Evaluation of sparsity metrics and evolutionary algorithms applied for normalization of H&E histological images
Pattern Analysis and Applications(2024)
摘要
Color variations in H E histological images can impact the segmentation and classification stages of computational systems used for cancer diagnosis. To address these variations, normalization techniques can be applied to adjust the colors of histological images. Estimates of stain color appearance matrices and stain density maps can be employed to carry out these color adjustments. This study explores these estimates by leveraging a significant biological characteristic of stain mixtures, which is represented by a sparsity parameter. Computationally estimating this parameter can be accomplished through various sparsity measures and evolutionary algorithms. Therefore, this study aimed to evaluate the effectiveness of different sparsity measures and algorithms for color normalization of H E-stained histological images. The results obtained demonstrated that the choice of different sparsity measures significantly impacts the outcomes of normalization. The sparsity metric l_ϵ^0 proved to be the most suitable for it. Conversely, the evolutionary algorithms showed little variations in the conducted quantitative analyses. Regarding the selection of the best evolutionary algorithm, the results indicated that particle swarm optimization with a population size of 250 individuals is the most appropriate choice.
更多查看译文
关键词
Histopathology image analysis,H E color normalization,Sparsity estimation,Evolutionary algorithms,Sparse nonnegative matrix factorization
AI 理解论文
溯源树
样例
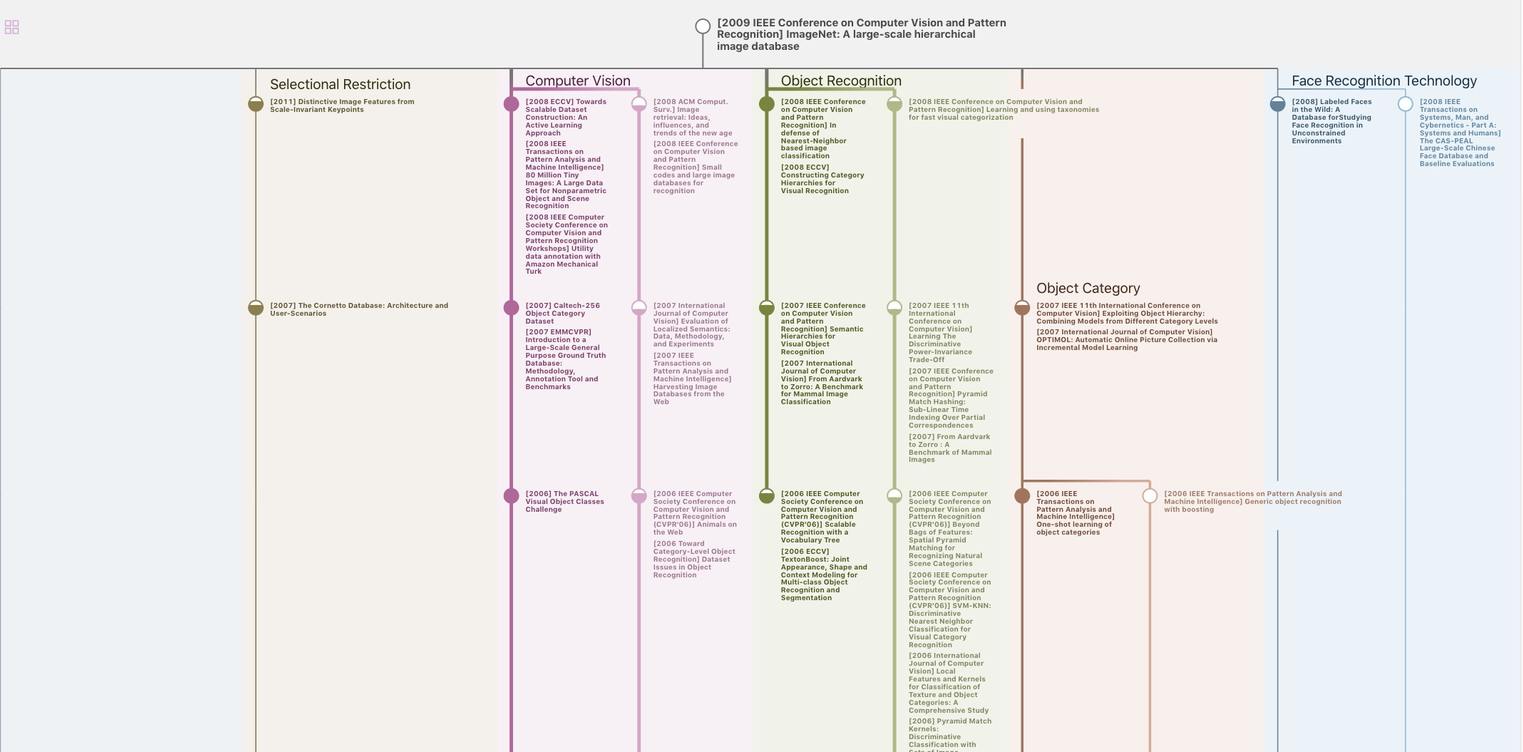
生成溯源树,研究论文发展脉络
Chat Paper
正在生成论文摘要