Exploring COVID-related relationship extraction: Contrasting data sources and analyzing misinformation
HELIYON(2024)
摘要
The COVID-19 pandemic presented an unparalleled challenge to global healthcare systems. A central issue revolves around the urgent need to swiftly amass critical biological and medical knowledge concerning the disease, its treatment, and containment. Remarkably, text data remains an underutilized resource in this context. In this paper, we delve into the extraction of COVID-related relations using transformer-based language models, including Bidirectional Encoder Representations from Transformers (BERT) and DistilBERT. Our analysis scrutinizes the performance of five language models, comparing information from both PubMed and Reddit, and assessing their ability to make novel predictions, including the detection of "misinformation." Key findings reveal that, despite inherent differences, both PubMed and Reddit data contain remarkably similar information, suggesting that Reddit can serve as a valuable resource for rapidly acquiring information during times of crisis. Furthermore, our results demonstrate that language models can unveil previously unseen entities and relations, a crucial aspect in identifying instances of misinformation.
更多查看译文
关键词
Relation extraction,Deep learning,Natural language processing,Data science,Artificial intelligence,Misinformation,Public health
AI 理解论文
溯源树
样例
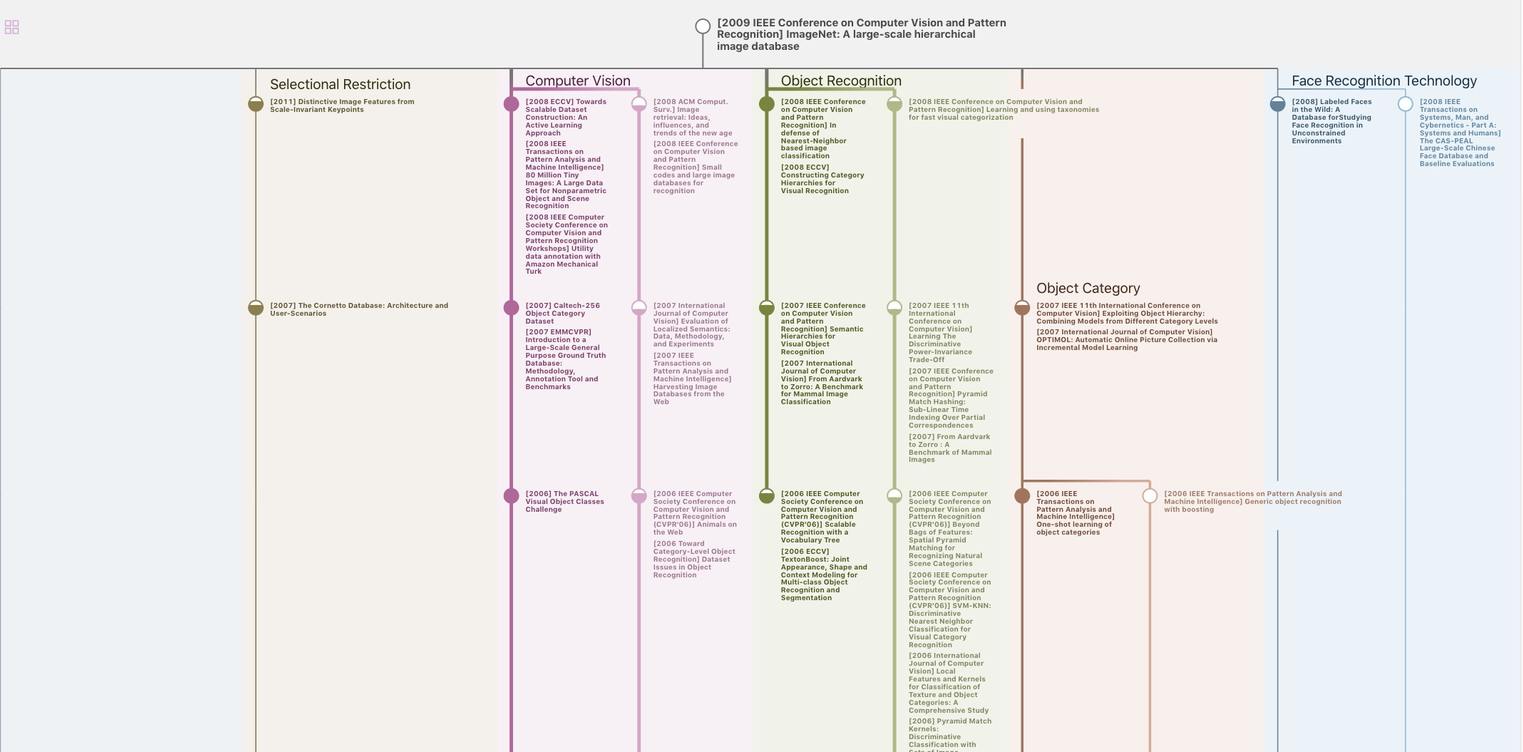
生成溯源树,研究论文发展脉络
Chat Paper
正在生成论文摘要