Action Knowledge Graph for Violence Detection Using Audiovisual Features.
IEEE International Conference on Consumer Electronics(2024)
摘要
Detecting violent content in video frames is a crucial aspect of violence detection. Combining visual and audio cues is often the most effective way to identify violent behavior, as they complement each other. However, studies that examine the fusion of these cues in violence detection are computationally expensive and limited. To address this problem, we investigated various methods for integrating visual and audio information and proposed a Fused Vision-based Action Knowledge Graph (FV-AKG) for violence detection using audiovisual information. The authors have designed a network with three parallel branches named integrated, specialized, and scoring that capture and integrate the distinct relationships between audio and video samples. Our proposed FV-AKG captures the long-range dependencies based on similarity priors in the integrated branch, while proximity priors are used for local positional relationships in the specialized branch. In addition, the scoring branch indicates how close the predictions are to reality. We used two key operations during model training: aggregation and update, each with its learnable weights. In the aggregation operation, long-range dependencies are compiled from global vertices, whereas in the update function, nonlinear transforms are used to compute new representations. We thoroughly investigated the possibilities of temporal context modeling using graphs and found that FV-AKG is the best option for real-time violence detection. Our experiments showed that FV-AKG outperforms the current top State-of-The-Art (SoTA) methods on the XD-Violence datasets.
更多查看译文
关键词
Audio-visual Features,Violence Detection,Visual Information,Visual Cues,Learned Weights,Audio Data,Long-range Dependencies,Video Samples,Aggregation Operators,Audio Cues,Audio Information,Audiovisual Information,Visual Features,Unimodal,Relationship Matrix,Optical Flow,Graph Convolutional Network,Graph Convolution,Extract Visual Features,RGB Features
AI 理解论文
溯源树
样例
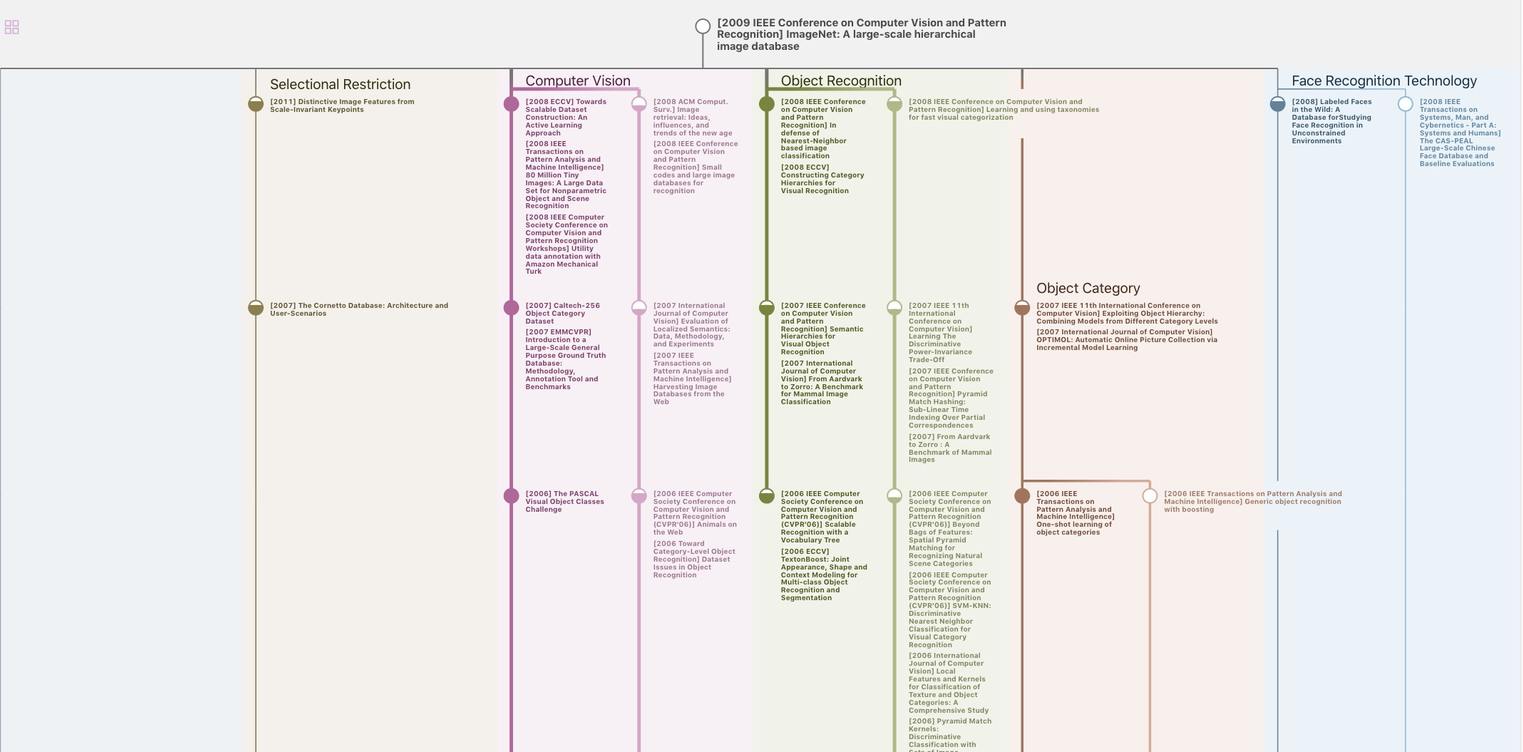
生成溯源树,研究论文发展脉络
Chat Paper
正在生成论文摘要