aiGeneR 1.0: An Artificial Intelligence Technique for the Revelation of Informative and Antibiotic Resistant Genes in Escherichia coli
FRONTIERS IN BIOSCIENCE-LANDMARK(2024)
摘要
Background: There are several antibiotic resistance genes (ARG) for the Escherichia coli (E. coli) bacteria that cause urinary tract infections (UTI), and it is therefore important to identify these ARG. Artificial Intelligence (AI) has been used previously in the field of gene expression data, but never adopted for the detection and classification of bacterial ARG. We hypothesize, if the data is correctly conferred, right features are selected, and Deep Learning (DL) classification models are optimized, then (i) non-linear DL models would perform better than Machine Learning (ML) models, (ii) leads to higher accuracy, (iii) can identify the hub genes, and, (iv) can identify gene pathways accurately. We have therefore designed aiGeneR, the first of its kind system that uses DL -based models to identify ARG in E. coli in gene expression data. Methodology: The aiGeneR consists of a tandem connection of quality control embedded with feature extraction and AI -based classification of ARG. We adopted a cross -validation approach to evaluate the performance of aiGeneR using accuracy, precision, recall, and F1 -score. Further, we analyzed the effect of sample size ensuring generalization of models and compare against the power analysis. The aiGeneR was validated scientifically and biologically for hub genes and pathways. We benchmarked aiGeneR against two linear and two other non-linear AI models. Results: The aiGeneR identifies tetM (an ARG) and showed an accuracy of 93% with area under the curve (AUC) of 0.99 (p < 0.05). The mean accuracy of non-linear models was 22% higher compared to linear models. We scientifically and biologically validated the aiGeneR. Conclusions: aiGeneR successfully detected the E. coli genes validating our four hypotheses.
更多查看译文
关键词
antimicrobial resistance,antibiotic resistance genes,urine tract infection,artificial intelligence,machine learning,eXtreme Gradient Boosting,deep learning
AI 理解论文
溯源树
样例
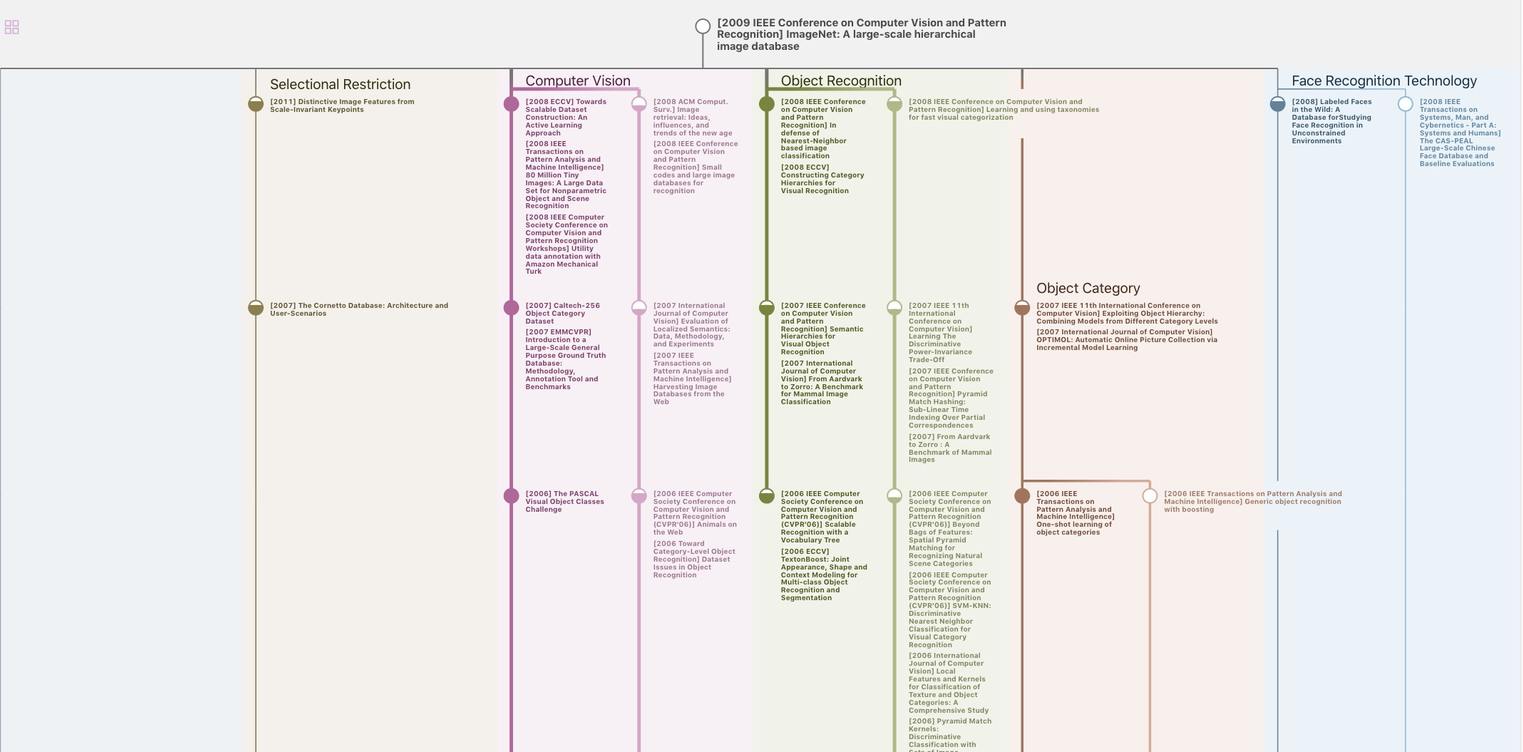
生成溯源树,研究论文发展脉络
Chat Paper
正在生成论文摘要