Transformer-based Parameter Estimation in Statistics
Mathematics(2024)
摘要
Parameter estimation is one of the most important tasks in statistics, and is
key to helping people understand the distribution behind a sample of
observations. Traditionally parameter estimation is done either by closed-form
solutions (e.g., maximum likelihood estimation for Gaussian distribution), or
by iterative numerical methods such as Newton-Raphson method when closed-form
solution does not exist (e.g., for Beta distribution).
In this paper we propose a transformer-based approach to parameter
estimation. Compared with existing solutions, our approach does not require a
closed-form solution or any mathematical derivations. It does not even require
knowing the probability density function, which is needed by numerical methods.
After the transformer model is trained, only a single inference is needed to
estimate the parameters of the underlying distribution based on a sample of
observations. In the empirical study we compared our approach with maximum
likelihood estimation on commonly used distributions such as normal
distribution, exponential distribution and beta distribution. It is shown that
our approach achieves similar or better accuracy as measured by
mean-square-errors.
更多查看译文
关键词
parameter estimation,transformer,deep learning
AI 理解论文
溯源树
样例
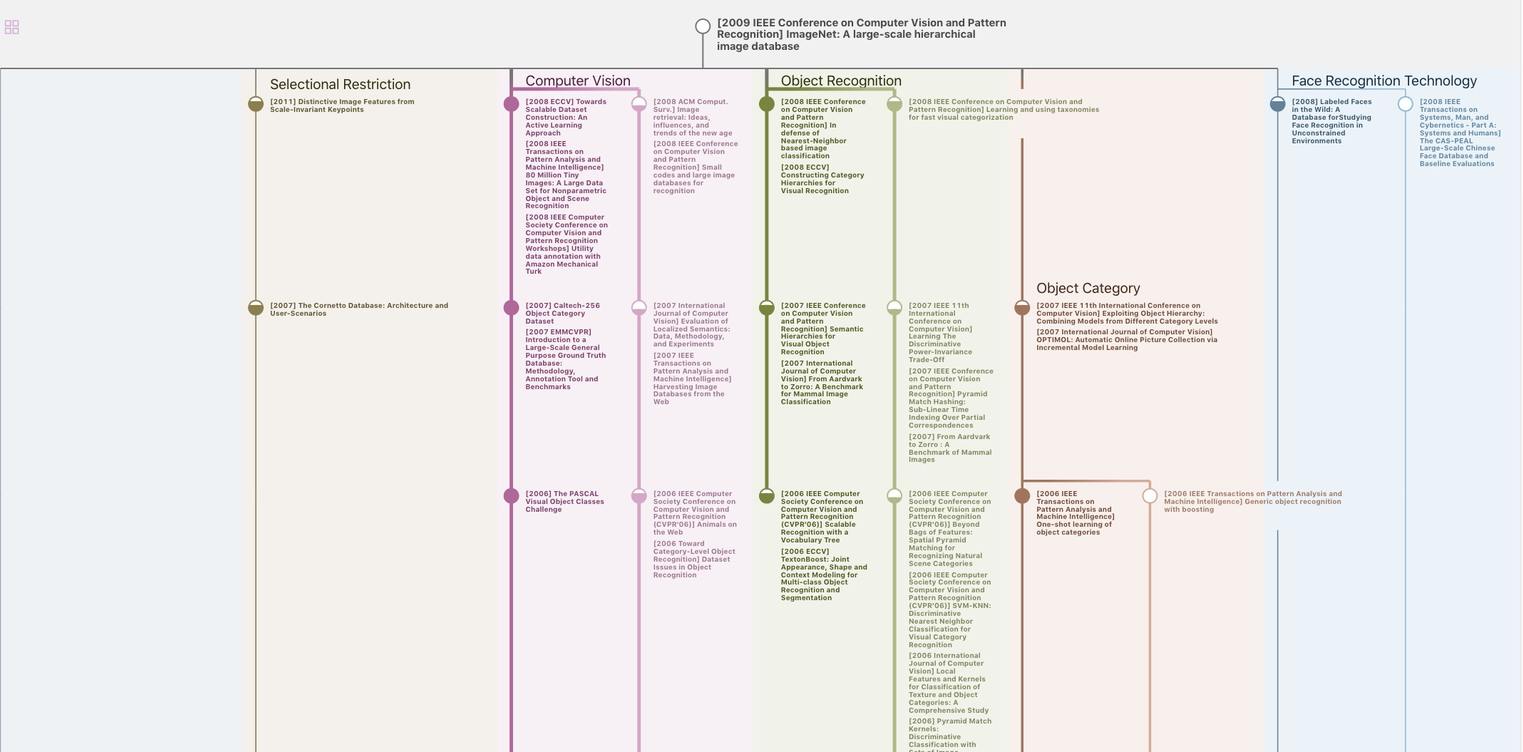
生成溯源树,研究论文发展脉络
Chat Paper
正在生成论文摘要