An optimized EEGNet processor for low-power and real-time EEG classification in wearable brain–computer interfaces
Microelectronics Journal(2024)
摘要
Brain–computer interfaces (BCIs) based on electroencephalogram (EEG) signals have recently gained significant attention. EEGNet is a lightweight convolutional neural network designed for EEG-based BCIs. Previous EEGNet processors are implemented with high-precision fixed-point numbers, resulting in high power consumption and resource utilization. To address these drawbacks, this paper proposes a low-precision EEGNet (LPEEGNet) processor designed with a data-driven processing flow to achieve power-efficient and real-time EEG classification. For algorithm, we reconstruct EEGNet into LPEEGNet through low-precision quantization. Regarding hardware, we first propose a heterogeneous streaming architecture based on layer fusion. Furthermore, we utilize global feature maps memory to improve memory access efficiency. Finally, we achieve both inter-layer parallelism and intra-layer pipelining to enhance inference efficiency. Compared with the state-of-the-art EEGNet processor, experimental results on FPGA show that our processor implementation reduces the power consumption, LUT utilization, and inference latency by 52.6%, 36.2%, and 25.8%, respectively. With an accuracy of 93.06% on the event-related potential dataset, our processor is more suitable for wearable BCIs.
更多查看译文
关键词
EEGNet,Brain–computer interface (BCI),Convolutional neural networks (CNNs),FPGA,Electroencephalography
AI 理解论文
溯源树
样例
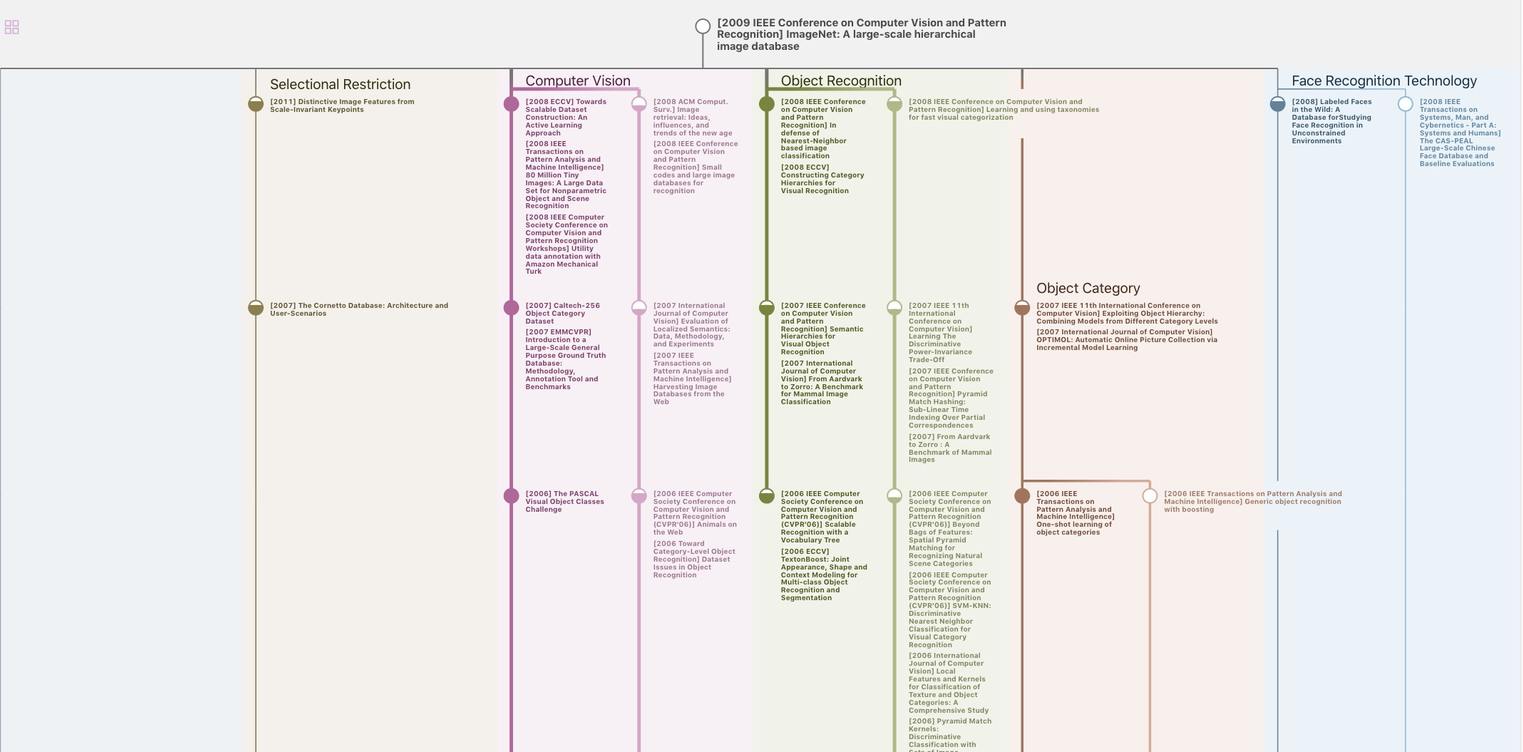
生成溯源树,研究论文发展脉络
Chat Paper
正在生成论文摘要