Scale-Invariant Gradient Aggregation for Constrained Multi-Objective Reinforcement Learning
arxiv(2024)
摘要
Multi-objective reinforcement learning (MORL) aims to find a set of Pareto
optimal policies to cover various preferences. However, to apply MORL in
real-world applications, it is important to find policies that are not only
Pareto optimal but also satisfy pre-defined constraints for safety. To this
end, we propose a constrained MORL (CMORL) algorithm called Constrained
Multi-Objective Gradient Aggregator (CoMOGA). Recognizing the difficulty of
handling multiple objectives and constraints concurrently, CoMOGA relaxes the
original CMORL problem into a constrained optimization problem by transforming
the objectives into additional constraints. This novel transformation process
ensures that the converted constraints are invariant to the objective scales
while having the same effect as the original objectives. We show that the
proposed method converges to a local Pareto optimal policy while satisfying the
predefined constraints. Empirical evaluations across various tasks show that
the proposed method outperforms other baselines by consistently meeting
constraints and demonstrating invariance to the objective scales.
更多查看译文
AI 理解论文
溯源树
样例
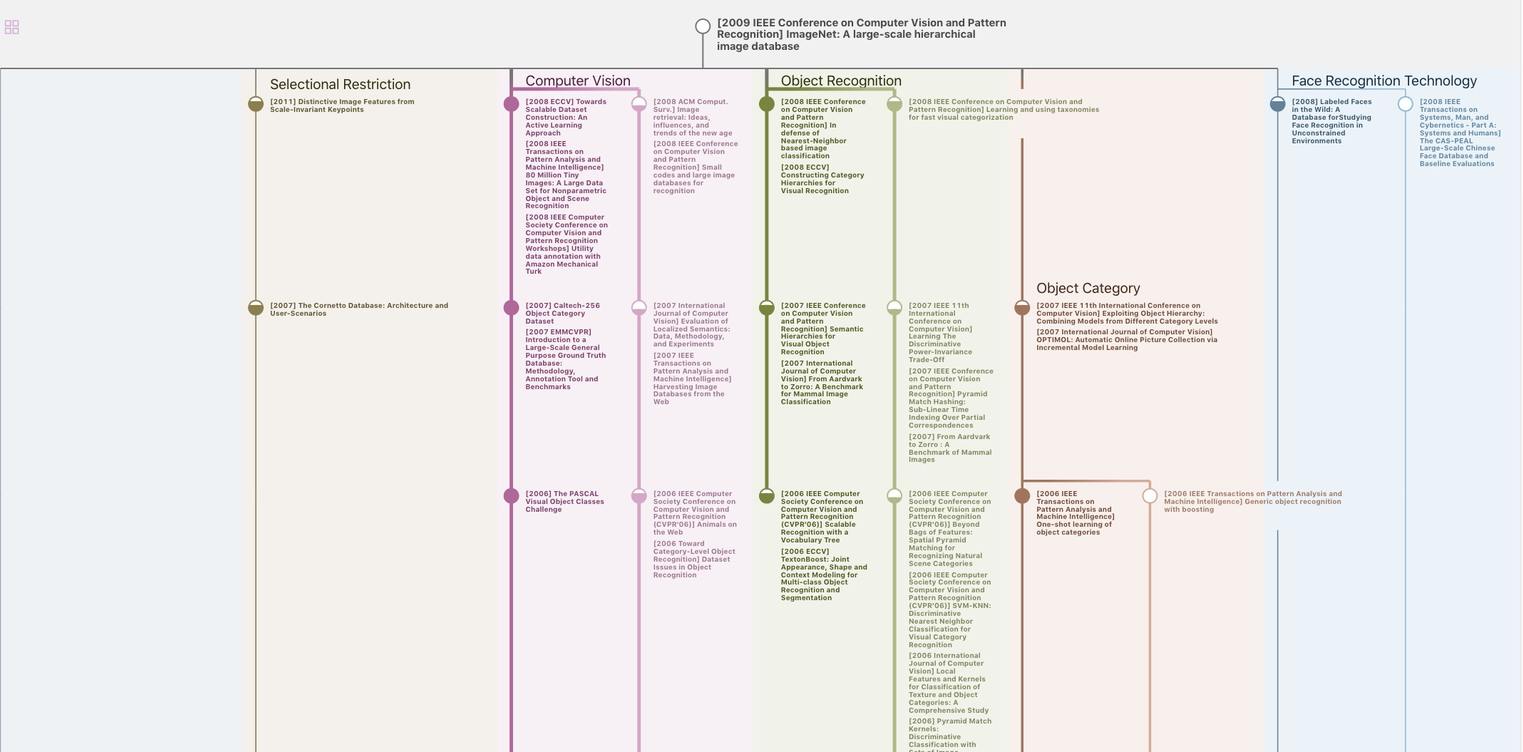
生成溯源树,研究论文发展脉络
Chat Paper
正在生成论文摘要