MINet: Multiscale Interactive Network for Real-Time Salient Object Detection of Strip Steel Surface Defects
IEEE TRANSACTIONS ON INDUSTRIAL INFORMATICS(2024)
摘要
The automated surface defect detection is a fundamental task in industrial production, and the existing saliency-based works overcome the challenging scenes and give promising detection results. However, the cutting-edge efforts often suffer from large parameter size, heavy computational cost, and slow inference speed, which heavily limits the practical applications. To this end, we devise a multiscale interactive (MI) module, which employs depthwise convolution (DWConv) and pointwise convolution (PWConv) to independently extract and interactively fuse features of different scales, respectively. Particularly, the MI module can provide satisfactory characterization for defect regions with fewer parameters. Embarking on this module, we propose a lightweight multiscale interactive network (MINet) to conduct real-time salient object detection of strip steel surface defects. Comprehensive experimental results on SD-Saliency-900 dataset, which contains three kinds of strip steel surface defect detection images (i.e., inclusion, patches, and scratches), demonstrate that the proposed MINet presents comparable detection accuracy with the state-of-the-art methods while running at a GPU speed of 721 FPS and a CPU speed of 6.3 FPS for 368x368 images with only 0.28 M parameters.
更多查看译文
关键词
Computational cost,interaction,multiscale,real-time,surface defect detection
AI 理解论文
溯源树
样例
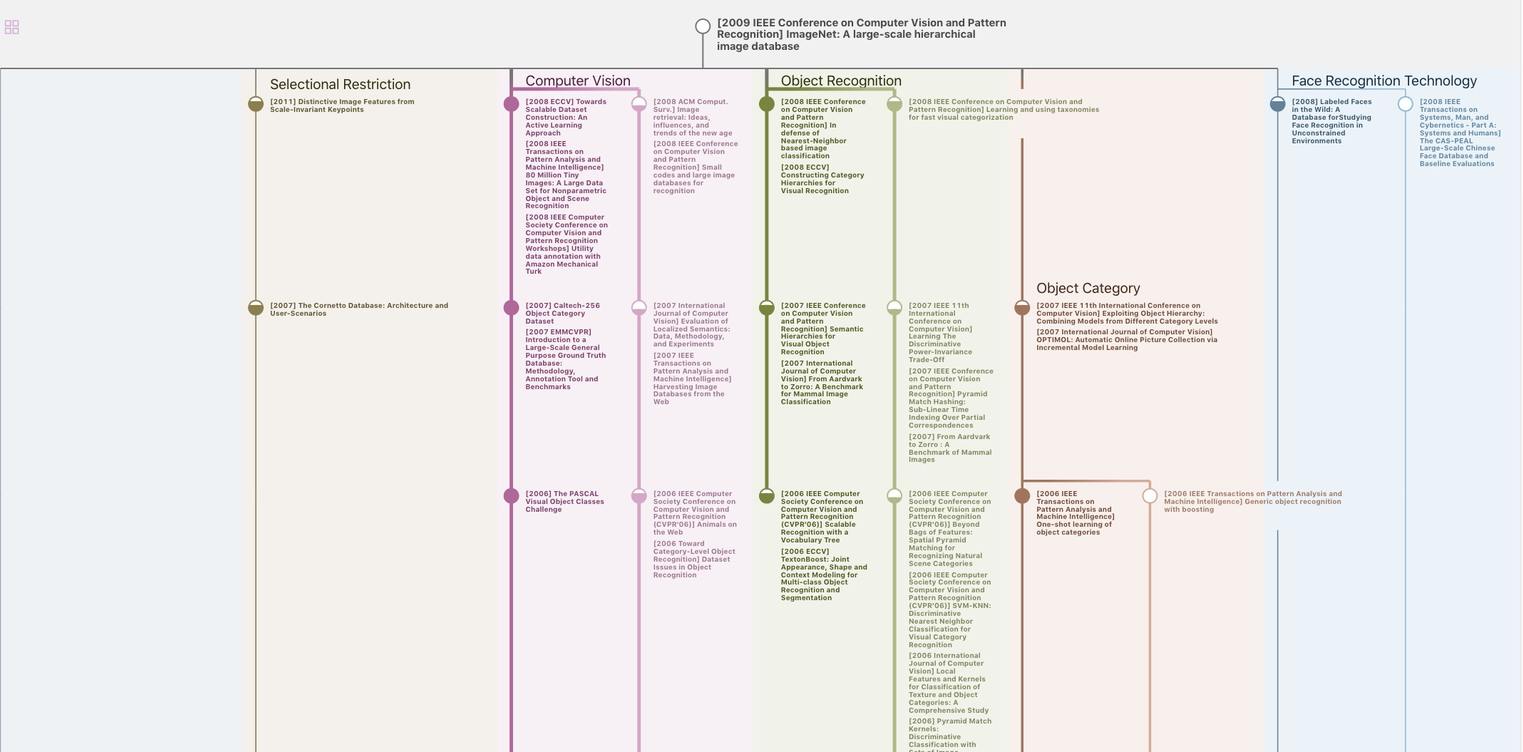
生成溯源树,研究论文发展脉络
Chat Paper
正在生成论文摘要