ACE-CNN: Approximate Carry Disregard Multipliers for Energy-Efficient CNN-Based Image Classification
IEEE TRANSACTIONS ON CIRCUITS AND SYSTEMS I-REGULAR PAPERS(2024)
摘要
This paper presents the design and development of Signed Carry Disregard Multiplier (SCDM8), a family of signed approximate multipliers tailored for integration into Convolutional Neural Networks (CNNs). Extensive experiments were conducted on popular pre-trained CNN models, including VGG16, VGG19, ResNet101, ResNet152, MobileNetV2, InceptionV3, and ConvNeXt-T to evaluate the trade-off between accuracy and approximation. The results demonstrate that ACE-CNN outperforms other configurations, offering a favorable balance between accuracy and computational efficiency. In our experiments, when applied to VGG16, SCDM8 achieves an average reduction in power consumption of 35% with a marginal decrease in accuracy of only 1.5%. Similarly, when incorporated into ResNet152, SCDM8 yields an energy saving of 42% while sacrificing only 1.8% in accuracy. ACE-CNN provides the first approximate version of ConvNeXt which yields up to 72% energy improvement at the price of less than only 1.3% Top-1 accuracy. These results highlight the suitability of SCDM8 as an approximation method across various CNN models. Our analysis shows that the ACE-CNN outperforms state-of-the-art approaches in accuracy, energy efficiency, and computation precision for image classification tasks in CNNs. Our study investigated the resiliency of CNN models to approximate multipliers, revealing that ResNet101 demonstrated the highest resiliency with an average difference in the accuracy of 0.97%, whereas LeNet5 Inspired-CNN exhibited the lowest resiliency with an average difference of 2.92%. These findings aid in selecting energy-efficient approximate multipliers for CNN-based systems, and contribute to the development of energy-efficient deep learning systems by offering an effective approximation technique for multipliers in CNNs. The proposed SCDM8 family of approximate multipliers opens new avenues for efficient deep learning applications, enabling significant energy savings with virtually no loss in accuracy.
更多查看译文
关键词
Hardware,Convolutional neural networks,Image classification,Task analysis,Energy efficiency,Delays,Computer architecture,convolutional neural network,approximate multiplier,image classification
AI 理解论文
溯源树
样例
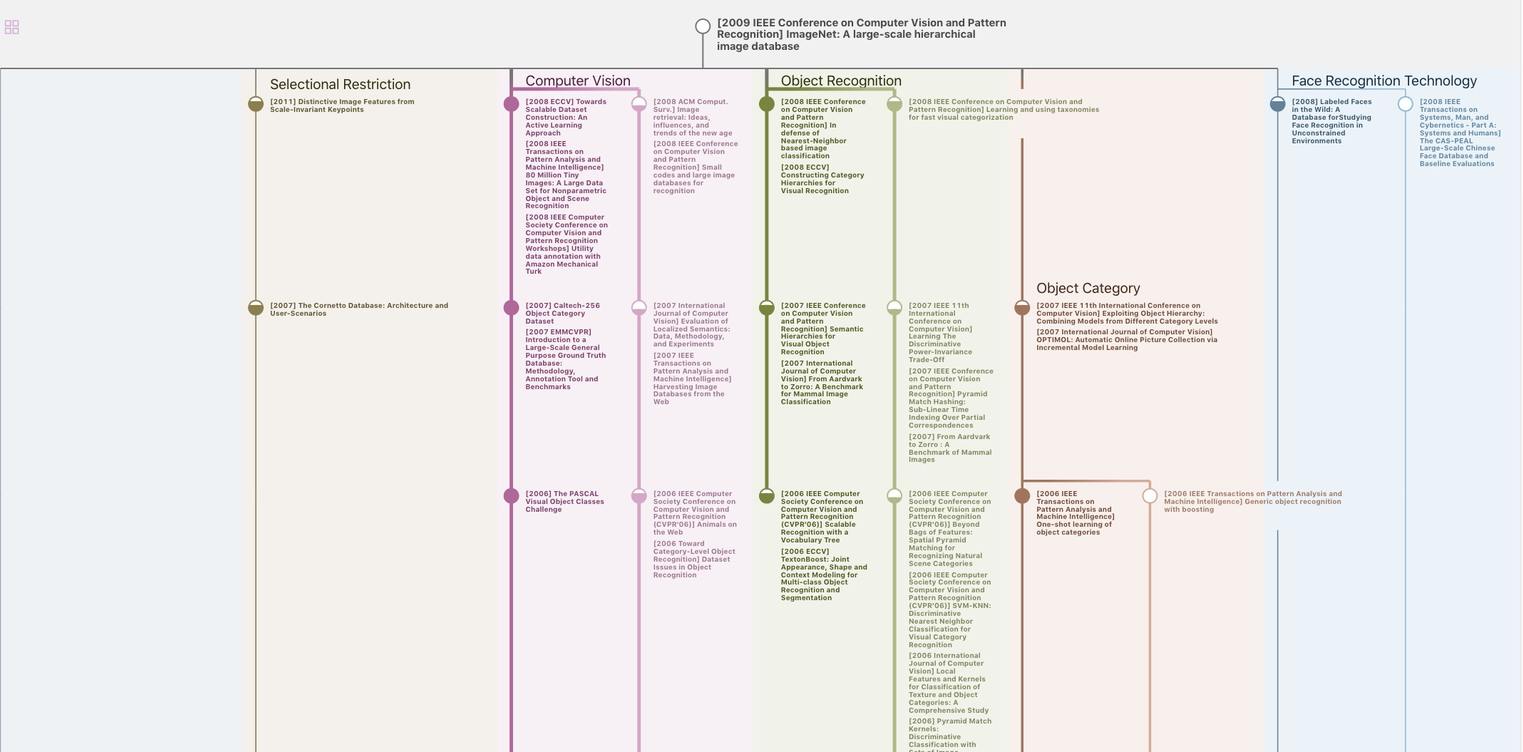
生成溯源树,研究论文发展脉络
Chat Paper
正在生成论文摘要