Conjectural Online Learning with First-order Beliefs in Asymmetric Information Stochastic Games
CoRR(2024)
摘要
Stochastic games arise in many complex socio-technical systems, such as
cyber-physical systems and IT infrastructures, where information asymmetry
presents challenges for decision-making entities (players). Existing
computational methods for asymmetric information stochastic games (AISG) are
primarily offline, targeting special classes of AISGs to avoid belief
hierarchies, and lack online adaptability to deviations from equilibrium. To
address this limitation, we propose a conjectural online learning (COL), a
learning scheme for generic AISGs. COL, structured as a forecaster-actor-critic
(FAC) architecture, utilizes first-order beliefs over the hidden states and
subjective forecasts of the opponent's strategies. Against the conjectured
opponent, COL updates strategies in an actor-critic approach using online
rollout and calibrates conjectures through Bayesian learning. We prove that
conjecture in COL is asymptotically consistent with the information feedback in
the sense of a relaxed Bayesian consistency. The resulting empirical strategy
profile converges to the Berk-Nash equilibrium, a solution concept
characterizing rationality under subjectivity. Experimental results from an
intrusion response use case demonstrate COL's superiority over state-of-the-art
reinforcement learning methods against nonstationary attacks.
更多查看译文
AI 理解论文
溯源树
样例
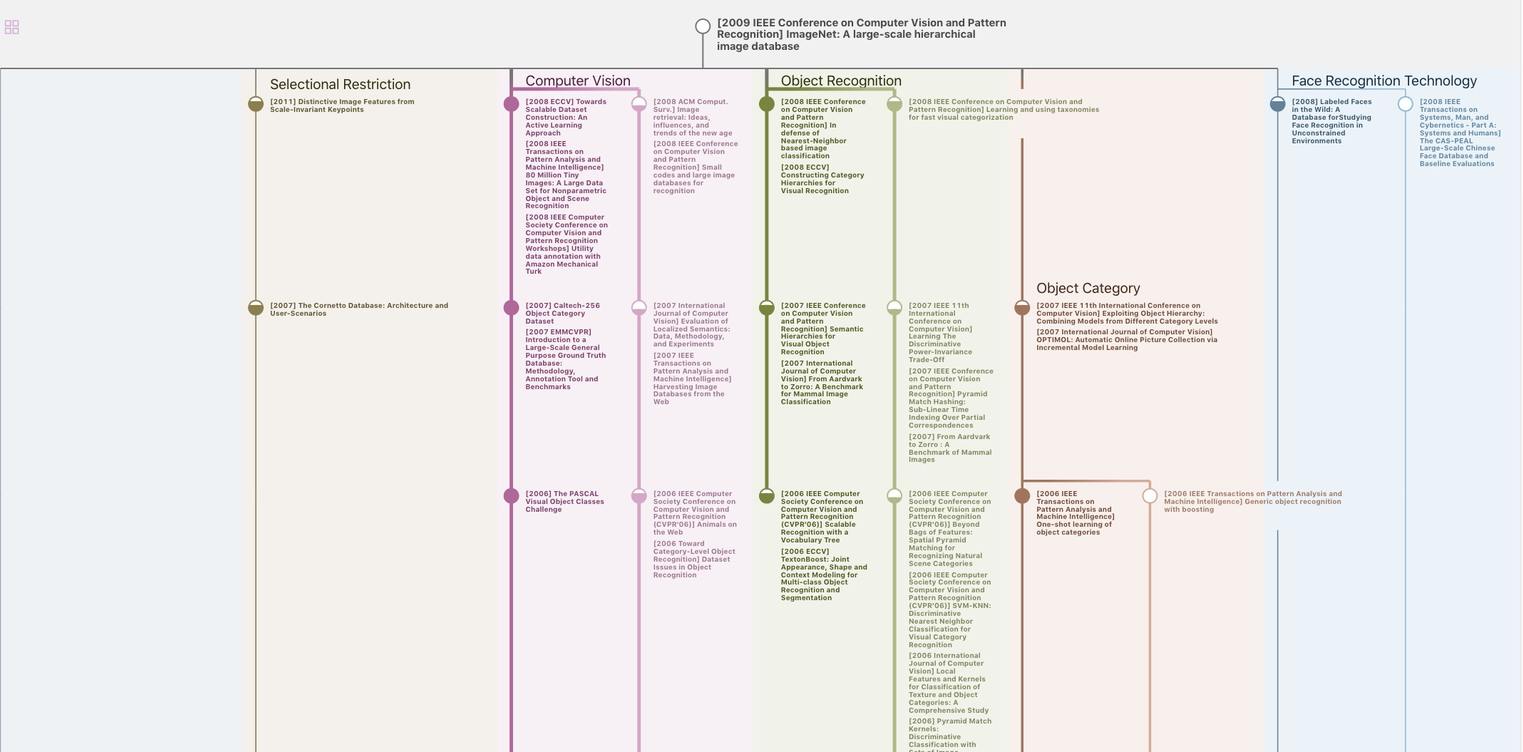
生成溯源树,研究论文发展脉络
Chat Paper
正在生成论文摘要