Fast Bootstrapping Nonparametric Maximum Likelihood for Latent Mixture Models
IEEE SIGNAL PROCESSING LETTERS(2024)
摘要
Estimating the mixing density of a latent mixture model is an important task in signal processing. Nonparametric maximum likelihood estimation is one popular approach to this problem. If the latent variable distribution is assumed to be continuous, then bootstrapping can be used to approximate it. However, traditional bootstrapping requires repeated evaluations on resampled data and is not scalable. In this letter, we construct a generative process to rapidly produce nonparametric maximum likelihood bootstrap estimates. Our method requires only a single evaluation of a novel two-stage optimization algorithm. Simulations and real data analyses demonstrate that our procedure accurately estimates the mixing density with little computational cost even when there are a hundred thousand observations.
更多查看译文
关键词
Bootstrap/resampling,deep neural network,generative process,mixing density estimation,nonparametric maximum likelihood estimation,two-stage algorithm
AI 理解论文
溯源树
样例
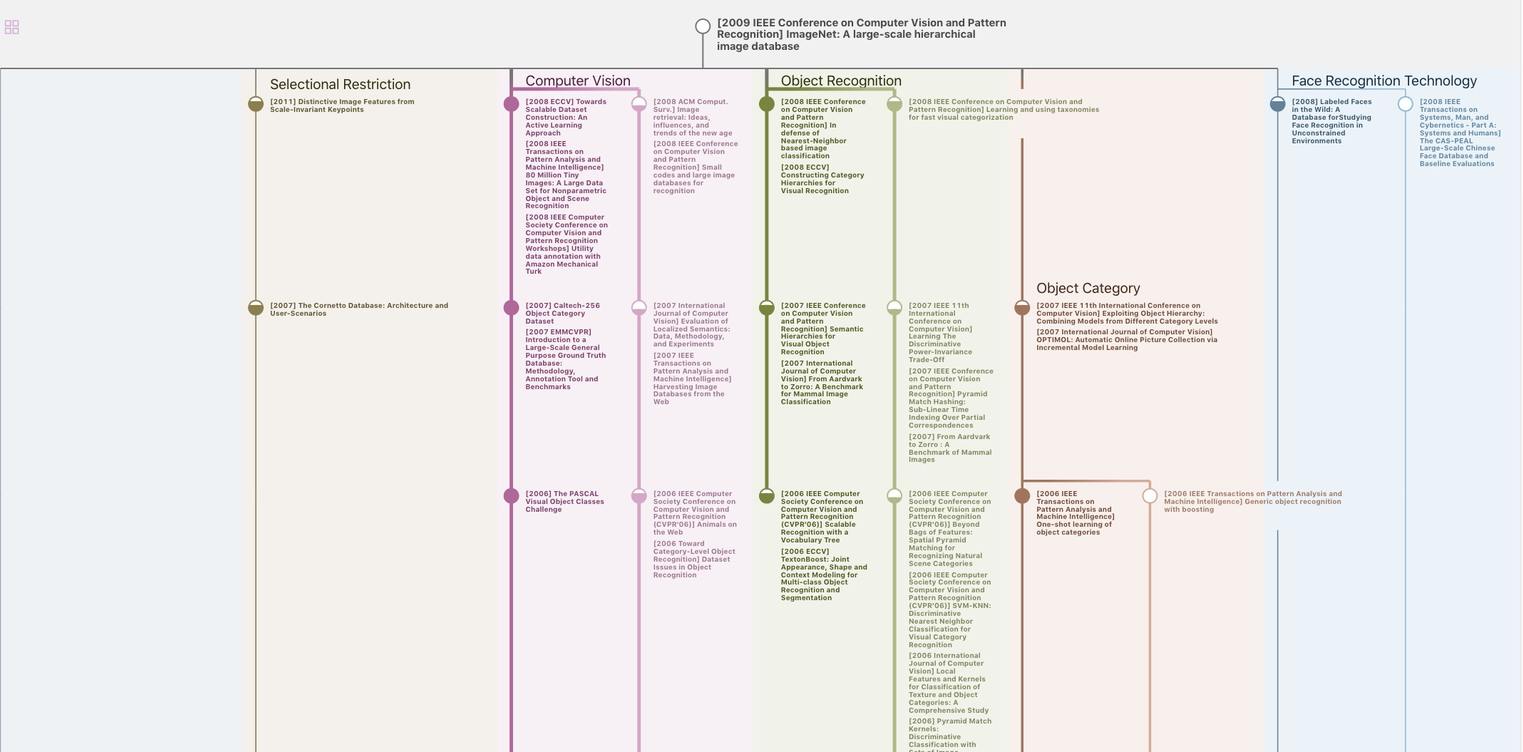
生成溯源树,研究论文发展脉络
Chat Paper
正在生成论文摘要