A time-stepping deep gradient flow method for option pricing in (rough) diffusion models
arxiv(2024)
摘要
We develop a novel deep learning approach for pricing European options in
diffusion models, that can efficiently handle high-dimensional problems
resulting from Markovian approximations of rough volatility models. The option
pricing partial differential equation is reformulated as an energy minimization
problem, which is approximated in a time-stepping fashion by deep artificial
neural networks. The proposed scheme respects the asymptotic behavior of option
prices for large levels of moneyness, and adheres to a priori known bounds for
option prices. The accuracy and efficiency of the proposed method is assessed
in a series of numerical examples, with particular focus in the lifted Heston
model.
更多查看译文
AI 理解论文
溯源树
样例
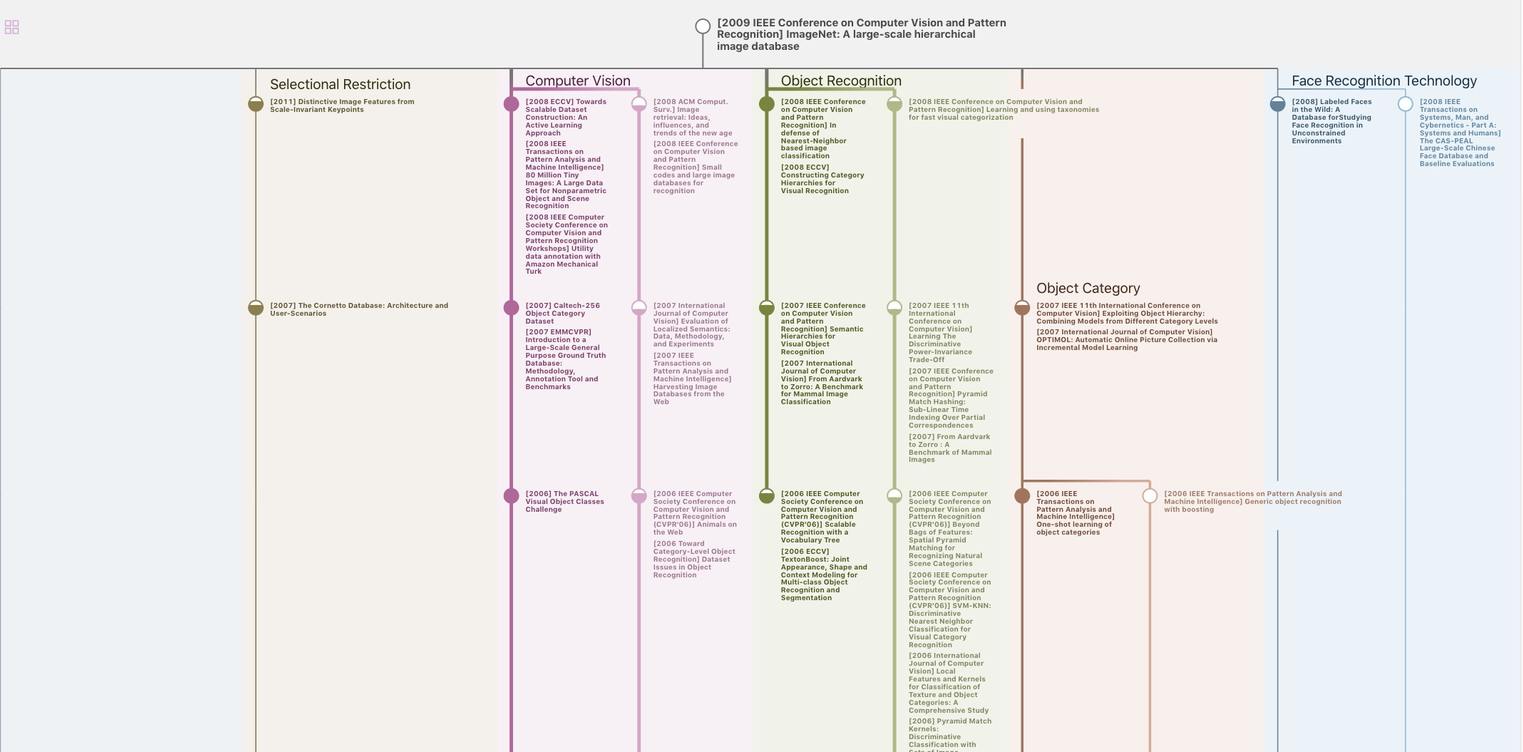
生成溯源树,研究论文发展脉络
Chat Paper
正在生成论文摘要