Evaluating the Impact of Adversarial Factors on Membership Inference Attacks
2023 IEEE Smart World Congress (SWC)(2023)
摘要
Existing works have demonstrated that machine learning models may leak sensitive information of the training set to adversaries who launch the membership inference attacks (MIAs). Most of the existing studies on MIAs focus on improving the accuracy of attacks and relaxing assumptions about adversaries, and lack systematical evaluation on the impact of adversarial factors on MIAs. In this paper, we implement several recent attacks and compare their performance to study the importance of seven typical factors, including four background knowledge and three attack parameters. Specifically, we classify these factors according to the target model, the shadow model and the attack model. In our experiments, we use five datasets, CIFAR-100, CIFAR-10, MNIST, Purchase and Location to evaluate the performance of different MIAs with varied factors. Results indicate that factors about the shadow model structure, training data distribution and the target model output exert the dominant impact on the attack performance. We further explore and interpret how these dominant factors affect the success rate of MIAs through experimental analysis.
更多查看译文
关键词
membership inference,privacy evaluation,deep learning
AI 理解论文
溯源树
样例
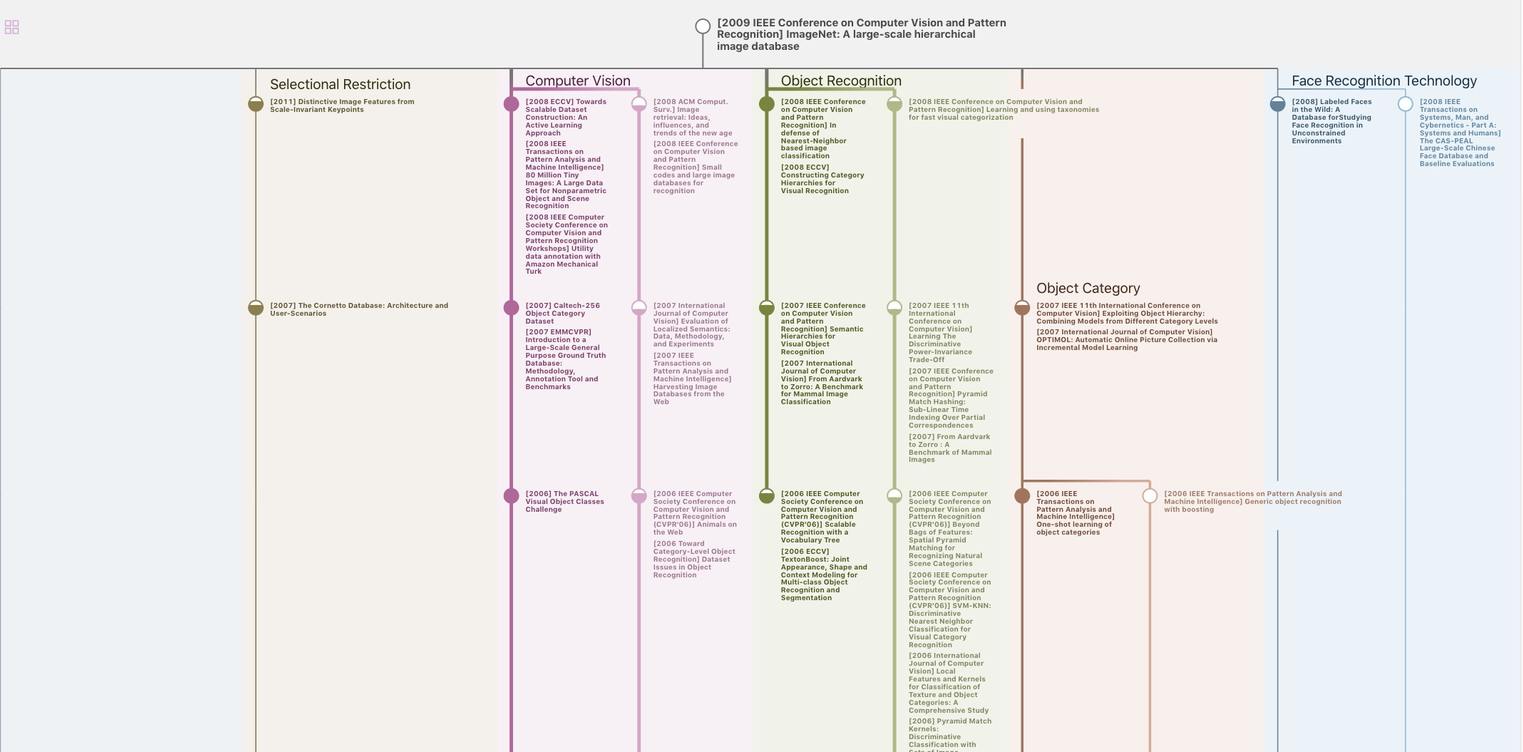
生成溯源树,研究论文发展脉络
Chat Paper
正在生成论文摘要