Efficient Multi-Object Recognition Using GMM Segmentation Feature Fusion Approach
IEEE ACCESS(2024)
摘要
Machines need to be able to recognize and understand complex visual surroundings to function at their best in a variety of contexts. Here, we address the difficult problem of multi-object recognition to obtain a sophisticated knowledge of complex visual environments, tackling issues such as size, occlusion, fluctuations in object traits, and complicated backdrops. Our contribution is to provide novel methods (Gaussian mixture model and mean-shift algorithms) for inferring multiple object segmentation in complicated visuals, introducing a unique multiclass object classification strategy utilizing benchmark datasets. Notably, by utilizing local appearance, texture, and geometry characteristics, our technique considerably improves classification accuracy by integrating a Multi-Layer Perceptron (MLP) with area signatures and local descriptors. By facilitating accurate object matching and identification based on local appearance, texture, and geometric features, local descriptors are essential for collecting particular information and regions of interest in images. When compared to state-of-the-art methods, empirical validation on MSRC and Corel 10k datasets shows better performance, especially when managing object occlusion problems. With an accuracy of 90.6% and 89.69%, respectively, our suggested system performs better than industry standards for multi-object classification on both datasets, highlighting the significant progress our method makes to the area of multiclass object classification in challenging visual contexts.
更多查看译文
关键词
Image segmentation,Object recognition,Feature extraction,Visualization,Statistics,Gaussian mixture model,Task analysis,Saliency detection,mean-shift segmentation,feature fusion,saliency map,multi-object categorization,local descriptors
AI 理解论文
溯源树
样例
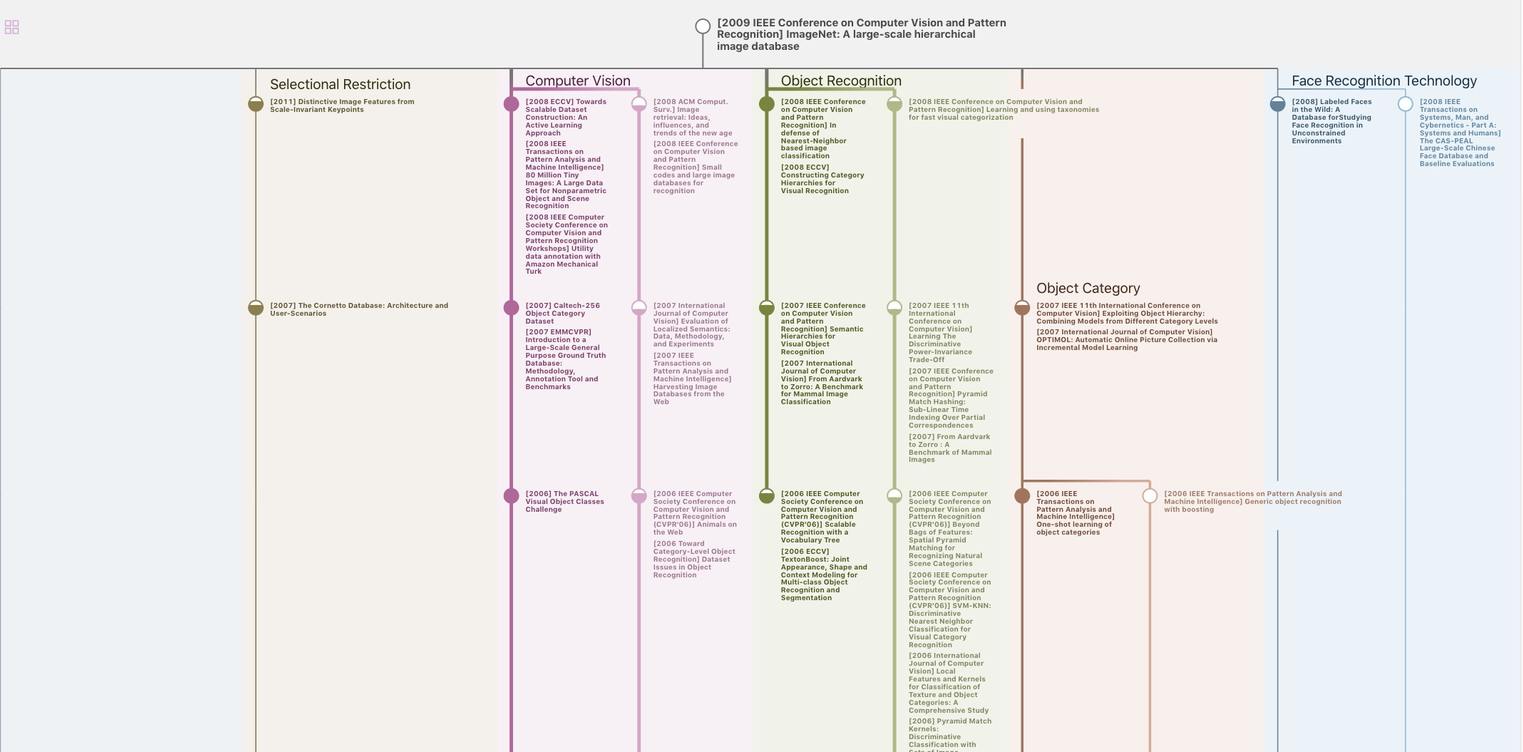
生成溯源树,研究论文发展脉络
Chat Paper
正在生成论文摘要