Learning Instance Geometric Graph for Monocular 3D Object Detection
2023 IEEE Smart World Congress (SWC)(2023)
摘要
Obtaining and handling geometric information is vital to monocular 3D object detection. The performance of current monocular 3D object detection models is largely limited by inaccurate depth estimation of object centers. Most existing methods improve object depth estimation accuracy either by introducing additional geometric information, or by novel network design. However the geometric correlation among objects is often omitted. In this work, we analyze the reason of inaccurate object depth estimation, and propose an instance geometric graph (IGG) based on self-attention mechanism. Specifically, we extract deep geometric features of instances, and construct a fully connected graph based on instance features. The edge of the graph encodes geometric correlations among instances and the instance features are updated by propagating geometric information for improved performance. Our IGG is independent of any geometric constrains and can be implemented within any monocular 3D object detection models. Experiment results on benchmark dataset show that our IGG outperforms state-of-the-art methods.
更多查看译文
关键词
3D Object Detection,graph learning,geometric feature learning
AI 理解论文
溯源树
样例
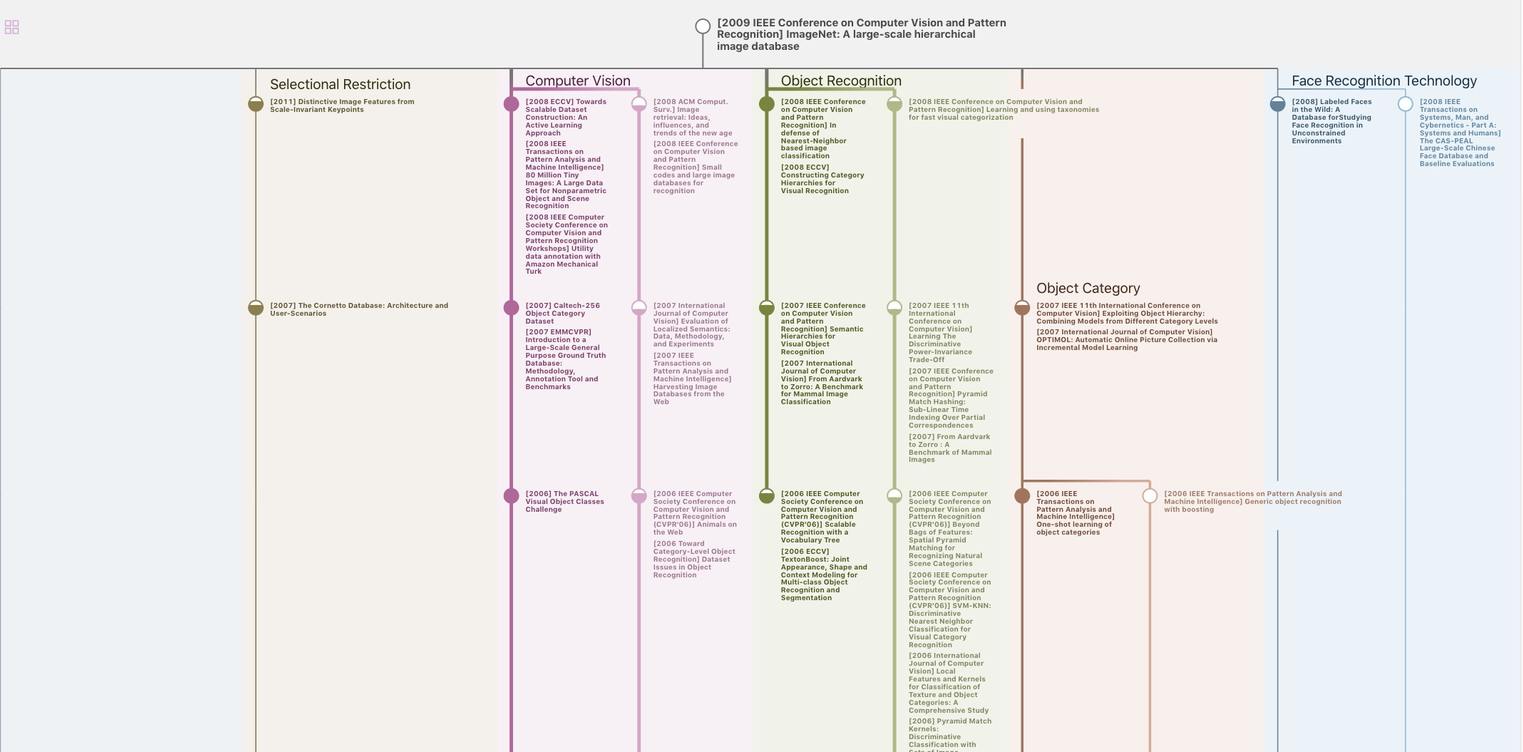
生成溯源树,研究论文发展脉络
Chat Paper
正在生成论文摘要