Learning Multi-Frequency Integration Network for RGBT Tracking
IEEE Sensors Journal(2024)
摘要
RGBT tracking is an attractive topic that benefits from the complementarity of visible and thermal sensors to better handle tracking tasks in atrocious scenarios. Existing RGBT trackers typically introduce self-attention to capture long-range dependencies. However, recent findings suggest that self-attention is a low-pass filter, meaning that high-frequency clues involving local edges and texture may be repressed. Aim at the problem, this paper comprehensively considers the multi-frequency knowledge of heterogeneous modalities and proposes a Learning Multi-frequency Integration Network for RGBT tracking to effectively implement adaptive extraction, enhancement and integration of multi-frequency cues. The proposed LMINet primarily benefits from the deployment of three crucial components: Pattern-aware Reinforcement (PR), Multi-frequency Enhancement (ME) and Multi-frequency Integration (MI). Specifically, the PR part consists of carefully designed Reinforcement Unit and Learnable Weighting Strategy 1 (LWS
1
). The former extracts information from the data flow to enhance the backbone, while the latter is a data-driven regulation mechanism that adaptively adjusts the enhancement intensity via learning the input. Then, the ME component separates high- and low-frequency knowledge via High-level Branch (HB) and Common Unit, and further adjusts the improvement intensity of multi-frequency cues via the learning of LWS
2
to achieve intra-modal refinement. Moreover, the MI part first extracts high and low frequency signals via HB and Low-level Branch, and implements cross-modal integration of high and low frequency cues through LWS
3
respectively. Extensive experimental results on GTOT, RGBT234 and LasHeR demonstrate that the proposed LMINet is effective and competitive with state-of-the-art algorithms. The code will be open sourced at https://github.com/mjt1312/Lminet.
更多查看译文
关键词
RGBT tracking,multi-frequency integration,modal heterogeneity,intra-modal,inter-modal
AI 理解论文
溯源树
样例
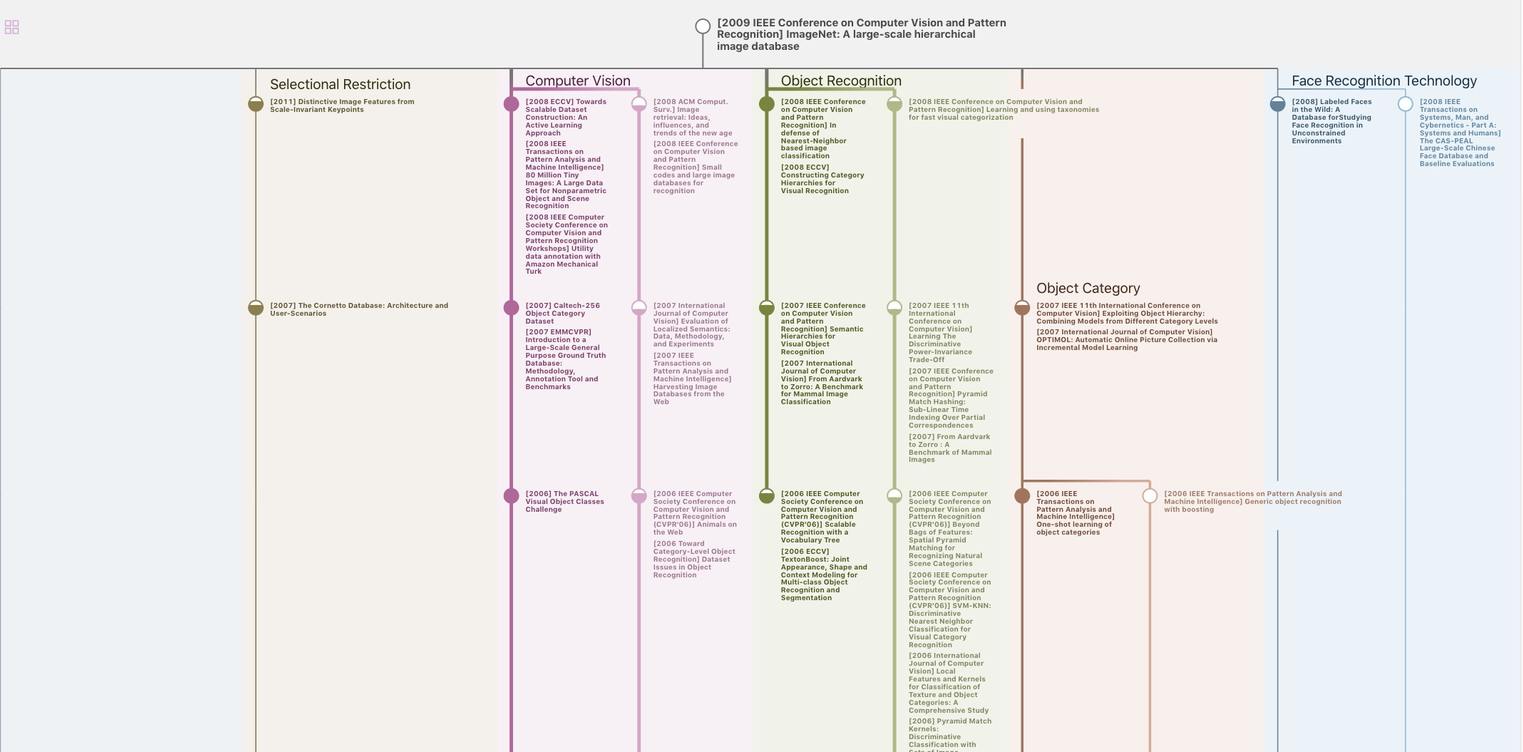
生成溯源树,研究论文发展脉络
Chat Paper
正在生成论文摘要