LFC-UNet: learned lossless medical image fast compression with U-Net
PEERJ COMPUTER SCIENCE(2024)
摘要
In the field of medicine, the rapid advancement of medical technology has significantly increased the speed of medical image generation, compelling us to seek efficient methods for image compression. Neural networks, owing to their outstanding image estimation capabilities, have provided new avenues for lossless compression. In recent years, learning -based lossless image compression methods, combining neural network predictions with residuals, have achieved performance comparable to traditional nonlearning algorithms. However, existing methods have not taken into account that residuals often concentrate excessively, hindering the neural network's ability to learn accurate residual probability estimation. To address this issue, this study employs a weighted cross -entropy method to handle the imbalance in residual categories. In terms of network architecture, we introduce skip connections from U -Net to better capture image features, thereby obtaining accurate probability estimates. Furthermore, our framework boasts excellent encoding speed, as the model is able to acquire all residuals and residual probabilities in a single inference pass. The experimental results demonstrate that the proposed method achieves state-of-the-art performance on medical datasets while also offering the fastest processing speed. As illustrated by an instance using head CT data, our approach achieves a compression efficiency of 2.30 bits per pixel, with a processing time of only 0.320 seconds per image.
更多查看译文
关键词
Lossless compression,Medical image,Neural network
AI 理解论文
溯源树
样例
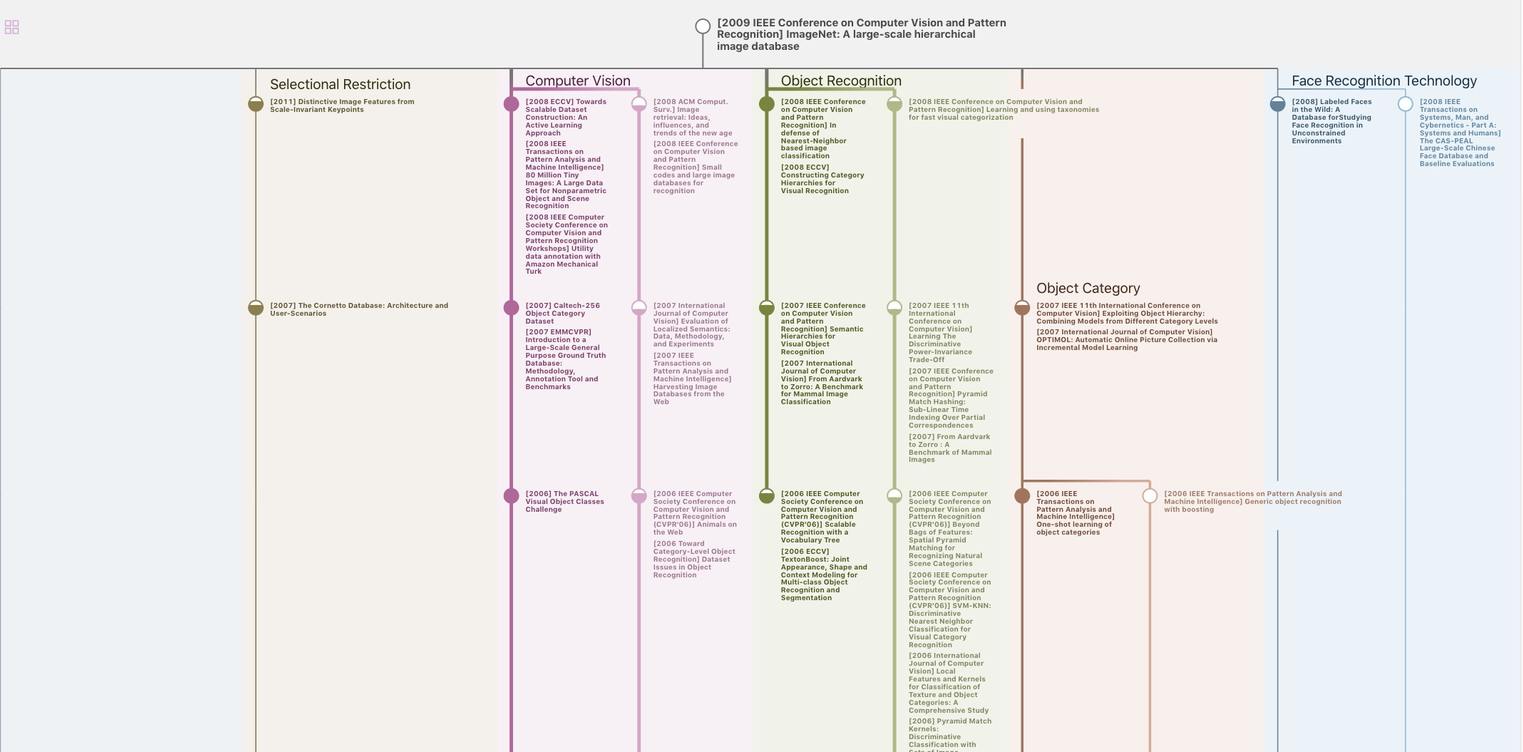
生成溯源树,研究论文发展脉络
Chat Paper
正在生成论文摘要