Skip-attention Optimization Methods for Machine Learning Models for Papillary Thyroid Carcinoma Detection Based on Ultrasound Images
2023 IEEE International Conference on Electrical, Automation and Computer Engineering (ICEACE)(2023)
摘要
Ultrasound images are commonly utilized for detecting papillary thyroid carcinoma (PTC). Nevertheless, this approach is time-consuming and resource intensive. Machine learning (ML) algorithms have shown proficiency in extracting meaningful patterns and features from medical images, enabling them to identify subtle differences between benign and malignant thyroid nodules, which might challenge human observers. While VGG16 is a common ML architecture for PTC detection, it exhibits a limitation of limited contextual information. In response, this study proposes an optimization method based on the Skip-attention model optimization mechanism to address this limitation. The results demonstrate that the model's performance in terms of accuracy (96.62% vs. 78.00%), AUC (96.62% vs. 82.10%), sensitivity (94.58% vs. 88.11%), and Fl-score (84.00% vs. 71.00%) is significantly improved by this mechanism. The proposed method makes several significant contributions to ML model optimizations, including improved accuracy, enhanced interpretability, reduced overfitting, transferable insights, potential clinical impact, benchmarking for future research, generalization to other architectures, and robustness to image variability. These findings provide crucial reference value for future related research endeavors.
更多查看译文
关键词
Machine learning,Papillary thyroid carcinoma,Artificial Intelligence,Skip-attention optimization methods
AI 理解论文
溯源树
样例
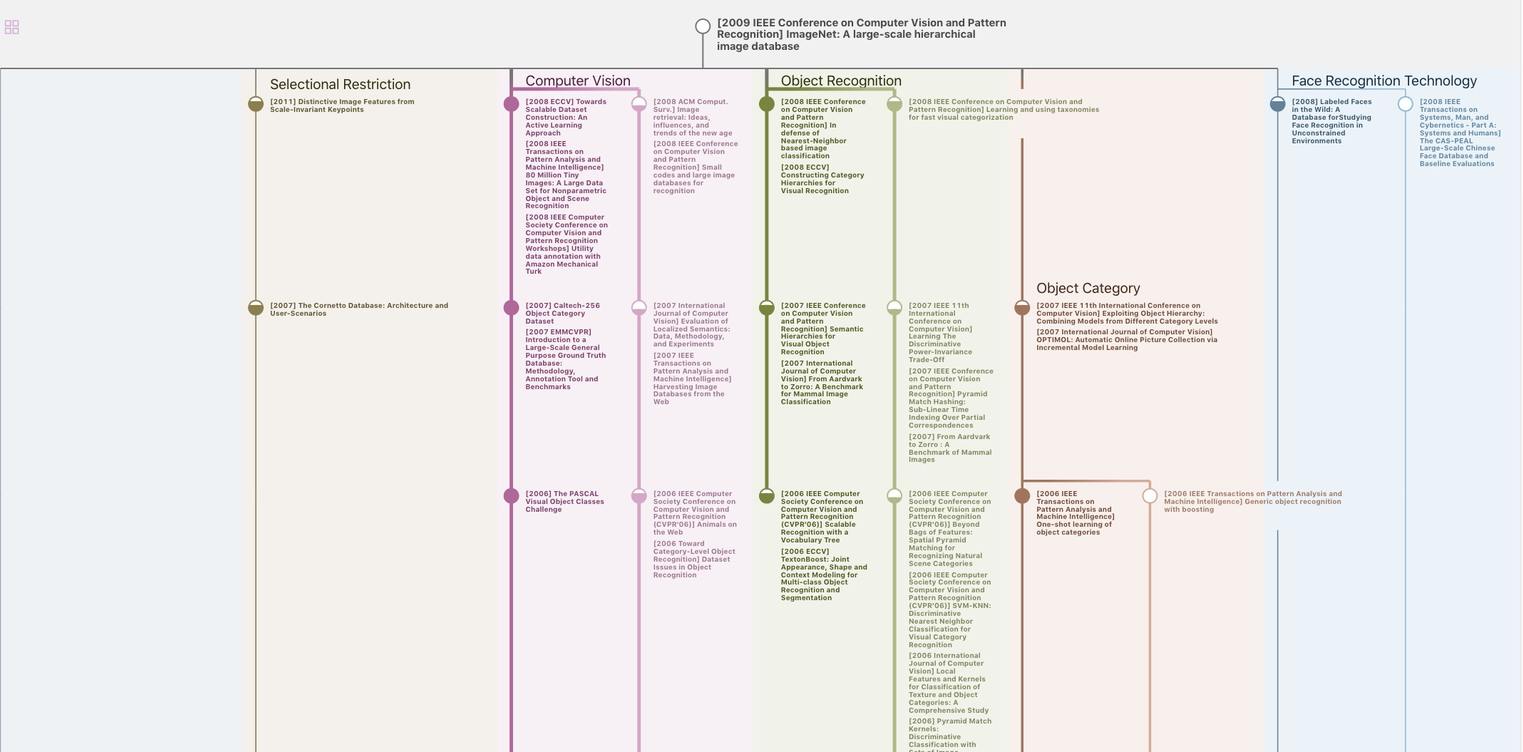
生成溯源树,研究论文发展脉络
Chat Paper
正在生成论文摘要