Revisiting Disentanglement in Downstream Tasks: A Study on Its Necessity for Abstract Visual Reasoning
AAAI 2024(2024)
摘要
In representation learning, a disentangled representation is highly desirable as it encodes generative factors of data in a separable and compact pattern. Researchers have advocated leveraging disentangled representations to complete downstream tasks with encouraging empirical evidence. This paper further investigates the necessity of disentangled representation in downstream applications. Specifically, we show that dimension-wise disentangled representations are unnecessary on a fundamental downstream task, abstract visual reasoning. We provide extensive empirical evidence against the necessity of disentanglement, covering multiple datasets, representation learning methods, and downstream network architectures. Furthermore, our findings suggest that the informativeness of representations is a better indicator of downstream performance than disentanglement. Finally, the positive correlation between informativeness and disentanglement explains the claimed usefulness of disentangled representations in previous works. The source code is available at https://github.com/Richard-coder-Nai/disentanglement-lib-necessity.git
更多查看译文
关键词
ML: Representation Learning
AI 理解论文
溯源树
样例
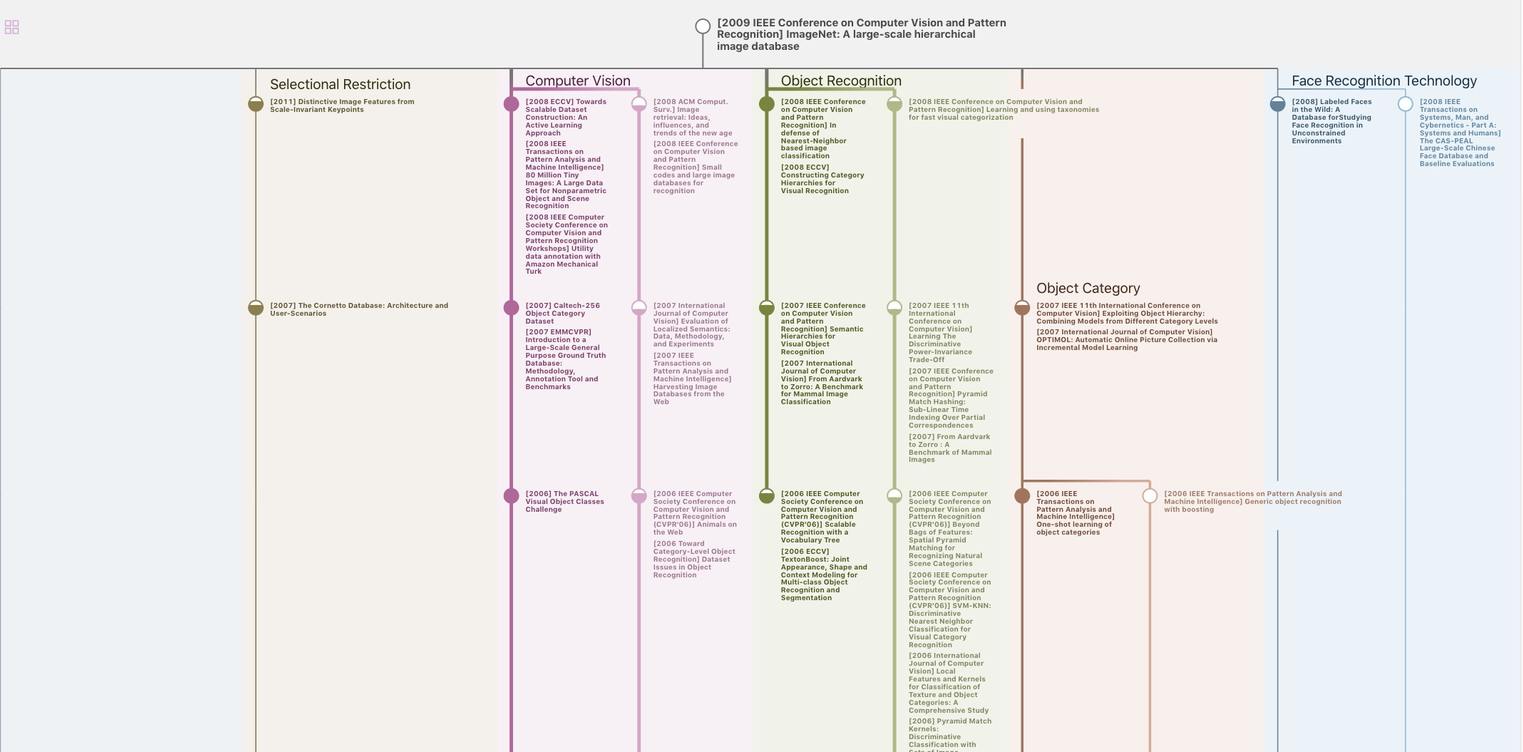
生成溯源树,研究论文发展脉络
Chat Paper
正在生成论文摘要