Meta-evaluation for 3D Face Reconstruction Via Synthetic Data
2023 IEEE INTERNATIONAL JOINT CONFERENCE ON BIOMETRICS, IJCB(2023)
摘要
The standard benchmark metric for 3D face reconstruction is the geometric error between reconstructed meshes and the ground truth. Nearly all recent reconstruction methods are validated on real ground truth scans, in which case one needs to establish point correspondence prior to error computation, which is typically done with the Chamfer (i.e., nearest neighbor) criterion. However, a simple yet fundamental question have not been asked: Is the Chamfer error an appropriate and fair benchmark metric for 3D face reconstruction? More generally, how can we determine which error estimator is a better benchmark metric? We present a meta-evaluation framework that uses synthetic data to evaluate the quality of a geometric error estimator as a benchmark metric for face reconstruction. Further, we use this framework to experimentally compare four geometric error estimators. Results show that the standard approach not only severely underestimates the error, but also does so inconsistently across reconstruction methods, to the point of even altering the ranking of the compared methods. Moreover, although non-rigid ICP leads to a metric with smaller estimation bias, it could still not correctly rank all compared reconstruction methods, and is significantly more time consuming than Chamfer. In sum, we show several issues present in the current benchmarking and propose a procedure using synthetic data to address these issues.
更多查看译文
关键词
Oral And Maxillofacial Surgery,3D Face,3D Face Reconstruction,Estimation Error,Biased Estimates,3D Reconstruction,Reconstruction Method,Corresponding Points,Standard Benchmark,Geometric Errors,Current Benchmark,Variance Estimates,Facial Features,3D Scanning,Mesh Network,Ranking Method,Point Index,True Error,Tip Of The Nose,Unfair Advantage,Evaluation Of Different Methods,Shape Coefficient,Chamfer Distance,Subjective Errors
AI 理解论文
溯源树
样例
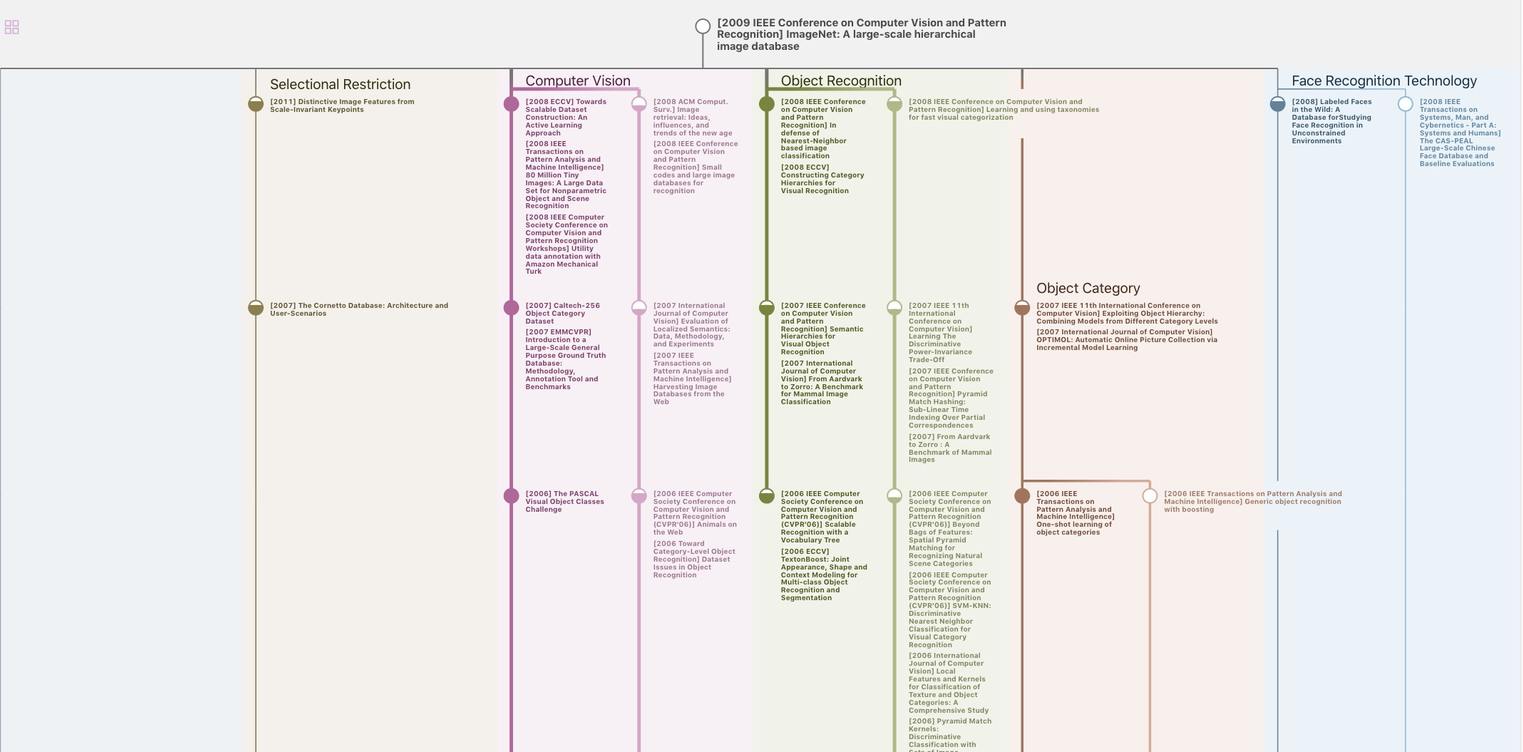
生成溯源树,研究论文发展脉络
Chat Paper
正在生成论文摘要