Machine Learning-Assisted Discovery of Propane-Selective Metal-Organic Frameworks
JOURNAL OF THE AMERICAN CHEMICAL SOCIETY(2024)
摘要
Machine learning is gaining momentum in the prediction and discovery of materials for specific applications. Given the abundance of metal-organic frameworks (MOFs), computational screening of the existing MOFs for propane/propylene (C3H8/C3H6) separation could be equally important for developing new MOFs. Herein, we report a machine learning-assisted strategy for screening C3H8-selective MOFs from the CoRE MOF database. Among the four algorithms applied in machine learning, the random forest (RF) algorithm displays the highest degree of accuracy. We experimentally verified the identified top-performing MOF (JNU-90) with its benchmark selectivity and separation performance of directly producing C3H6. Considering its excellent hydrolytic stability, JNU-90 shows great promise in the energy-efficient separation of C3H8/C3H6. This work may accelerate the development of MOFs for challenging separations.
更多查看译文
AI 理解论文
溯源树
样例
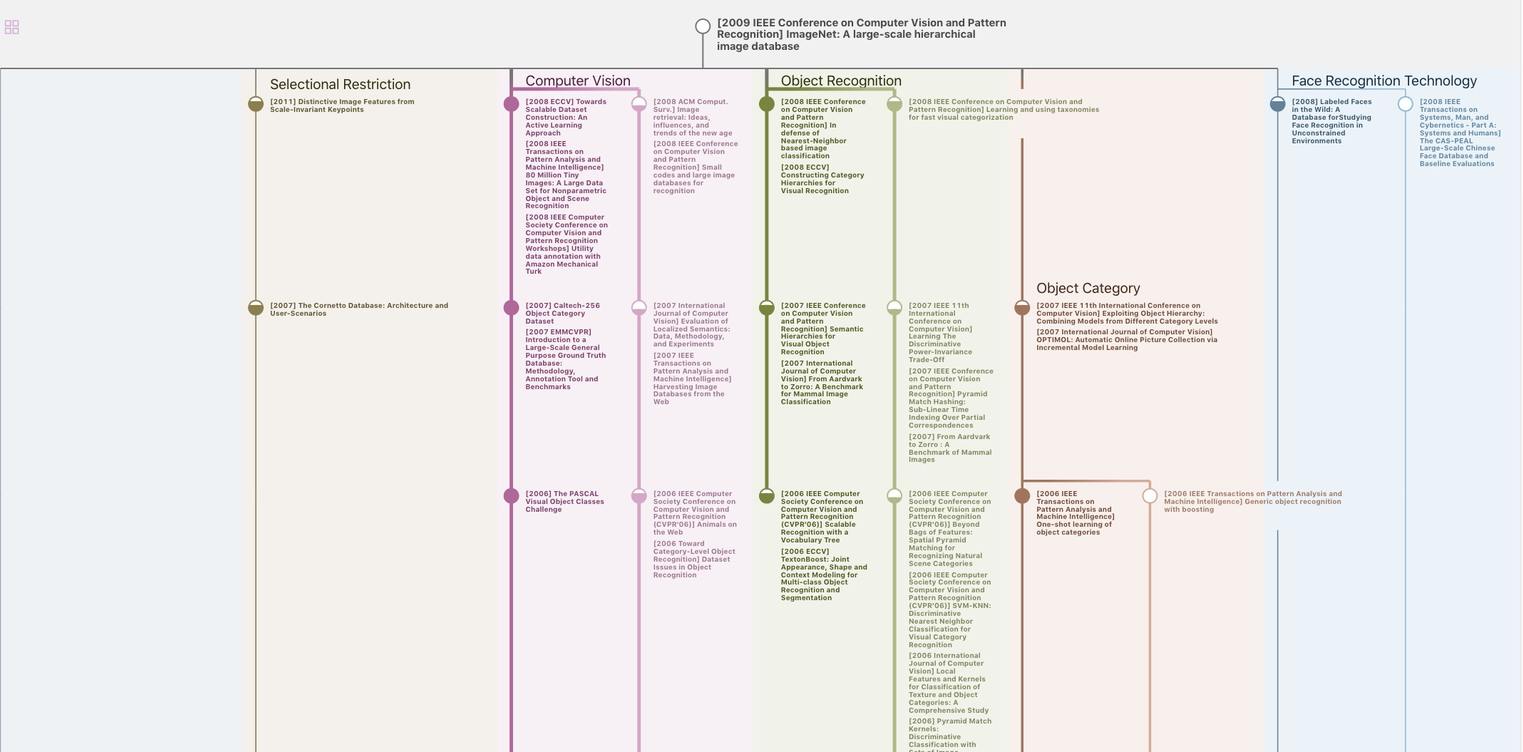
生成溯源树,研究论文发展脉络
Chat Paper
正在生成论文摘要