self-Consistent Decoding for More Factual Open Responses
arxiv(2024)
摘要
Self-consistency has emerged as a powerful method for improving the accuracy
of short answers generated by large language models. As previously defined, it
only concerns the accuracy of a final answer parsed from generated text. In
this work, we extend the idea to open response generation, by integrating
voting into the decoding method. Each output sentence is selected from among
multiple samples, conditioning on the previous selections, based on a simple
token overlap score. We compare this "Sample Select" method to greedy
decoding, beam search, nucleus sampling, and the recently introduced
hallucination avoiding decoders of DoLA, P-CRR, and S-CRR. We show that Sample
Select improves factuality by a 30
NLI-based evaluation on the subsets of CNN/DM and XSum used in the FRANK
benchmark, while maintaining comparable ROUGE-1 F1 scores against reference
summaries. We collect human verifications of the generated summaries,
confirming the factual superiority of our method.
更多查看译文
AI 理解论文
溯源树
样例
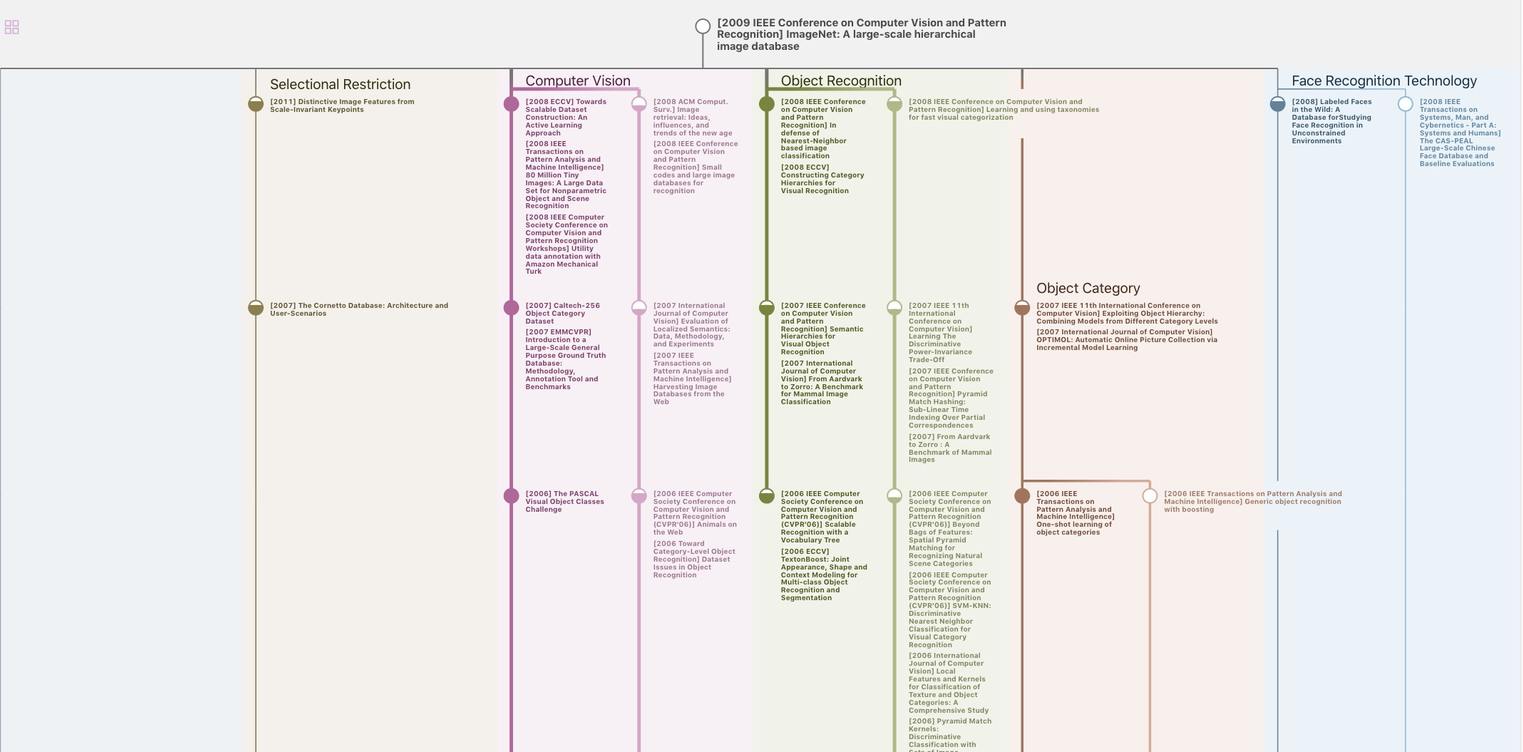
生成溯源树,研究论文发展脉络
Chat Paper
正在生成论文摘要