Differentially Private Worst-group Risk Minimization
CoRR(2024)
摘要
We initiate a systematic study of worst-group risk minimization under
(ϵ, δ)-differential privacy (DP). The goal is to privately find a
model that approximately minimizes the maximal risk across p sub-populations
(groups) with different distributions, where each group distribution is
accessed via a sample oracle. We first present a new algorithm that achieves
excess worst-group population risk of Õ(p√(d)/Kϵ +
√(p/K)), where K is the total number of samples drawn from all
groups and d is the problem dimension. Our rate is nearly optimal when each
distribution is observed via a fixed-size dataset of size K/p. Our result is
based on a new stability-based analysis for the generalization error. In
particular, we show that Δ-uniform argument stability implies
Õ(Δ + 1/√(n)) generalization error w.r.t. the
worst-group risk, where n is the number of samples drawn from each sample
oracle. Next, we propose an algorithmic framework for worst-group population
risk minimization using any DP online convex optimization algorithm as a
subroutine. Hence, we give another excess risk bound of Õ(
√(d^1/2/ϵ K) +√(p/Kϵ^2)).
Assuming the typical setting of ϵ=Θ(1), this bound is more
favorable than our first bound in a certain range of p as a function of K
and d. Finally, we study differentially private worst-group empirical risk
minimization in the offline setting, where each group distribution is observed
by a fixed-size dataset. We present a new algorithm with nearly optimal excess
risk of Õ(p√(d)/Kϵ).
更多查看译文
AI 理解论文
溯源树
样例
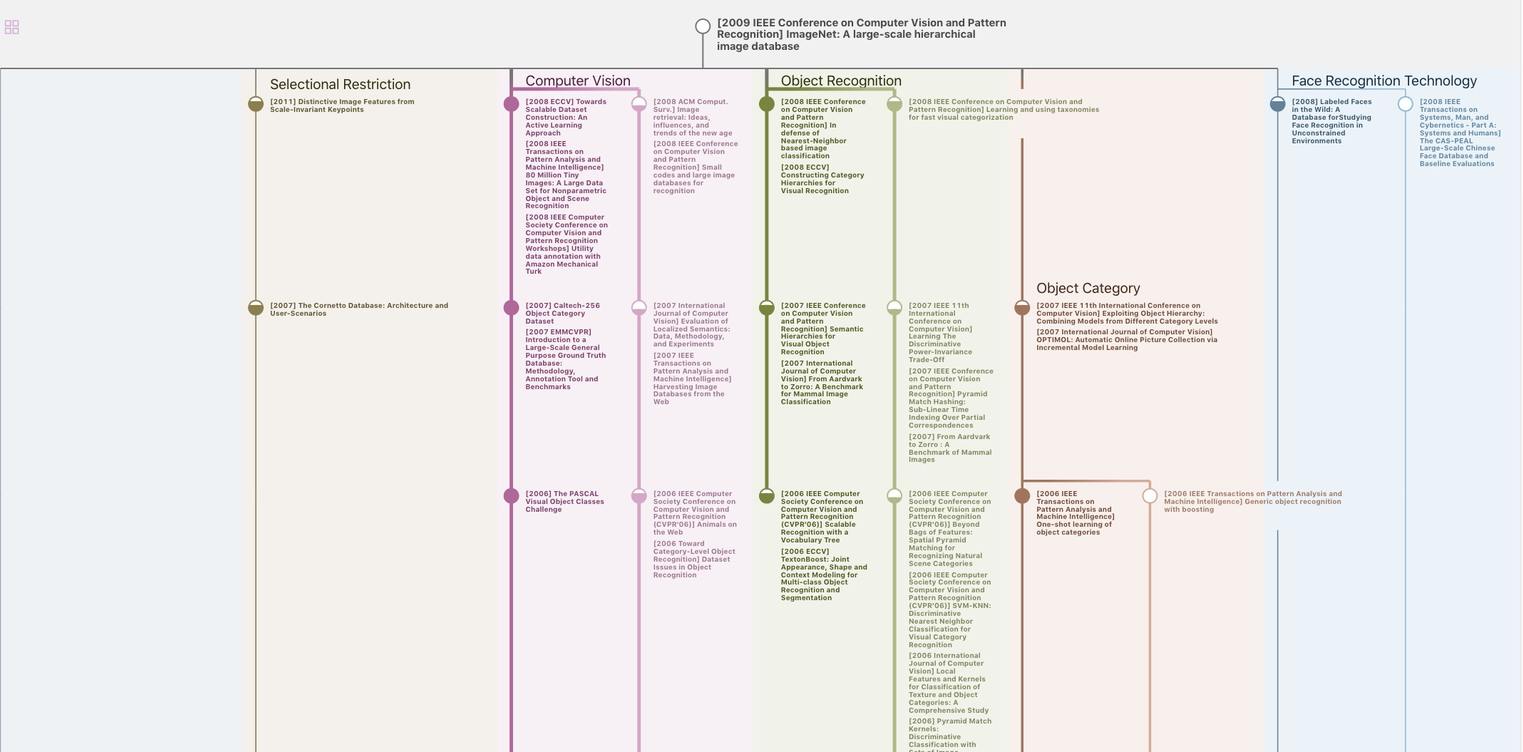
生成溯源树,研究论文发展脉络
Chat Paper
正在生成论文摘要