GrassCaré: Visualizing the Grassmannian on the Poincaré Disk
SN Computer Science(2024)
摘要
This paper introduces a novel method for visualizing high-dimensional Grassmannians through 2D embeddings on the Poincaré disk. The proposed approach involves the construction of affinity matrices on each manifold, followed by the minimization of KL-divergence between the geodesics affinity. This process enables the identification of an optimal projection that effectively preserves the geometry of the original high-dimensional Grassmannian. Our main theoretical contribution lies in bounding the embedding loss with respect to two factors: the logarithm of the number of subspaces and a term dependent on the distribution of subspaces within the Grassmannian. Notably, this term is smaller when subspaces exhibit well-defined clusters, and larger in the absence of any discernible structure. We complement our theoretical analysis with comprehensive experiments on both synthetic and real datasets. The experimental results showcase the superiority of our embedding in accurately visualizing Grassmannians compared to existing representations.
更多查看译文
关键词
Grassmannian,Manifold learning,Poincaré disk,t-SNE,High-dimensional data and dimensionality reduction
AI 理解论文
溯源树
样例
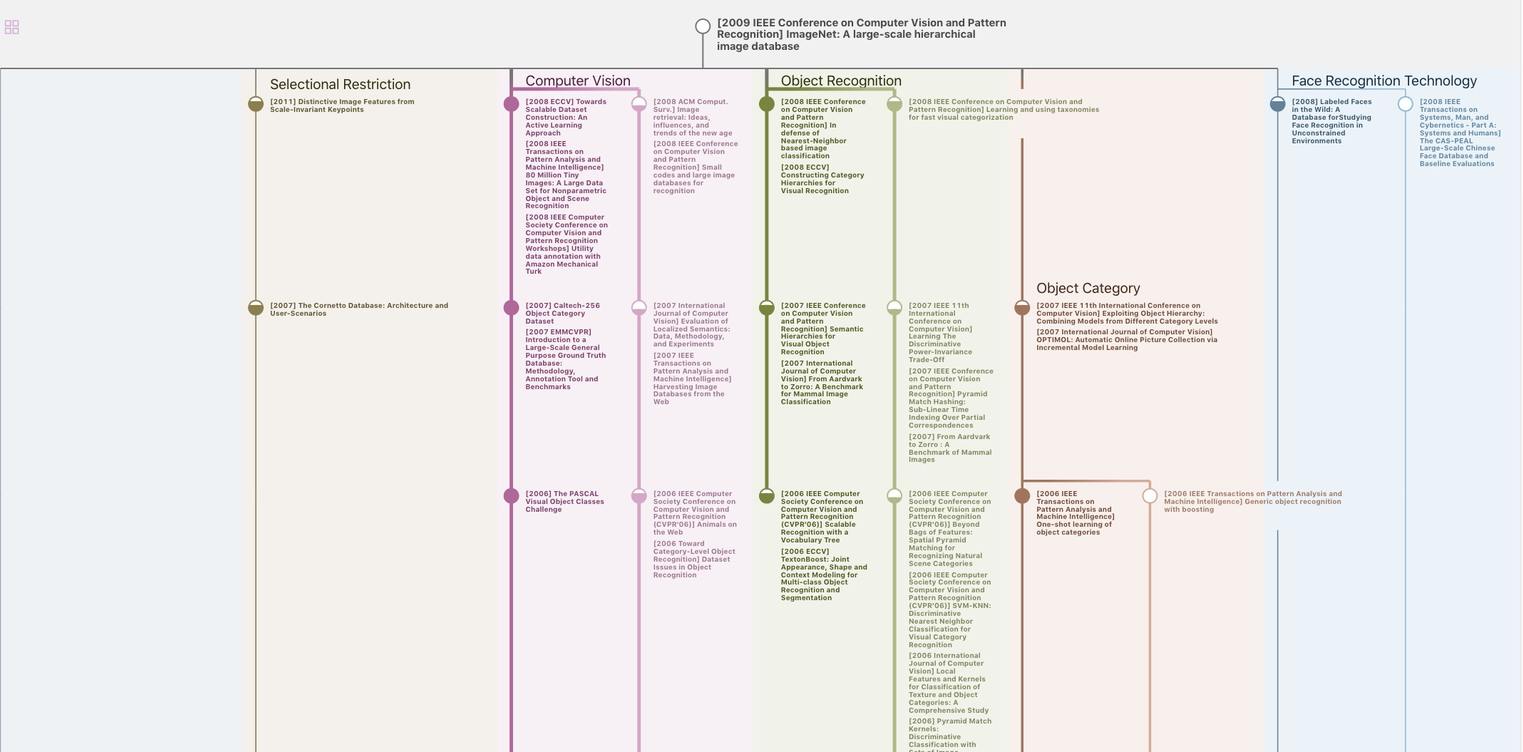
生成溯源树,研究论文发展脉络
Chat Paper
正在生成论文摘要