Deep Anomaly Detection of Voltage Waveform Distortion in the Transmission Grid Due to Geomagnetically Induced Currents
IEEE TRANSACTIONS ON INSTRUMENTATION AND MEASUREMENT(2024)
摘要
This work proposes applying a deep-learning (DL) method to detect anomalies in waveform distortion measurements and relate them to geomagnetically induced currents (GICs). GIC causes saturation of the transformers that inject high harmonic content into the grid. Such harmonics can propagate throughout the power grid, which can cause unwanted events, such as the tripping of protective relays. The worst scenario is when the GIC can cause a complete loss of the major lines in the transmission grid. The DL method allows the detection of the days with voltage waveform anomalies and further relates them with geomagnetic disturbances. The deep anomaly detection method is based on a deep autoencoder for dimensional reduction followed by clustering. The method is applied to harmonic measurements from four distinct locations in the Swedish transmission grid (400 kV) collected over four years with 10-min sampling. The results showed that harmonics present signatures not only in the even harmonic spectra, but also in the odd harmonic spectra. Furthermore, the postprocessing of the automatic results given by the deep anomaly detection shows that the solar cycle, the ground conductance, the variation of the Earth's magnetic field, and the load variation can play a role in the harmonic magnitude.
更多查看译文
关键词
Deep learning (DL),geomagnetically induced currents (GICs),power quality (PQ),transmission grid,waveform distortion
AI 理解论文
溯源树
样例
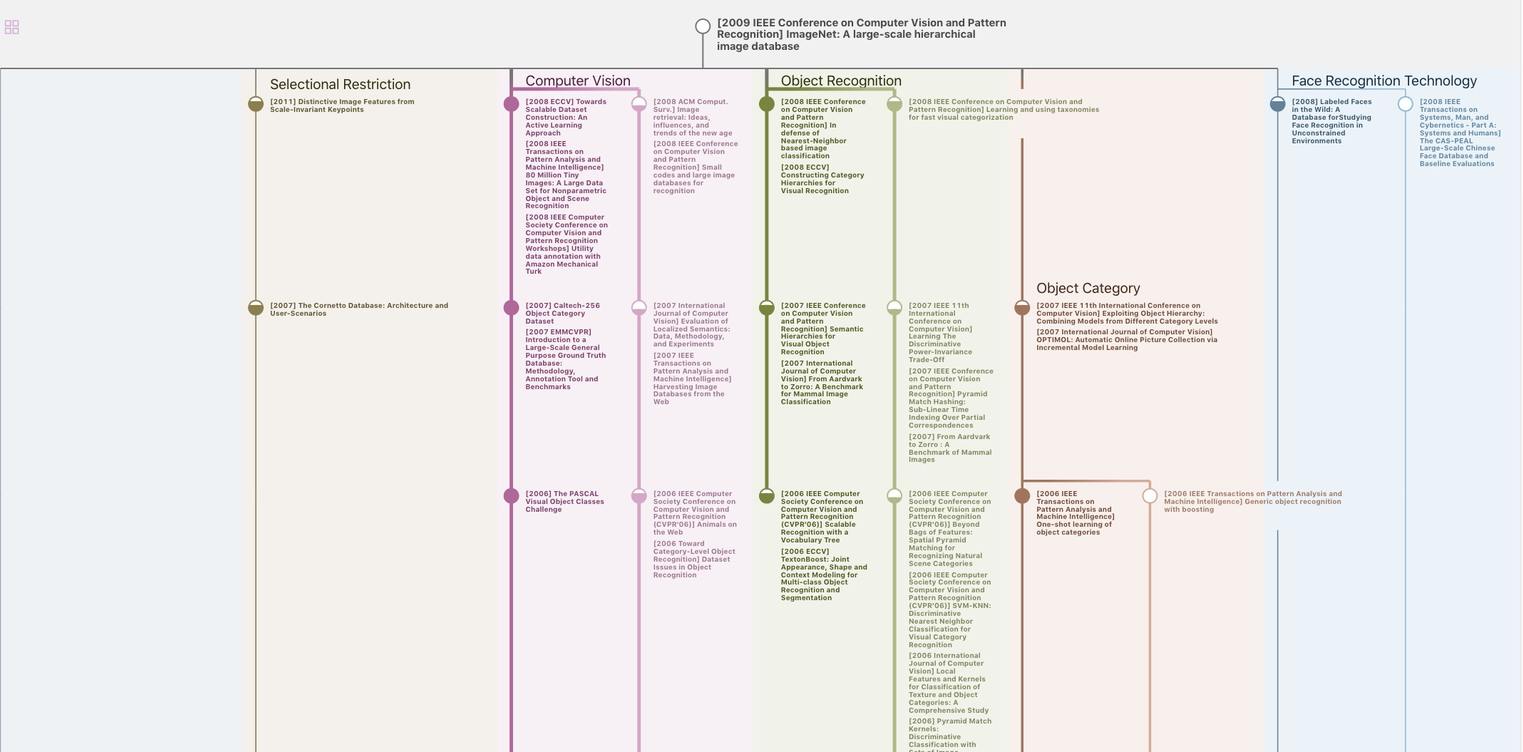
生成溯源树,研究论文发展脉络
Chat Paper
正在生成论文摘要