Multitasking Feedback Optimization Algorithm Based on an Evolutionary State Estimator
IEEE TRANSACTIONS ON EMERGING TOPICS IN COMPUTATIONAL INTELLIGENCE(2024)
摘要
Evolutionary multitasking optimization (EMTO), owing to its advantage of knowledge sharing, is capable of resolving multiple optimization tasks concurrently. Considering the evolutionary progresses between tasks may be inconsistent, it is necessary for EMTO to regulate the knowledge transfer strategy (KTS), which can alleviate the negative transfer caused by unmatched knowledge. Inspired by this, a multitasking feedback optimization algorithm is proposed with an evolutionary state estimator (MTFO-ESE). First, a multi-source knowledge acquisition strategy (MKA) is introduced to achieve inter-task knowledge, which promotes the tasks to seek the optimization directions in the search space. Second, an evolutionary state estimator (ESE) is established to evaluate the search progress of each task toward the optimal solution. The main idea is to measure the evolutionary pressure of the population under the current individual update strategy using prior and posterior observation. Third, a double-feedback adjustment mechanism (DFBA) is developed to manage KTS based on ESE. This mechanism contributes to alleviating the negative effect caused by unmatched knowledge and eliminating unnecessary exploration. Moreover, the convergence of the proposed MTFO-ESE is analyzed to ensure its effectiveness. Finally, the superior convergence and positive transfer ability of the proposed algorithm are verified through comparative experiments, ablation analyses, and a practical application.
更多查看译文
关键词
Task analysis,Optimization,Statistics,Sociology,Multitasking,Knowledge transfer,Search problems,Evolutionary multitasking optimization,evolutionary state estimator,knowledge transfer,negative transfer
AI 理解论文
溯源树
样例
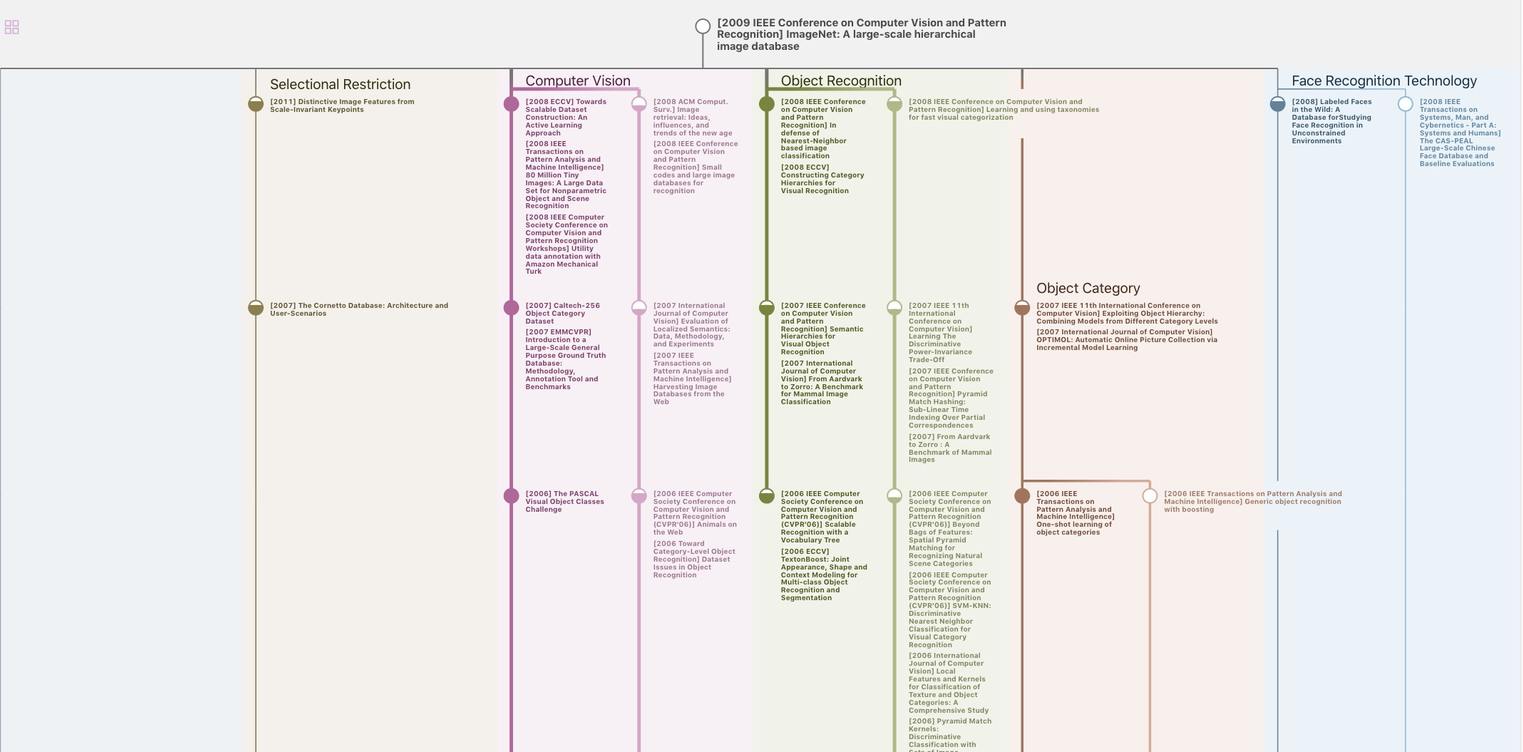
生成溯源树,研究论文发展脉络
Chat Paper
正在生成论文摘要