MC-SQ and MC-MQ: Ensembles for Multi-class Quantification
IEEE Transactions on Knowledge and Data Engineering(2024)
摘要
Quantification research proposes methods to estimate the class distribution in an independent sample. Quantification methods find applications in areas that rely on estimated aggregated quantities, such as epidemiology, sentiment analysis, political research, and ecological surveillance. For instance, epidemiologists are often concerned with the dynamics of the number of disease cases across space and time. Thus, while classification predicts individual subjects, quantifiers are the methods that directly estimate the number of cases. Although quantification is a thriving area of research, with numerous approaches proposed in the last decade, most focus has been on binary-class quantifiers. One common approach for multi-class quantification is the one-versus-all (OVA) approach, but empirical evidence suggests its performance is suboptimal. This paper's first contribution is to elucidate why OVA quantifiers struggle to perform well in multi-class settings due to a distribution shift. To circumvent this problem, our second proposal is two new multi-class quantifiers based on ensemble learning that significantly improve performance for binary and multi-class settings. Our comprehensive experimental setup with 37 state-of-the-art (single and ensemble) quantifiers shows that our ensembles are the best-performing quantifiers and rank first in a recent quantification competition.
更多查看译文
关键词
Quantification,prevalence estimation,class probability estimation,ensembles,multi-class,machine learning
AI 理解论文
溯源树
样例
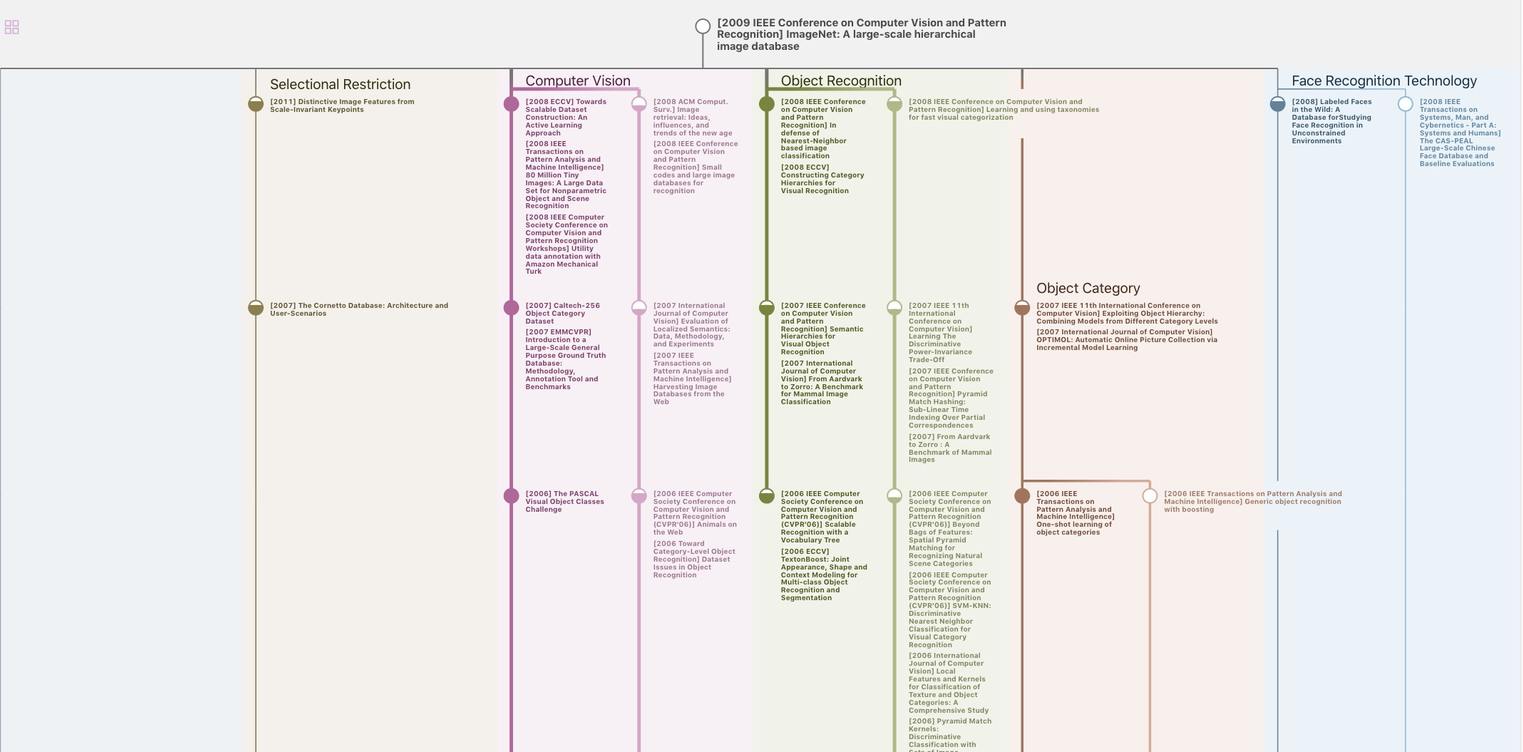
生成溯源树,研究论文发展脉络
Chat Paper
正在生成论文摘要