Ripple Transformer: A Human-Object Interaction Backbone and a New Prediction Strategy for Smart Surveillance Devices
IEEE Transactions on Consumer Electronics(2024)
摘要
Consumers have a growing demand for smart surveillance devices that can perform behavior analysis and provide danger alerts. Human-object interaction (HOI) detection is a crucial component in behavioral analysis. However, extracting interactive semantics is computationally intensive, especially when humans and objects are far apart, making it challenging to integrate HOI methods into smart surveillance devices, which are limited in computational capacity. Moreover, rare interactions cause HOI methods to suffer from a significant long-tail problem. In this paper, we propose a new HOI backbone, namely Ripple Transformer (RITR), and an Object-Centric Prediction (OCP) strategy to recognize HOIs accurately and cost-effectively. Specifically, RITR introduces a new ripple-window attention mechanism that can capture relationship semantics with low computational consumption. The OCP strategy mitigates the impact of the long-tail problem by reformulating the verb classification as a variable-domain multi-classification problem. The proposed methods are verified on ImageNet, COCO, MPII, and HICO-DET datasets. The experimental results showcase the accuracy and cost-efficiency of our methods in image classification, object detection, and HOI detection tasks, demonstrating their practicality for expanding the application scope of smart surveillance devices.
更多查看译文
关键词
Human-object interaction,cost-effectively backbone,ripple-window attention,smart surveillance devices
AI 理解论文
溯源树
样例
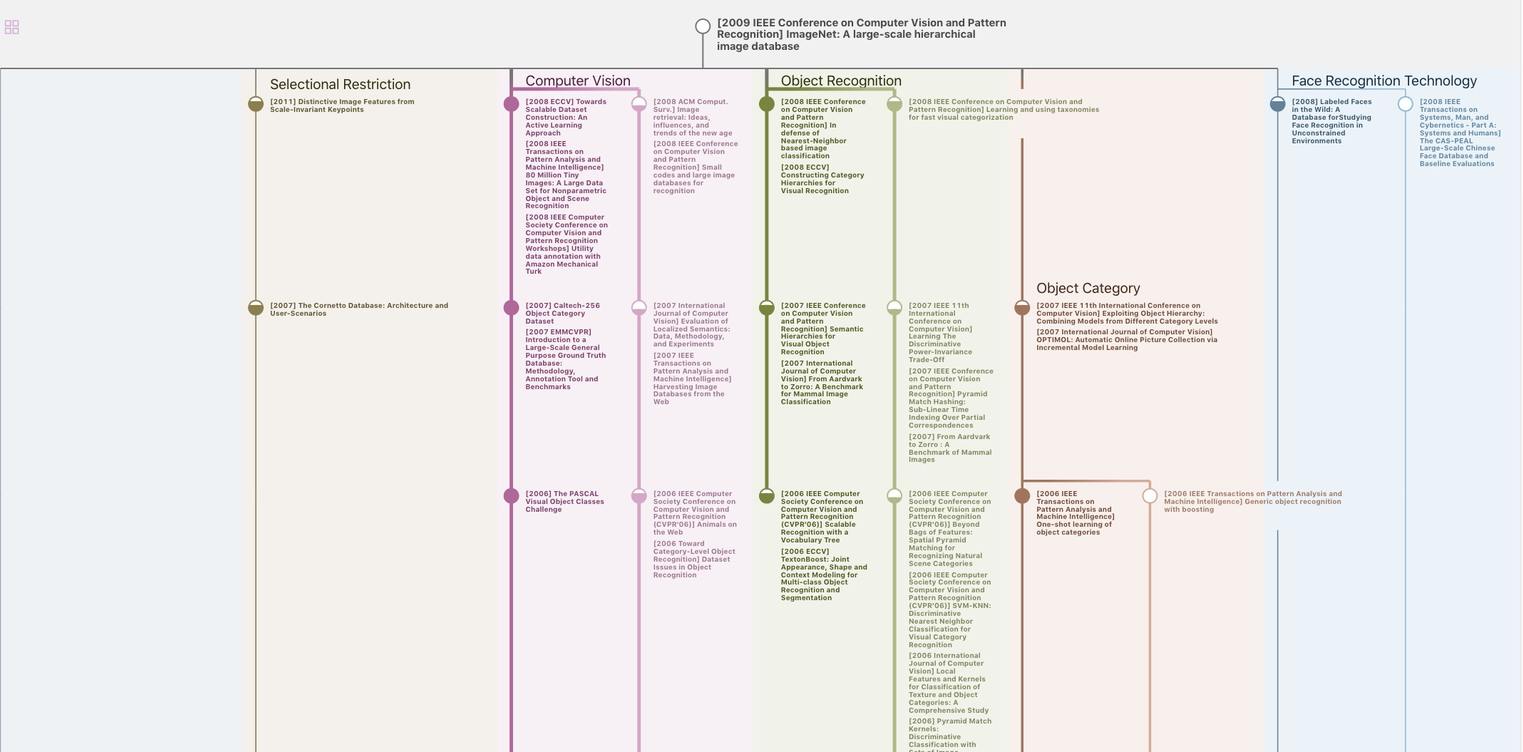
生成溯源树,研究论文发展脉络
Chat Paper
正在生成论文摘要