Deep Network for Image Compressed Sensing Coding Using Local Structural Sampling
ACM Transactions on Multimedia Computing, Communications, and Applications(2024)
摘要
Existing image compressed sensing (CS) coding frameworks usually solve an
inverse problem based on measurement coding and optimization-based image
reconstruction, which still exist the following two challenges: 1) The widely
used random sampling matrix, such as the Gaussian Random Matrix (GRM), usually
leads to low measurement coding efficiency. 2) The optimization-based
reconstruction methods generally maintain a much higher computational
complexity. In this paper, we propose a new CNN based image CS coding framework
using local structural sampling (dubbed CSCNet) that includes three functional
modules: local structural sampling, measurement coding and Laplacian pyramid
reconstruction. In the proposed framework, instead of GRM, a new local
structural sampling matrix is first developed, which is able to enhance the
correlation between the measurements through a local perceptual sampling
strategy. Besides, the designed local structural sampling matrix can be jointly
optimized with the other functional modules during training process. After
sampling, the measurements with high correlations are produced, which are then
coded into final bitstreams by the third-party image codec. At last, a
Laplacian pyramid reconstruction network is proposed to efficiently recover the
target image from the measurement domain to the image domain. Extensive
experimental results demonstrate that the proposed scheme outperforms the
existing state-of-the-art CS coding methods, while maintaining fast
computational speed.
更多查看译文
关键词
Compressed sensing (CS),compressed sensing coding,local structural sampling,convolutional neural network (CNN),third-party image codec
AI 理解论文
溯源树
样例
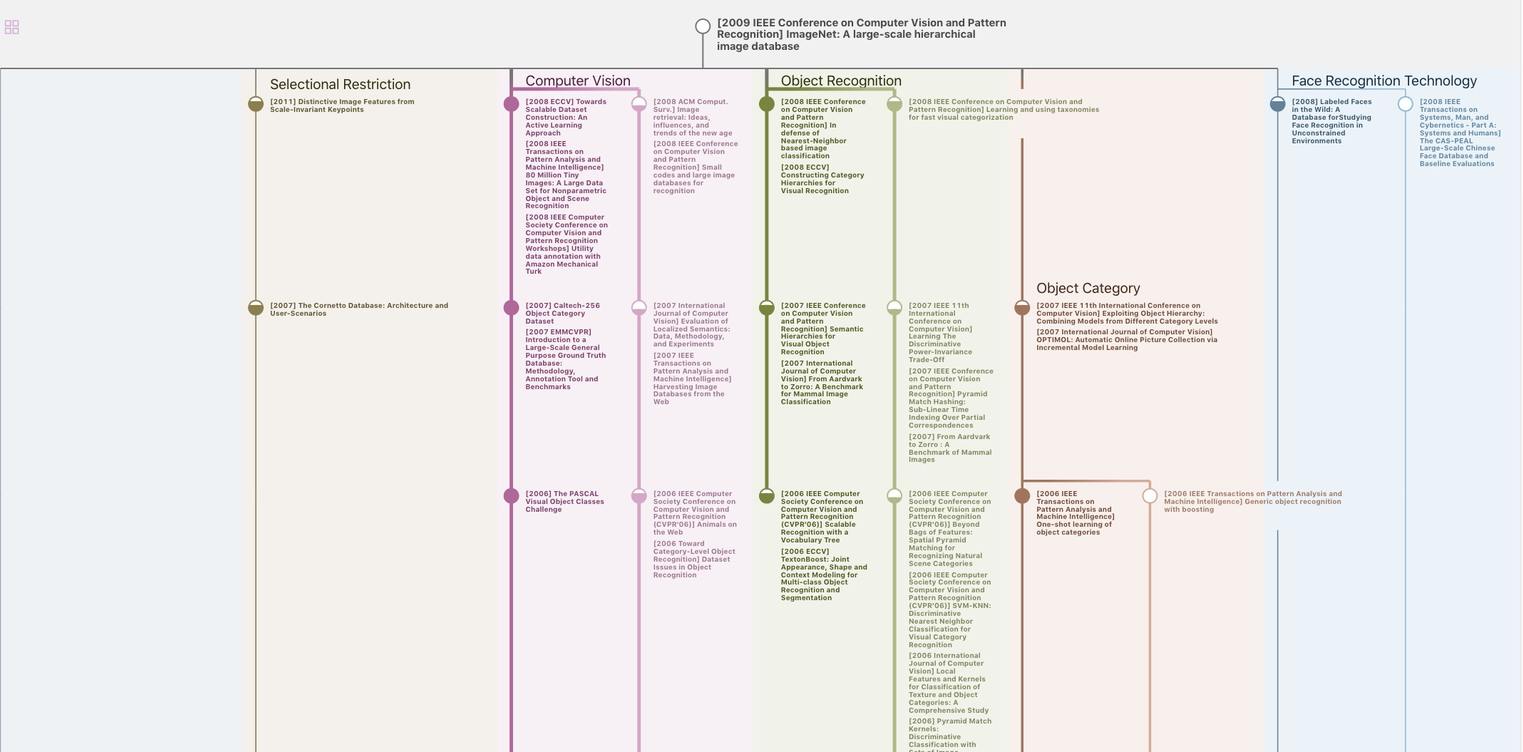
生成溯源树,研究论文发展脉络
Chat Paper
正在生成论文摘要