Standardizing the Measurement of Text Diversity: A Tool and a Comparative Analysis of Scores
arxiv(2024)
摘要
The diversity across outputs generated by large language models shapes the
perception of their quality and utility. Prompt leaks, templated answer
structure, and canned responses across different interactions are readily
noticed by people, but there is no standard score to measure this aspect of
model behavior. In this work we empirically investigate diversity scores on
English texts. We find that computationally efficient compression algorithms
capture information similar to what is measured by slow to compute n-gram
overlap homogeneity scores. Further, a combination of measures – compression
ratios, self-repetition of long n-grams and Self-BLEU and BERTScore – are
sufficient to report, as they have low mutual correlation with each other. The
applicability of scores extends beyond analysis of generative models; for
example, we highlight applications on instruction-tuning datasets and
human-produced texts. We release a diversity score package to facilitate
research and invite consistency across reports.
更多查看译文
AI 理解论文
溯源树
样例
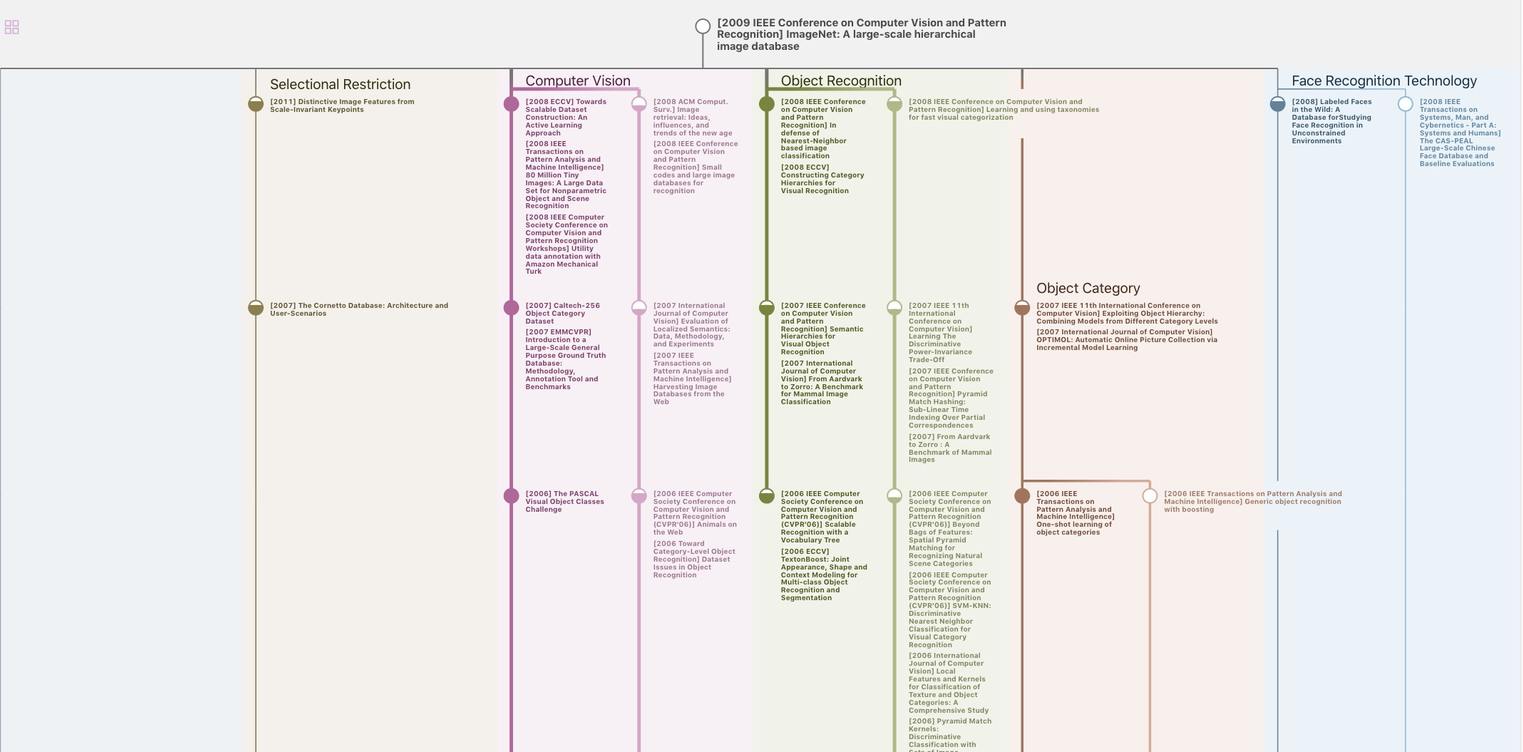
生成溯源树,研究论文发展脉络
Chat Paper
正在生成论文摘要