Epilepsy EEG signals classification based on sparse principal component logistic regression model
COMPUTER METHODS IN BIOMECHANICS AND BIOMEDICAL ENGINEERING(2024)
摘要
Epilepsy is a chronic brain disease caused by excessive discharge of brain neurons. Long-term recurrent seizures bring a lot of trouble to patients and their families. Prediction of different stages of epilepsy is of great significance. We extract pearson correlation coefficients (PCC) between channels in different frequency bands as features of EEG signals for epilepsy stages prediction. However, the features are of large feature dimension and serious multi-collinearity. To eliminate these adverse influence, the combination of traditional dimension reduction method principal component analysis (PCA) and logistic regression method with regularization term is proposed to avoid over-fitting and achieve the feature sparsity. The experiments are conducted on the widely used CHB-MIT dataset using different regularization terms L1 and L2, respectively. The proposed method identifies various stages of epilepsy quickly and efficiently, and it presents the best average accuracy of 94.86%, average precision of 96.71%, average recall of 93.48%, average kappa value of 0.90 and average Matthews correlation coefficient (MCC) value of 0.90 for all patients.
更多查看译文
关键词
EEG,pearson correlation coefficient,principal component analysis,logistic regression with regularization term,classification
AI 理解论文
溯源树
样例
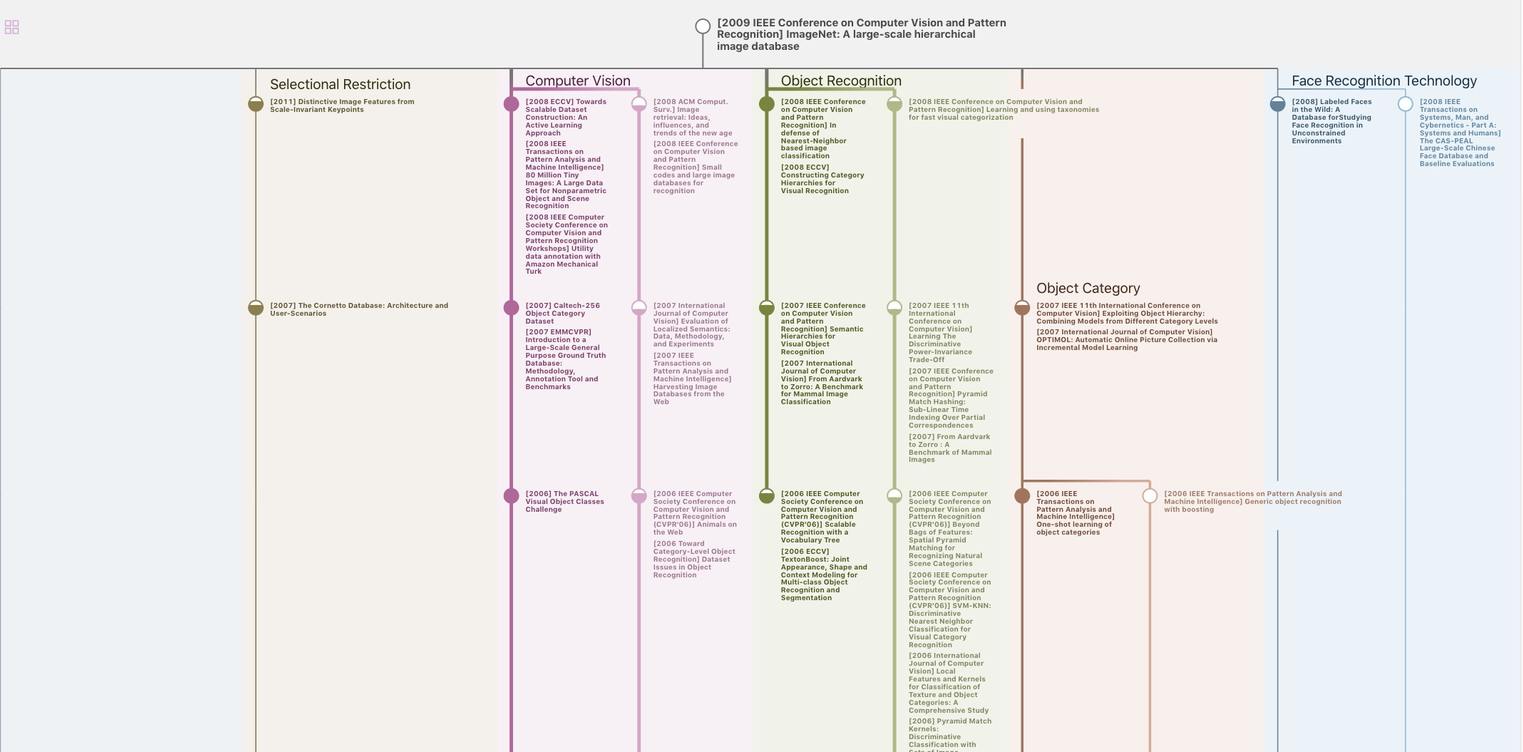
生成溯源树,研究论文发展脉络
Chat Paper
正在生成论文摘要