Guest Editorial Special Issue on Reinforcement Learning-Based Control: Data-Efficient and Resilient Methods
IEEE TRANSACTIONS ON NEURAL NETWORKS AND LEARNING SYSTEMS(2024)
摘要
As an important branch of machine learning, reinforcement learning (RL) has proved its efficiency in many emerging applications in science and engineering. A remarkable advantage of RL is that it enables agents to maximize their cumulative rewards through online exploration and interactions with unknown (or partially unknown) and uncertain environments, which is regarded as a variant of data-driven adaptive optimal control methods. However, the successful implementation of RL-based control systems usually relies on a good quantity of online data due to its data-driven nature. Therefore, it is imperative to develop data-efficient RL methods for control systems to reduce the required number of interactions with the external environment. Moreover, network-aware issues, such as cyberattacks, dropout packet and communication latency, and actuator and sensor faults, are challenging conundrums that threaten the safety, security, stability, and reliability of network control systems. Consequently, it is significant to develop safe and resilient RL mechanisms.
更多查看译文
关键词
Special issues and sections,Reinforcement learning,Learning systems,Data integrity,Data models,Computer network management,Resilience
AI 理解论文
溯源树
样例
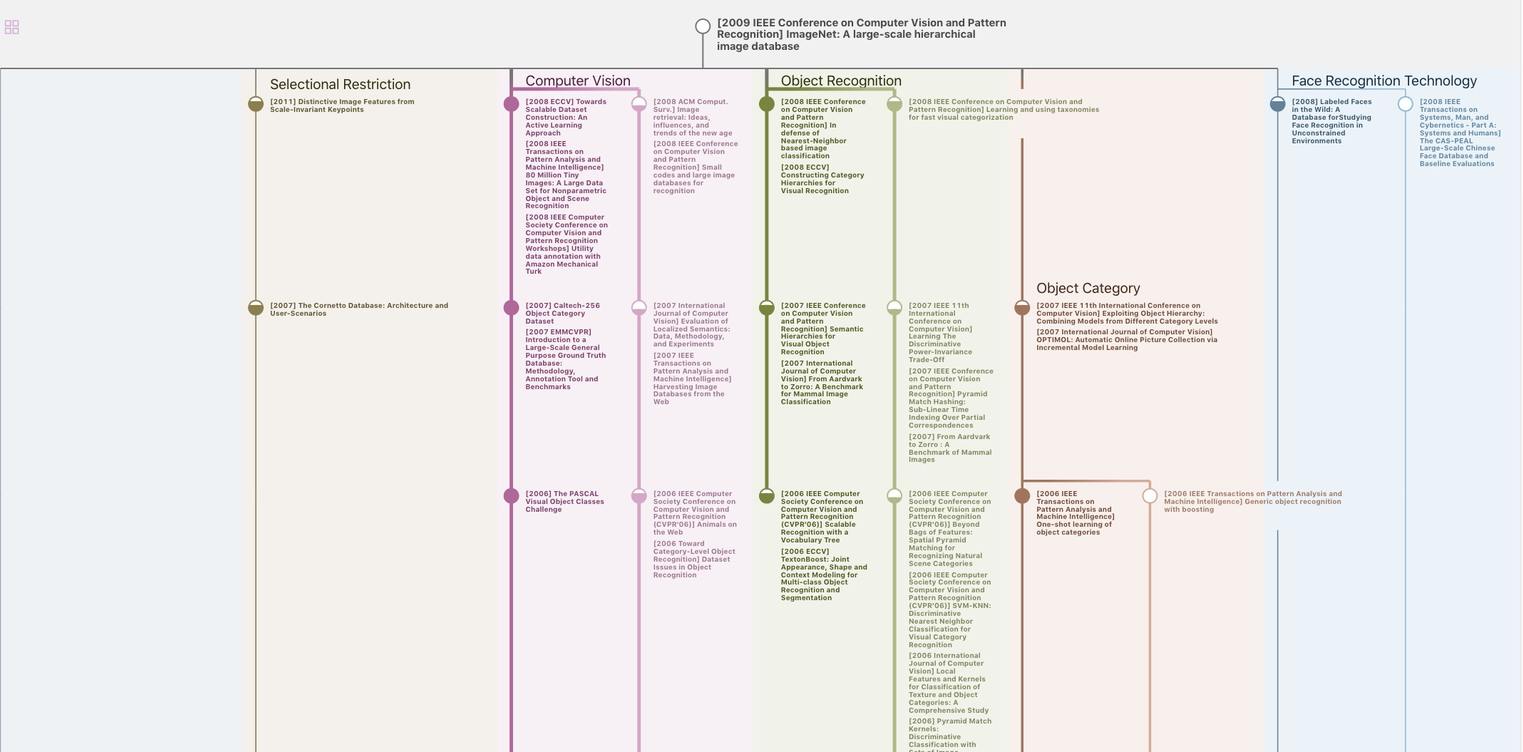
生成溯源树,研究论文发展脉络
Chat Paper
正在生成论文摘要