Load Forecasting-Based Learning System for Energy Management With Battery Degradation Estimation: A Deep Reinforcement Learning Approach
IEEE Transactions on Consumer Electronics(2024)
摘要
The emergence of energy storage system (ESS) enables the service provider to profit from the price difference between purchasing electric energy from utility companies and selling it to customers through battery operations while more frequent charging/discharging behaviors cause battery degradation. However, the accurate estimation of the ESS degradation cost is one of the main obstacles for ESS participating in energy management. This paper considers a smart grid scenario, consisting of an accurate lithium-ion battery degradation (ALBD) model, renewable energy generators and bilateral energy flow from/to the utility grid. We develop an online energy-scheduling approach aiming to maximize the operating profit of the system from energy trading and guarantee the long-term battery usage. We formulate the trade-off between energy trading profit and battery degradation cost as a joint optimization problem, and further transform it into a Markov Decision Process (MDP) problem. To solve the uncertainty of electricity price and demand, we apply a gated recurrent unit to forecast the next day’s price and demand. Then, we develop a deep Q-network to learn an optimized energy-scheduling strategy. The numerical simulations show that our proposed approach can improve the operating profit from 6.04% to 20.54% compared with the three baselines.
更多查看译文
关键词
Energy storage,energy management,battery degradation,deep reinforcement learning,neural networks
AI 理解论文
溯源树
样例
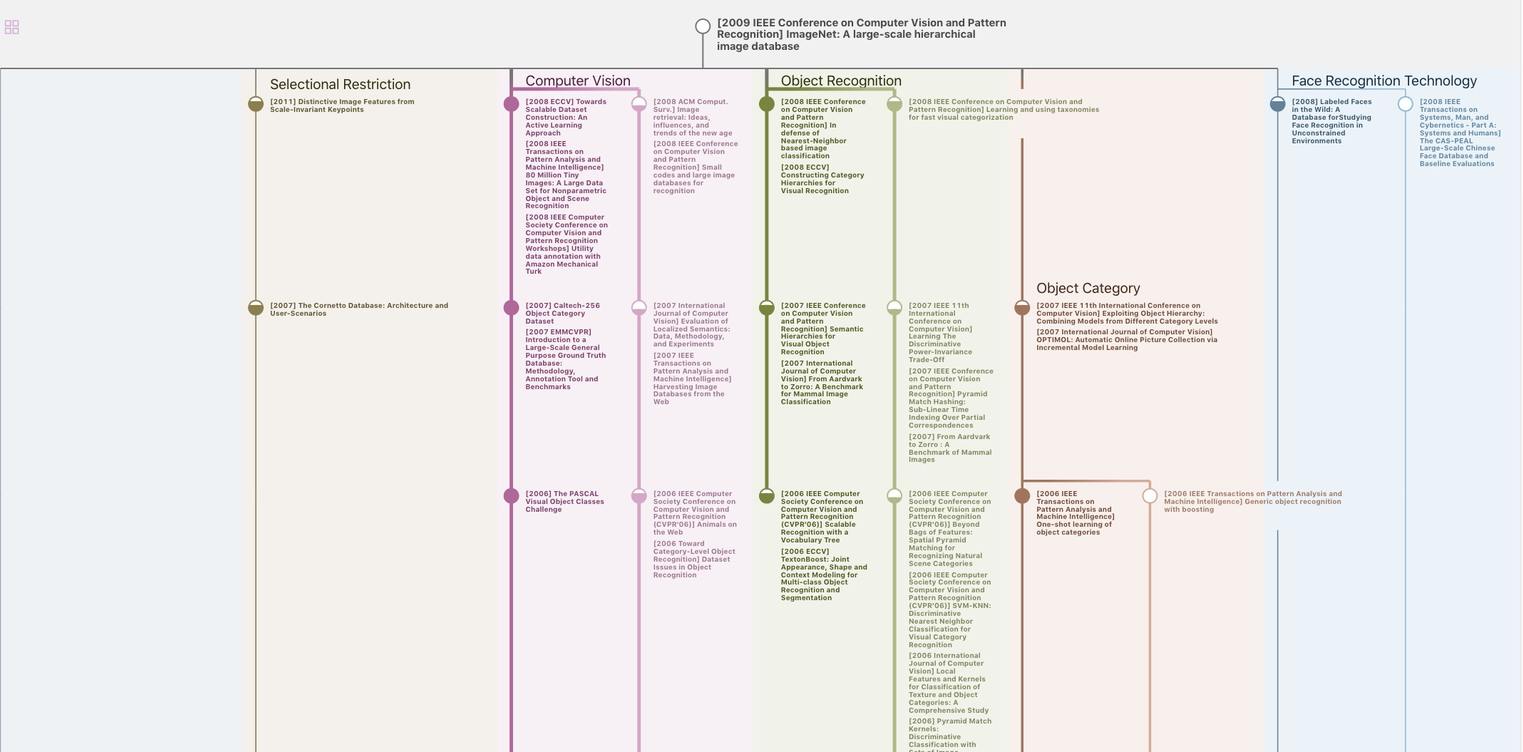
生成溯源树,研究论文发展脉络
Chat Paper
正在生成论文摘要