Progressive Contrastive Learning with Multi-Prototype for Unsupervised Visible-Infrared Person Re-identification
CoRR(2024)
摘要
Unsupervised visible-infrared person re-identification (USVI-ReID) aims to
match specified people in infrared images to visible images without annotation,
and vice versa. USVI-ReID is a challenging yet under-explored task. Most
existing methods address the USVI-ReID problem using cluster-based contrastive
learning, which simply employs the cluster center as a representation of a
person. However, the cluster center primarily focuses on shared information,
overlooking disparity. To address the problem, we propose a Progressive
Contrastive Learning with Multi-Prototype (PCLMP) method for USVI-ReID. In
brief, we first generate the hard prototype by selecting the sample with the
maximum distance from the cluster center. This hard prototype is used in the
contrastive loss to emphasize disparity. Additionally, instead of rigidly
aligning query images to a specific prototype, we generate the dynamic
prototype by randomly picking samples within a cluster. This dynamic prototype
is used to retain the natural variety of features while reducing instability in
the simultaneous learning of both common and disparate information. Finally, we
introduce a progressive learning strategy to gradually shift the model's
attention towards hard samples, avoiding cluster deterioration. Extensive
experiments conducted on the publicly available SYSU-MM01 and RegDB datasets
validate the effectiveness of the proposed method. PCLMP outperforms the
existing state-of-the-art method with an average mAP improvement of 3.9
source codes will be released.
更多查看译文
AI 理解论文
溯源树
样例
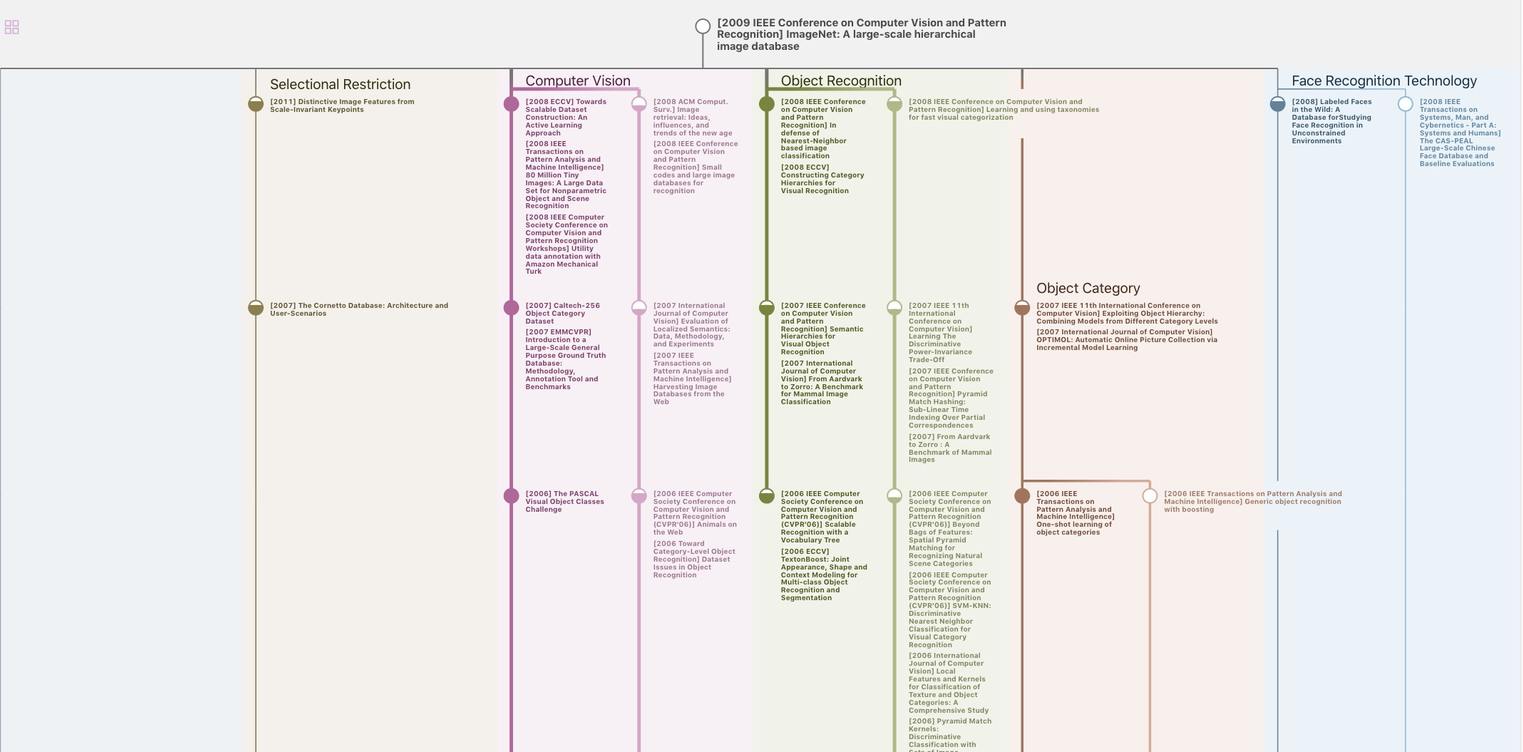
生成溯源树,研究论文发展脉络
Chat Paper
正在生成论文摘要