Safeguarding Privacy and Integrity of Federated Learning in Heterogeneous Cross-Silo IoRT Environments: A Moving Target Defense Approach
IEEE Network(2024)
摘要
In virtue of the Internet of Things and Cobots, the Internet of Robotic Things (IoRT) significantly accelerates production efficiency and quality. As the scope and complexity of IoRT continue to expand, federated learning among massive robots is in urgent need. Nonetheless, this growing demand is accompanied by heightened threats to data privacy and model integrity. Besides, the heterogeneity among cross-silo robots compounds these challenges. In this paper, we discuss the key concerns of collaborative training in IoRT, and propose a shuffling-based moving target defense approach for federated learning in heterogeneous cross-silo IoRT environments (FedMTD). Based on the hierarchical training structure with node clustering, FedMTD bounds heterogeneity by domains, thereby minimizing the learning error and privacy loss. It also enhances resistance towards poisoning adversaries through decentralized credit evaluation. Finally, experimental results illustrate that FedMTD jointly demonstrates significant improvements in learning performance, privacy enhancement, and poisoning resistance.
更多查看译文
关键词
Internet of robotic things,Federated learning,Privacy,Moving target defense
AI 理解论文
溯源树
样例
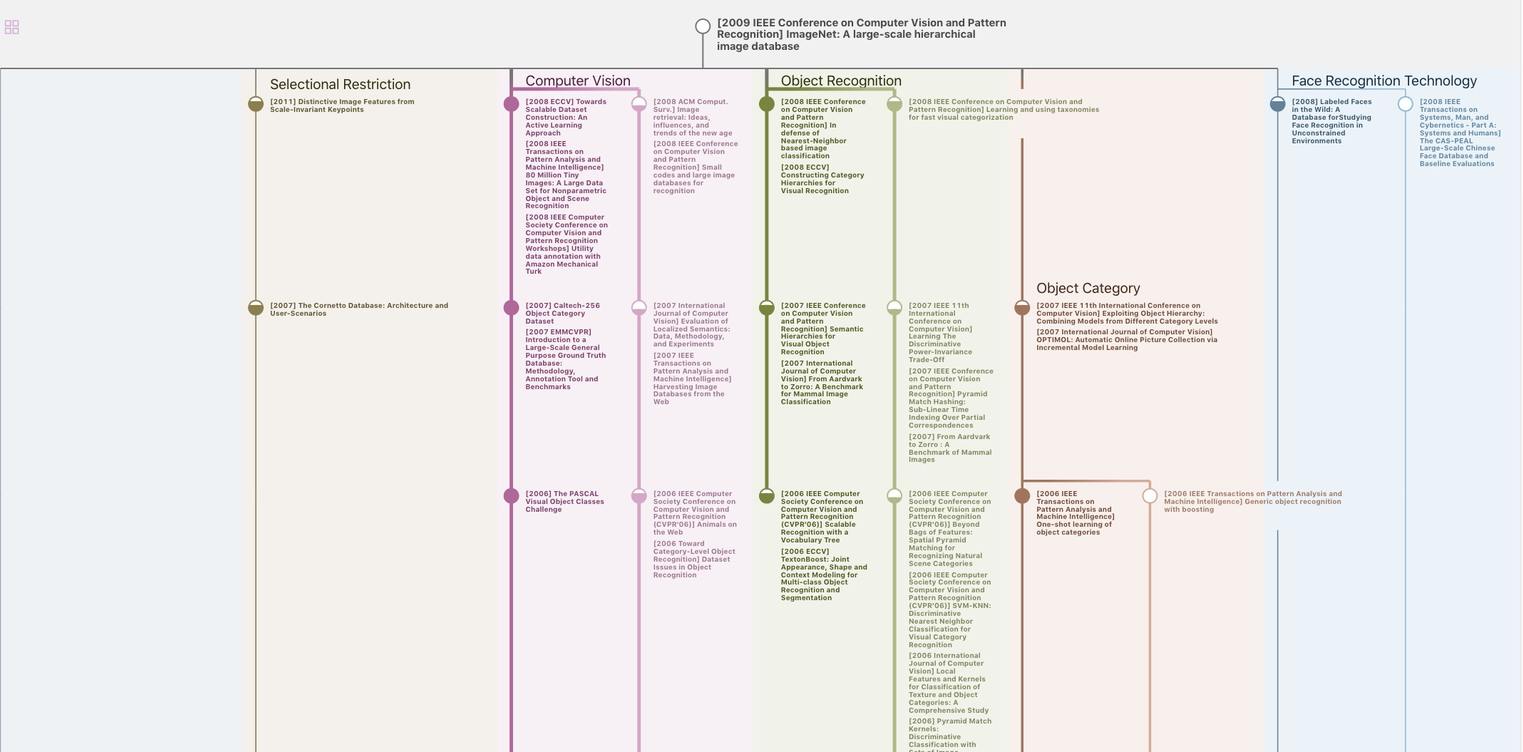
生成溯源树,研究论文发展脉络
Chat Paper
正在生成论文摘要