Graph Convolutional Neural Network for Intelligent Fault Diagnosis of Machines via Knowledge Graph
IEEE TRANSACTIONS ON INDUSTRIAL INFORMATICS(2024)
摘要
Considering the challenge of deep mining of root causes in machine failures, a knowledge aggregation fault diagnosis (KAFD) model is proposed, in which the graph convolutional network (GCN) GraphSAGE is improved and introduced into the knowledge graph (KG)-based fault diagnosis. Historical maintenance data of machines is used to construct a fault phenomenon-FBG, which is then combined with the fault diagnosis knowledge graph (FDKG) to form a collaborative FDKG. A single-layer knowledge aggregation network (KAN) that incorporates sensitivity factors and configures different types of GCN aggregators is constructed in the proposed KAFD. Based on deep neighbor aggregation operations on collaborative FDKG, KAFD obtained by stacking multiple KANs, can capture the higher order structural information and semantic information, which results in the multihop reasoning, improvement of the rationality and diversity of fault cause tracing. The KAFD is experimentally validated through two fault diagnosis datasets, which are constructed by the maintenance data of an industrial enterprise, and the results demonstrate the excellent performance.
更多查看译文
关键词
Fault diagnosis,Maintenance engineering,Knowledge graphs,Task analysis,Knowledge engineering,Sensitivity,Convolutional neural networks,graph neural networks,industrial machines,knowledge graph (KG)
AI 理解论文
溯源树
样例
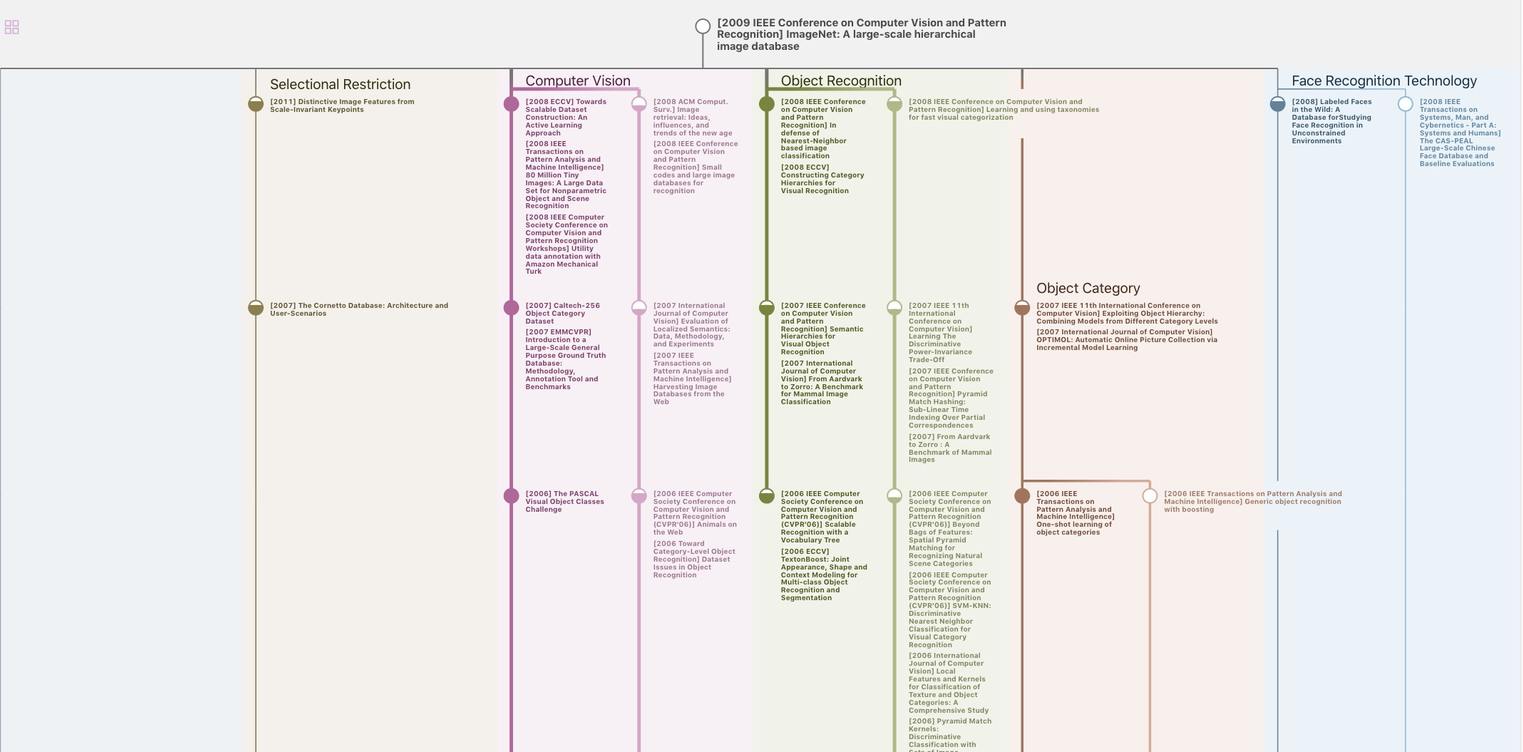
生成溯源树,研究论文发展脉络
Chat Paper
正在生成论文摘要