SURE: SUrvey REcipes for building reliable and robust deep networks
CVPR 2024(2024)
摘要
In this paper, we revisit techniques for uncertainty estimation within deep
neural networks and consolidate a suite of techniques to enhance their
reliability. Our investigation reveals that an integrated application of
diverse techniques–spanning model regularization, classifier and
optimization–substantially improves the accuracy of uncertainty predictions in
image classification tasks. The synergistic effect of these techniques
culminates in our novel SURE approach. We rigorously evaluate SURE against the
benchmark of failure prediction, a critical testbed for uncertainty estimation
efficacy. Our results showcase a consistently better performance than models
that individually deploy each technique, across various datasets and model
architectures. When applied to real-world challenges, such as data corruption,
label noise, and long-tailed class distribution, SURE exhibits remarkable
robustness, delivering results that are superior or on par with current
state-of-the-art specialized methods. Particularly on Animal-10N and Food-101N
for learning with noisy labels, SURE achieves state-of-the-art performance
without any task-specific adjustments. This work not only sets a new benchmark
for robust uncertainty estimation but also paves the way for its application in
diverse, real-world scenarios where reliability is paramount. Our code is
available at .
更多查看译文
AI 理解论文
溯源树
样例
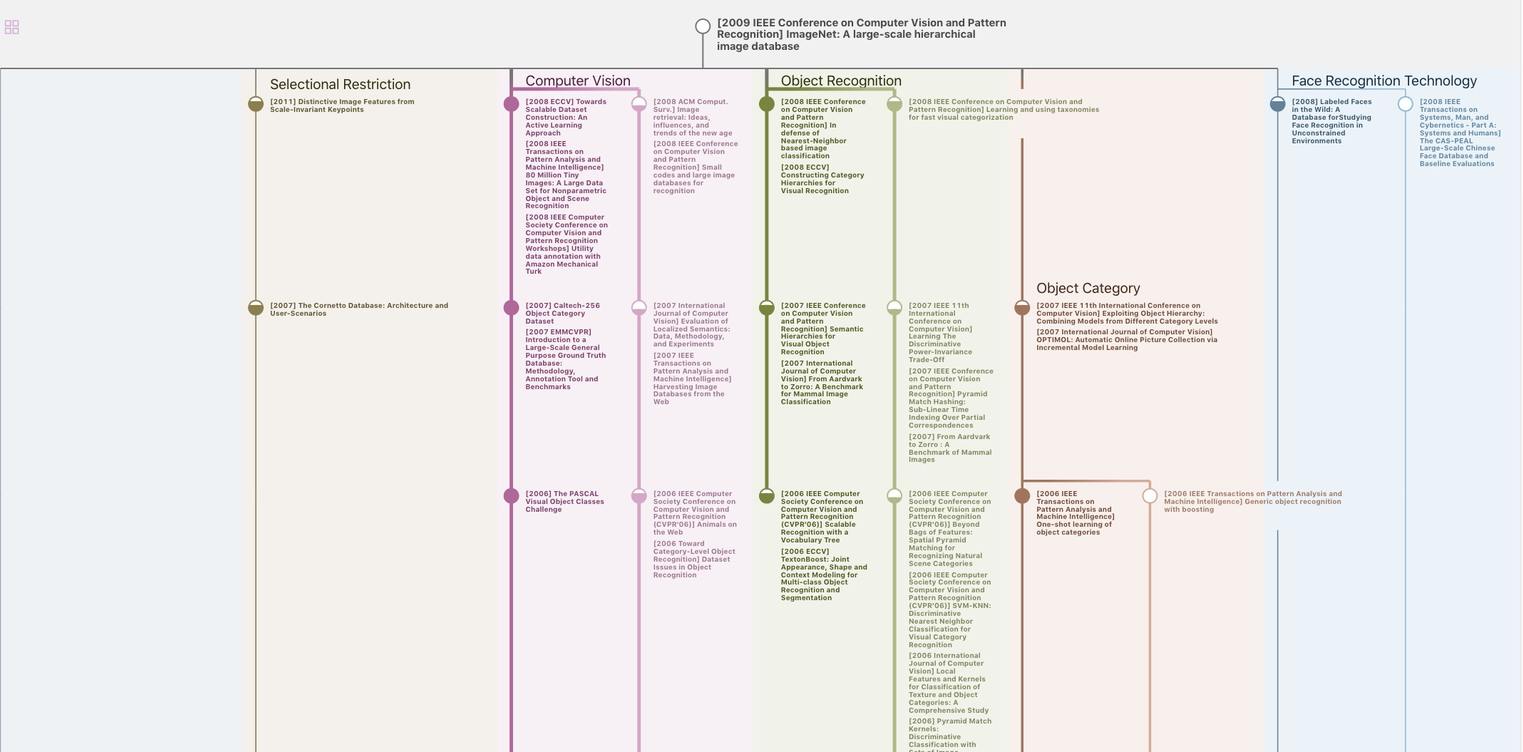
生成溯源树,研究论文发展脉络
Chat Paper
正在生成论文摘要